Probing Representations for Document-level Event Extraction.
CoRR(2023)
摘要
The probing classifiers framework has been employed for interpreting deep neural network models for a variety of natural language processing (NLP) applications. Studies, however, have largely focused on sentencelevel NLP tasks. This work is the first to apply the probing paradigm to representations learned for document-level information extraction (IE). We designed eight embedding probes to analyze surface, semantic, and event-understanding capabilities relevant to document-level event extraction. We apply them to the representations acquired by learning models from three different LLM-based document-level IE approaches on a standard dataset. We found that trained encoders from these models yield embeddings that can modestly improve argument detections and labeling but only slightly enhance event-level tasks, albeit trade-offs in information helpful for coherence and event-type prediction. We further found that encoder models struggle with document length and cross-sentence discourse.
更多查看译文
关键词
event,extraction,document-level
AI 理解论文
溯源树
样例
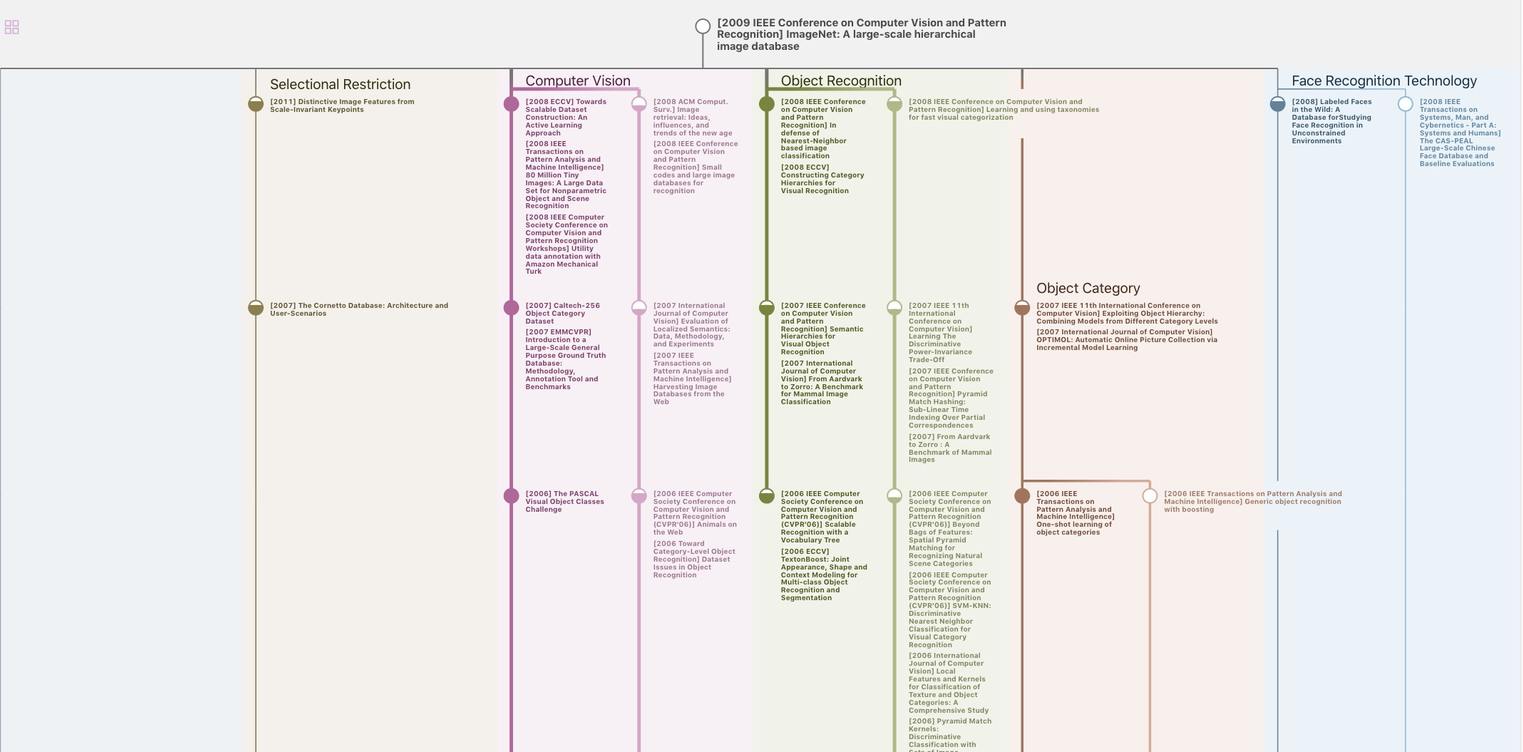
生成溯源树,研究论文发展脉络
Chat Paper
正在生成论文摘要