Computing Speed-of-Sound From Ultrasound: User-Agnostic Recovery and a New Benchmark
IEEE TRANSACTIONS ON BIOMEDICAL ENGINEERING(2024)
摘要
Objective: Medical ultrasound is one of the most accessible imaging modalities, but is a challenging modality for quantitative parameters comparison across vendors and sonographers. B-Mode imaging, with limited exceptions, provides a map of tissue boundaries; crucially, it does not provide diagnostically relevant physical quantities of the interior of organ domains.This can be remedied: the raw ultrasound signal carries significantly more information than is present in the B-Mode image. Specifically, the ability to recover speed-of-sound and attenuation maps from the raw ultrasound signal transforms the modality into a tissue-property modality. Deep learning was shown to be a viable tool for recovering speed-of-sound maps. A major hold-back towards deployment is the domain transfer problem, i.e., generalizing from simulations to real data. This is due in part to dependence on the (hard-to-calibrate) system response. Methods: We explore a remedy to the problem of operator-dependent effects on the system response by introducing a novel approach utilizing the phase information of the IQ demodulated signal. Results: We show that the IQ-phase information effectively decouples the operator-dependent system response from the data, significantly improving the stability of speed-of-sound recovery. We also introduce an improvement to the network topology providing faster and improved results to the state-of-the-art. We present the first publicly available benchmark for this problem: a simulated dataset for raw ultrasound plane wave processing. Conclusion: The consideration of the phase of the IQ-signals presents a promising appeal to traversing the transfer learning problem, advancing the goal of real-time speed-of-sound imaging.
更多查看译文
关键词
Ultrasonic imaging,Imaging,Attenuation,Shape,Reflection,Acoustic beams,Kidney,Deep learning,inverse problems,speed-of-sound inversion,ultrasound
AI 理解论文
溯源树
样例
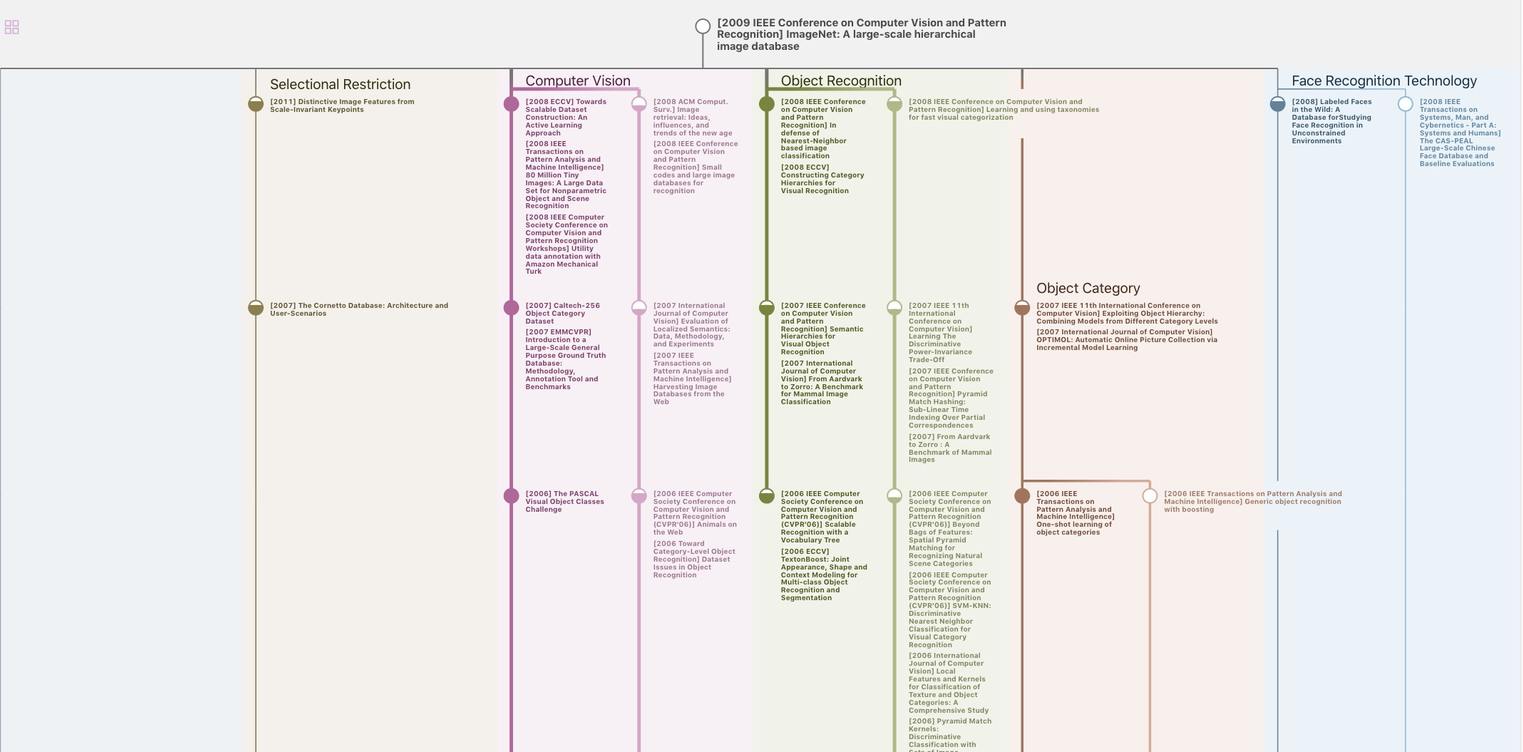
生成溯源树,研究论文发展脉络
Chat Paper
正在生成论文摘要