Addressing Label Noise for Electronic Health Records: Insights from Computer Vision for Tabular Data
BMC Medical Informatics and Decision Making(2024)
Key words
Machine learning,Electronic health records,Noisy labels
AI Read Science
Must-Reading Tree
Example
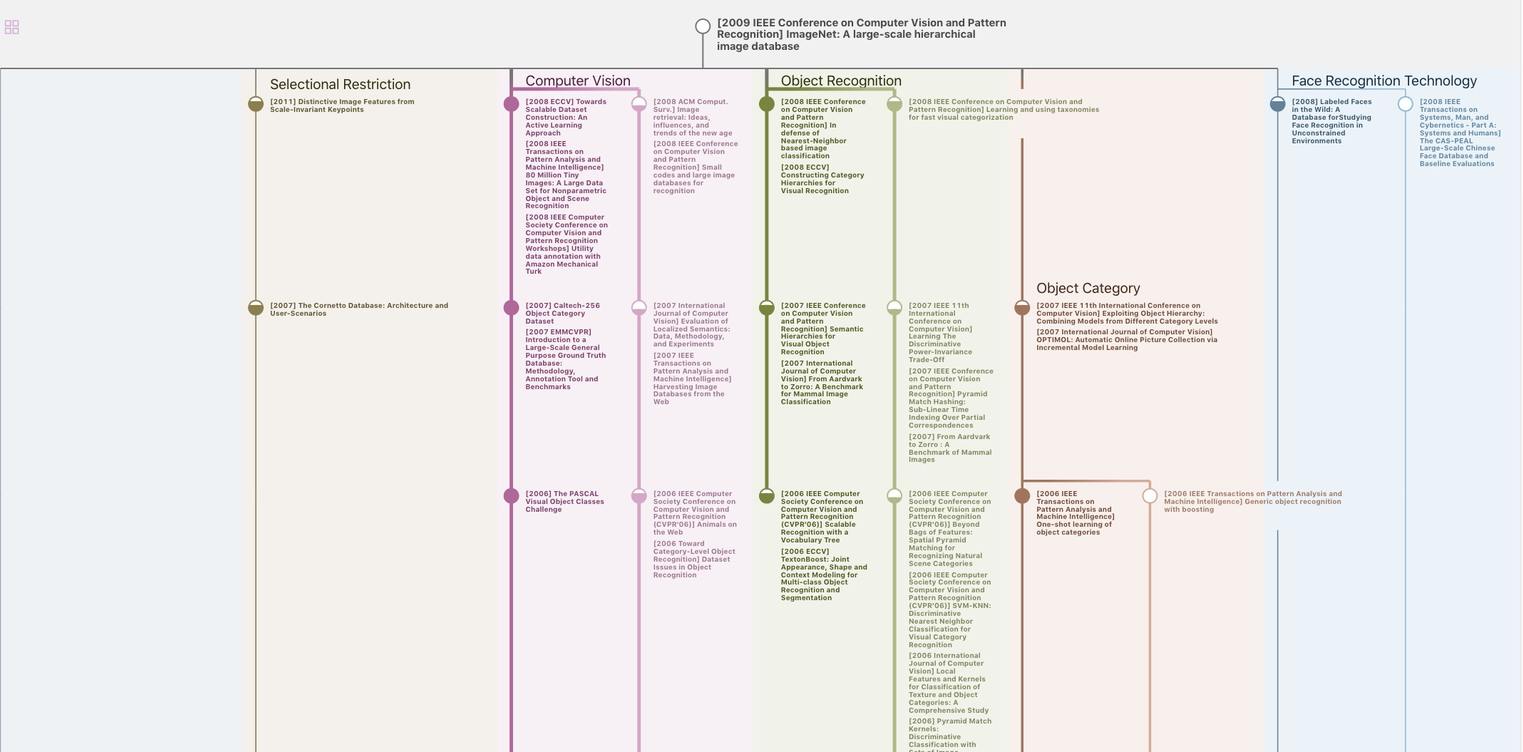
Generate MRT to find the research sequence of this paper
Chat Paper
Summary is being generated by the instructions you defined