Moment Detection in Long Tutorial Videos.
Proceedings of the IEEE/CVF International Conference on Computer Vision (ICCV)(2023)
摘要
Tutorial videos play an increasingly important role in professional development and self-directed education. For users to realise the full benefits of this medium, tutorial videos must be efficiently searchable. In this work, we focus on the task of moment detection, in which the goal is to localise the temporal window where a given event occurs within a given tutorial video. Prior work on moment detection has focused primarily on short videos (typically on videos shorter than three minutes). However, many tutorial videos are substantially longer (stretching to hours in duration), presenting significant challenges for existing moment detection approaches.To study this problem, we propose the first dataset of untrimmed, long-form tutorial videos for the task of Moment Detection called the Behance Moment Detection (BMD) dataset. BMD videos have an average duration of over one hour and are characterised by slowly evolving visual content and wide-ranging dialogue. To meet the unique challenges of this dataset, we propose a new framework, LongMoment-Detr, and demonstrate that it outperforms strong baselines. Additionally, we introduce a variation of the dataset that contains YouTube Chapter annotations and show that the features obtained by our framework can be successfully used to boost the performance on the task of chapter detection. Code and data can be found at https://github.com/ioanacroi/longmoment-detr.
更多查看译文
AI 理解论文
溯源树
样例
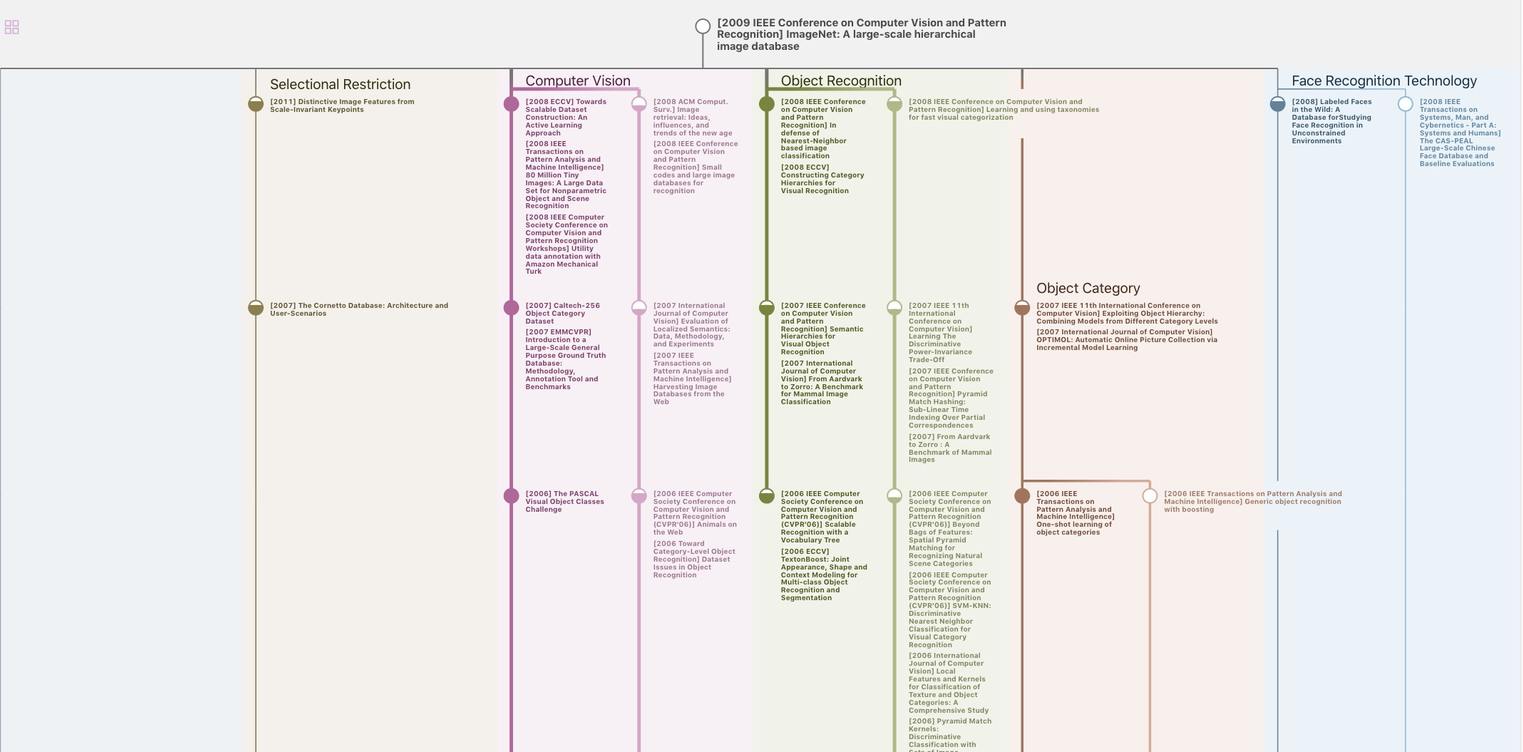
生成溯源树,研究论文发展脉络
Chat Paper
正在生成论文摘要