Towards Codebook-Free Deep Probabilistic Quantization for Image Retrieval
IEEE TRANSACTIONS ON PATTERN ANALYSIS AND MACHINE INTELLIGENCE(2024)
摘要
As a classical feature compression technique, quantization is usually coupled with inverted indices for scalable image retrieval. Most quantization methods explicitly divide feature space into Voronoi cells, and quantize feature vectors in each cell into the centroids learned from data distribution. However, Voronoi decomposition is difficult to achieve discriminative space partition for semantic image retrieval. In this paper, we explore semantic-aware feature space partition by deep neural network instead of Voronoi cells. To this end, we propose a new deep probabilistic quantization method, abbreviated as DeepIndex, which constructs inverted indices without explicit centroid learning. In our method, the deep neural network takes an image as input and outputs its probability of being put into each inverted index list. During training, we progressively quantize each image into the inverted lists with the top-$T$T maximal probabilities, and calculate the reward of each trial based on retrieval accuracy. We optimize the deep neural network to maximize the probability of the inverted list with maximal reward. In this way, the retrieval performance is directly optimized, leading to a more semantically discriminative space partition than other quantization methods. The experiments on public image datasets demonstrate the effectiveness of our DeepIndex method on semantic image retrieval.
更多查看译文
关键词
Deep probabilistic quantization,image retrieval,codebook-free
AI 理解论文
溯源树
样例
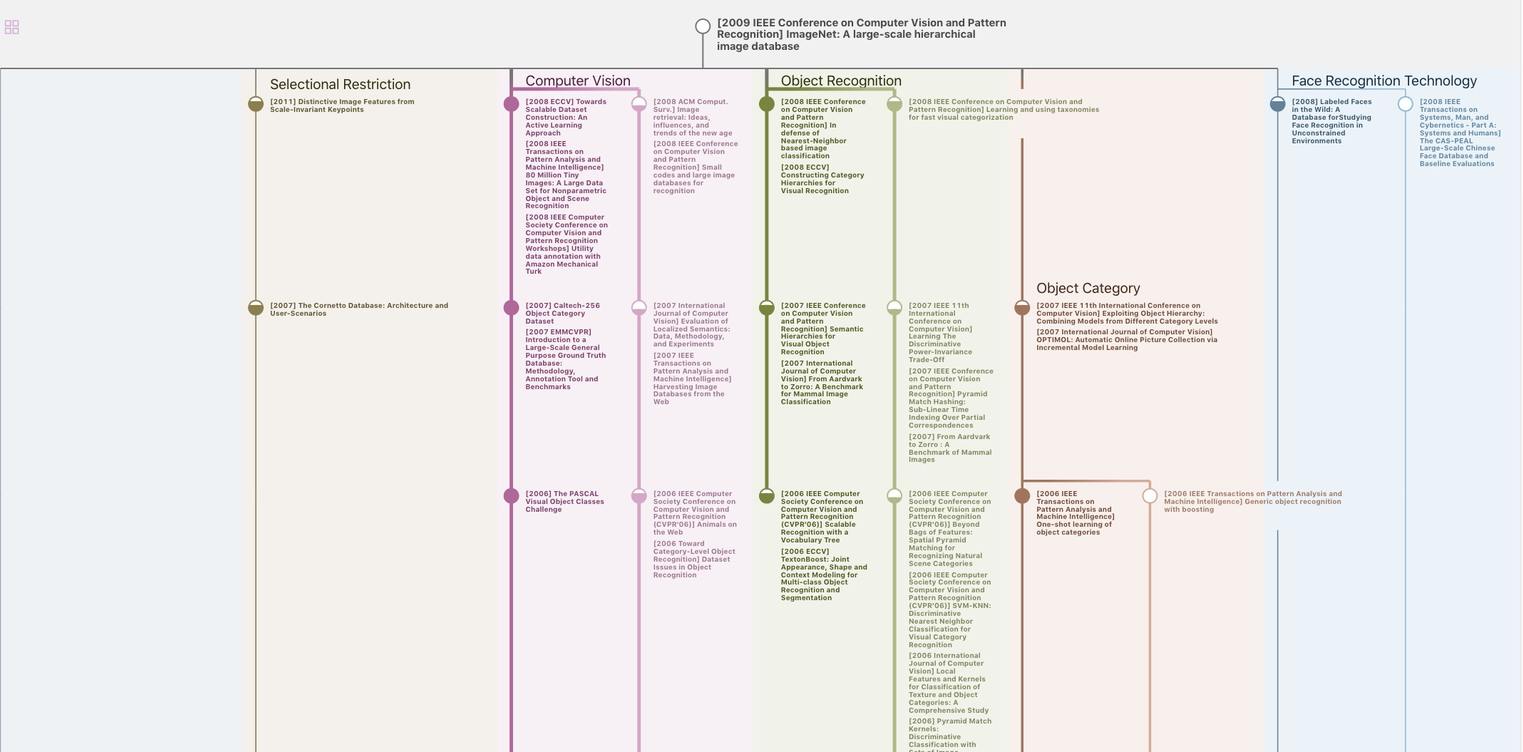
生成溯源树,研究论文发展脉络
Chat Paper
正在生成论文摘要