Towards Understanding Sycophancy in Language Models
arXiv (Cornell University)(2023)
摘要
Reinforcement learning from human feedback (RLHF) is a popular technique for training high-quality AI assistants. However, RLHF may also encourage model responses that match user beliefs over truthful responses, a behavior known as sycophancy. We investigate the prevalence of sycophancy in RLHF-trained models and whether human preference judgements are responsible. We first demonstrate that five state-of-the-art AI assistants consistently exhibit sycophantic behavior across four varied free-form text-generation tasks. To understand if human preferences drive this broadly observed behavior of RLHF models, we analyze existing human preference data. We find that when a response matches a user's views, it is more likely to be preferred. Moreover, both humans and preference models (PMs) prefer convincingly-written sycophantic responses over correct ones a negligible fraction of the time. Optimizing model outputs against PMs also sometimes sacrifices truthfulness in favor of sycophancy. Overall, our results indicate that sycophancy is a general behavior of RLHF models, likely driven in part by human preference judgements favoring sycophantic responses.
更多查看译文
关键词
AI safety,language models,sycophancy,human feedback,RLHF
AI 理解论文
溯源树
样例
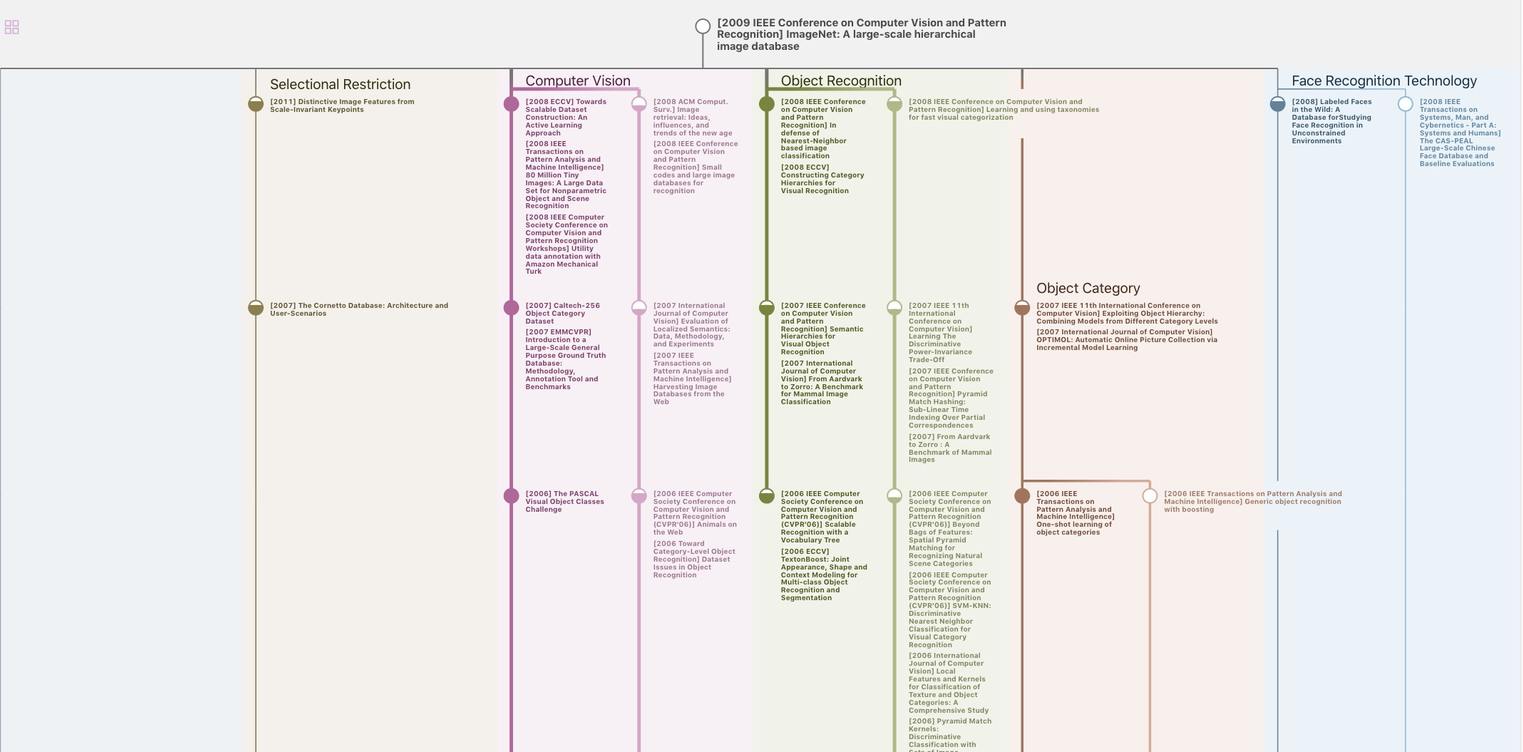
生成溯源树,研究论文发展脉络
Chat Paper
正在生成论文摘要