Development and Validation of an Interpretable Markov-embedded Multilabel Model for Predicting Risks of Multiple Postoperative Complications among Surgical Inpatients: a Multicenter Prospective Cohort Study.
INTERNATIONAL JOURNAL OF SURGERY(2024)
Key words
model interpretability,multilabel model,network analysis,postoperative complications,risk assessment
AI Read Science
Must-Reading Tree
Example
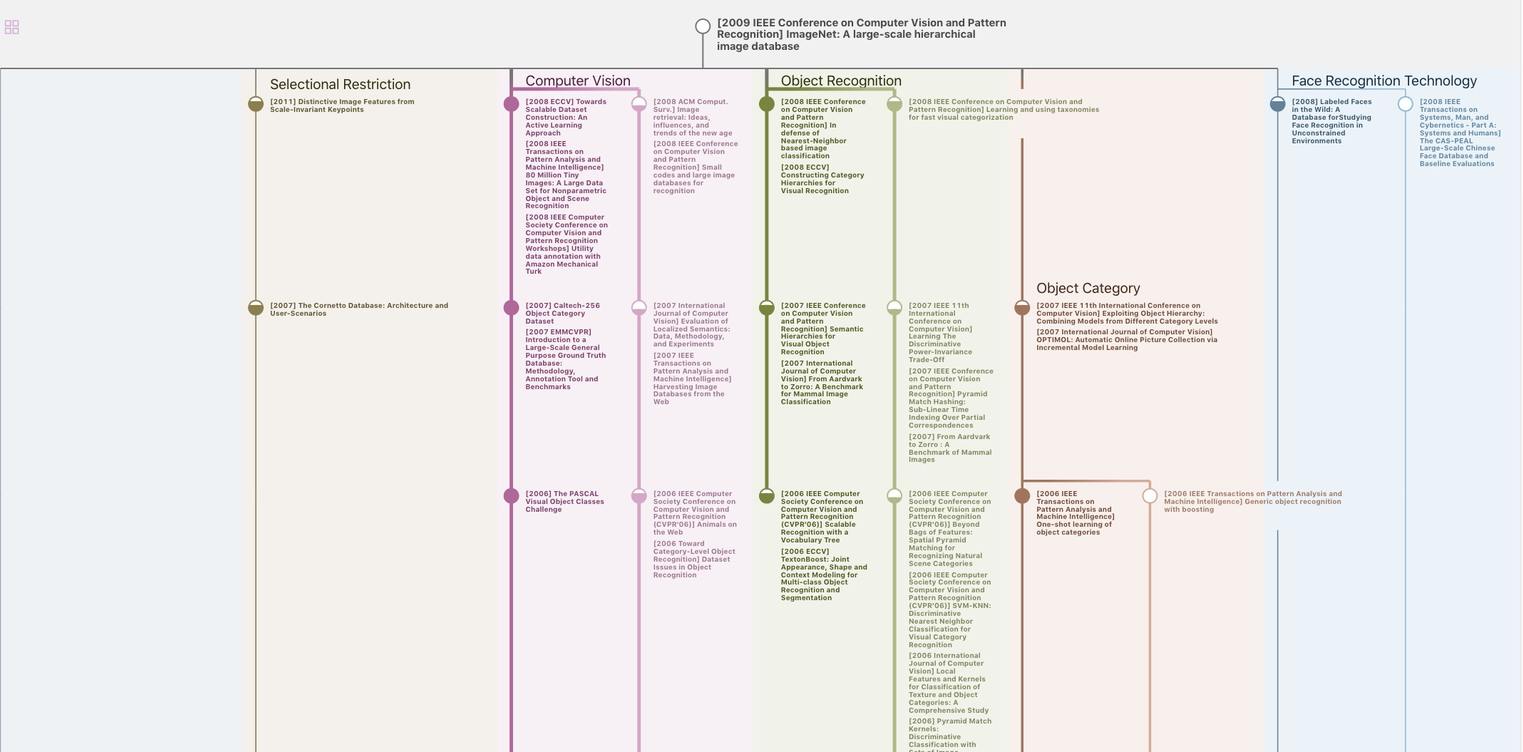
Generate MRT to find the research sequence of this paper
Chat Paper
Summary is being generated by the instructions you defined