Computationally Constrained Audio-Based Violence Detection Through Transfer Learning and Data Augmentation Techniques
APPLIED ACOUSTICS(2023)
关键词
Violence detection,Audio processing,Computational cost
AI 理解论文
溯源树
样例
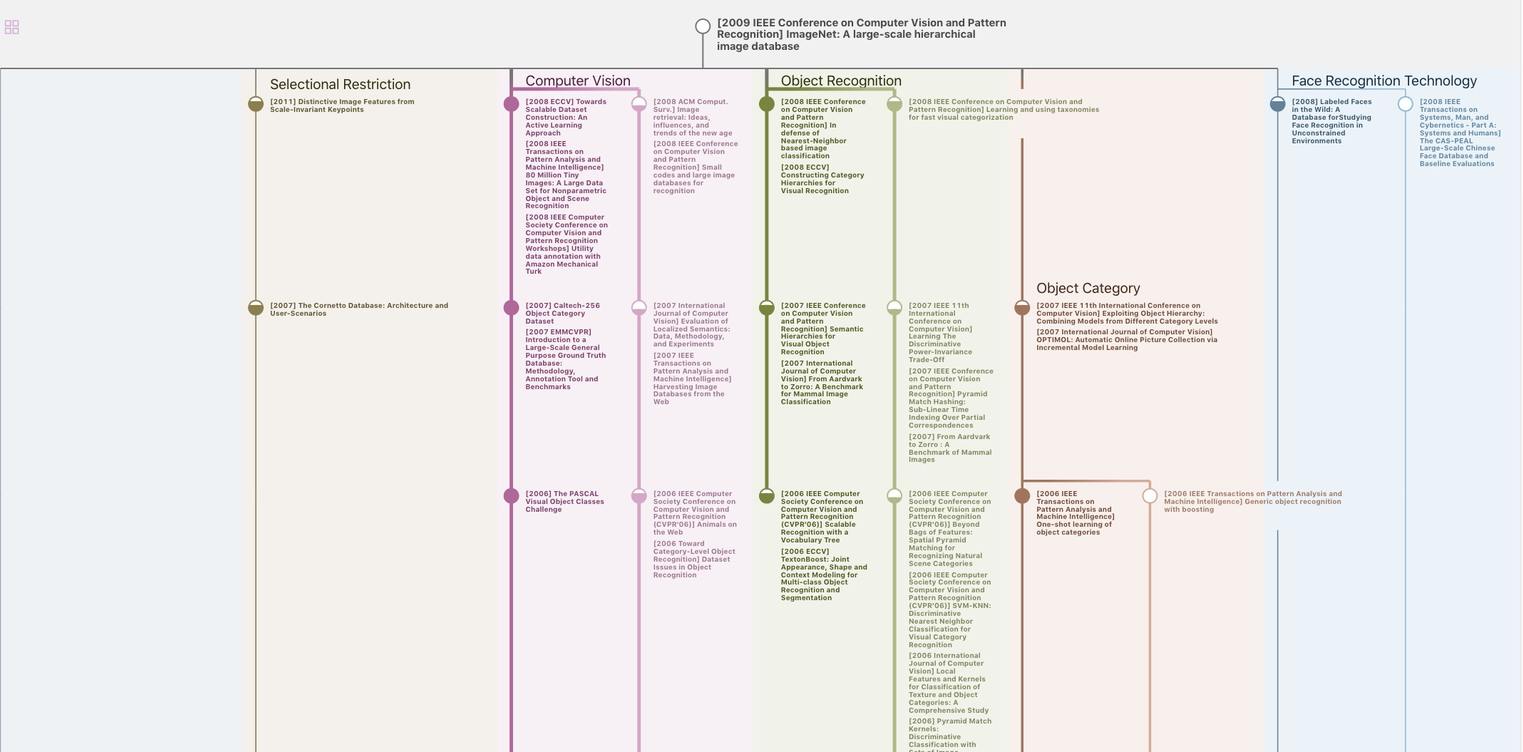
生成溯源树,研究论文发展脉络
Chat Paper
正在生成论文摘要