Regional-to-Local Point-Voxel Transformer for Large-Scale Indoor 3D Point Cloud Semantic Segmentation
Remote Sensing(2023)
摘要
Semantic segmentation of large-scale indoor 3D point cloud scenes is crucial for scene understanding but faces challenges in effectively modeling long-range dependencies and multi-scale features. In this paper, we present RegionPVT, a novel Regional-to-Local Point-Voxel Transformer that synergistically integrates voxel-based regional self-attention and window-based point-voxel self-attention for concurrent coarse-grained and fine-grained feature learning. The voxel-based regional branch focuses on capturing regional context and facilitating inter-window communication. The window-based point-voxel branch concentrates on local feature learning while integrating voxel-level information within each window. This unique design enables the model to jointly extract local details and regional structures efficiently and provides an effective and efficient solution for multi-scale feature fusion and a comprehensive understanding of 3D point clouds. Extensive experiments on S3DIS and ScanNet v2 datasets demonstrate that our RegionPVT achieves competitive or superior performance compared with state-of-the-art approaches, attaining mIoUs of 71.0% and 73.9% respectively, with significantly lower memory footprint.
更多查看译文
关键词
point cloud, semantic segmentation, regional-to-local, self-attention, multi-scale feature, deep learning
AI 理解论文
溯源树
样例
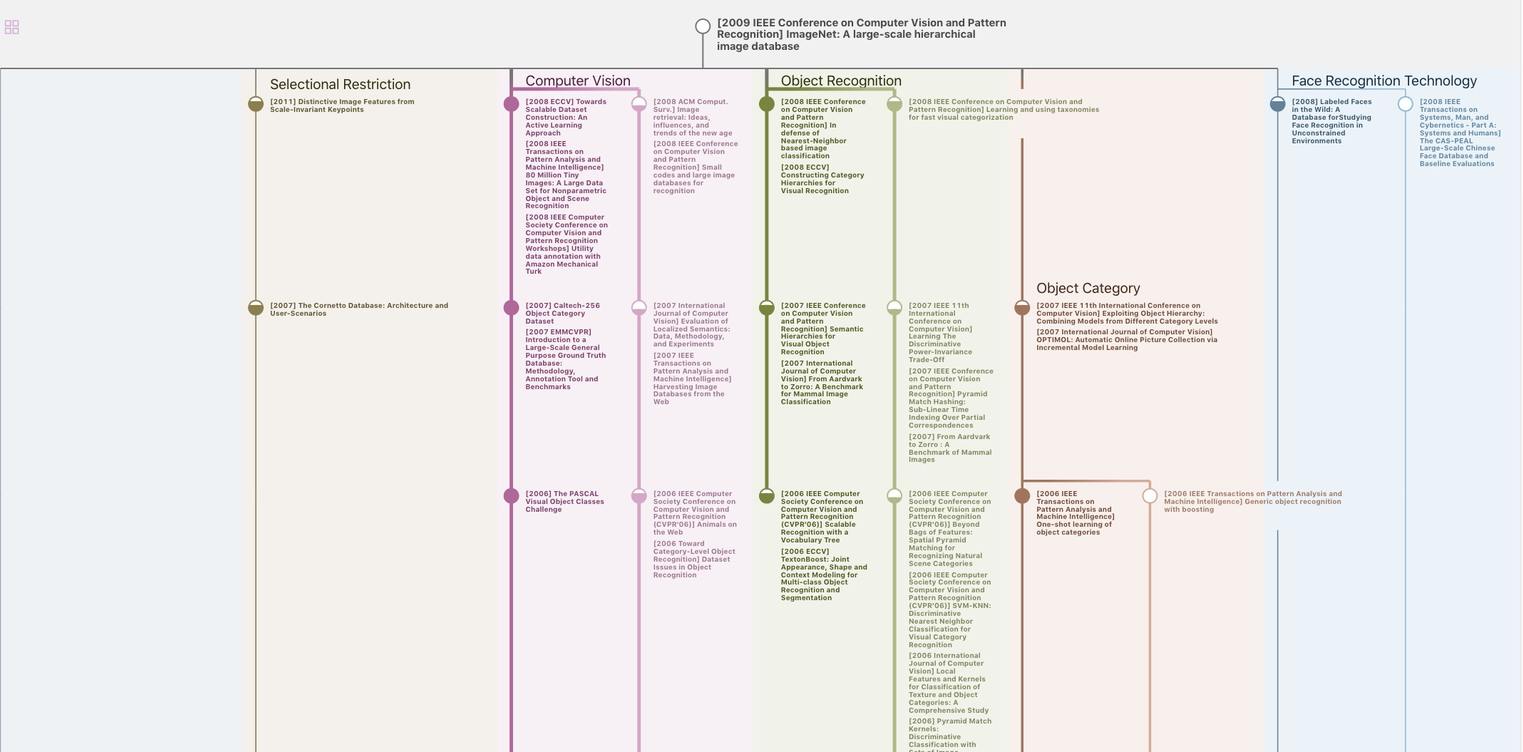
生成溯源树,研究论文发展脉络
Chat Paper
正在生成论文摘要