Deep Learning-Based Radiomic Nomogram to Predict Risk Categorization of Thymic Epithelial Tumors: A Multicenter Study.
EUROPEAN JOURNAL OF RADIOLOGY(2023)
Key words
Radiomics,Deep learning,Thymic epithelial tumors,Pathologic risk categorization,Computed tomography,Radiomic nomogram
AI Read Science
Must-Reading Tree
Example
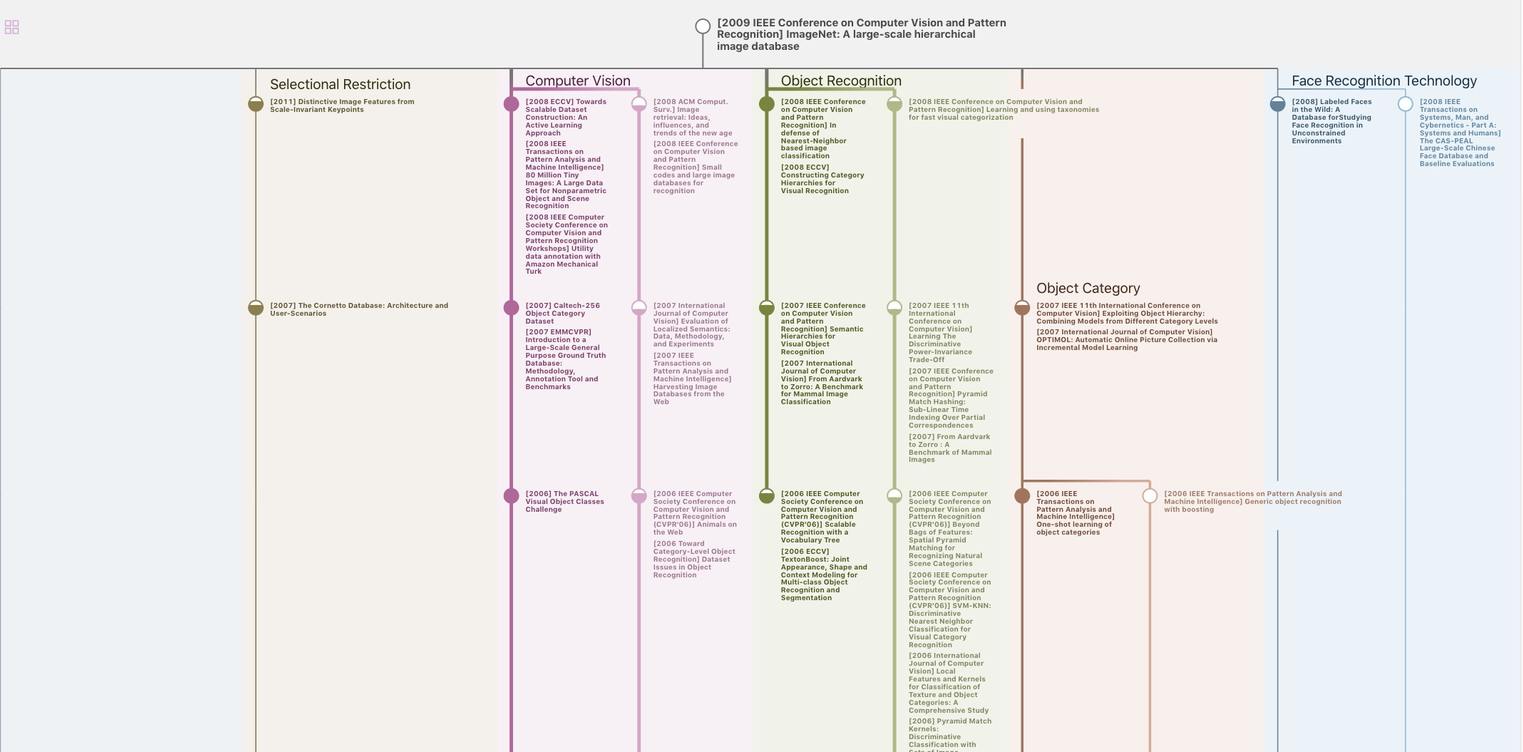
Generate MRT to find the research sequence of this paper
Chat Paper
Summary is being generated by the instructions you defined