Template-guided Hierarchical Feature Restoration for Anomaly Detection.
Proceedings of the IEEE/CVF International Conference on Computer Vision (ICCV)(2023)
摘要
Targeting for detecting anomalies of various sizes for complicated normal patterns, we propose a Template-guided Hierarchical Feature Restoration method, which introduces two key techniques, bottleneck compression and template-guided compensation, for anomaly-free feature restoration. Specially, our framework compresses hierarchical features of an image by bottleneck structure to preserve the most crucial features shared among normal samples. We design template-guided compensation to restore the distorted features towards anomaly-free features. Particularly, we choose the most similar normal sample as the template, and leverage hierarchical features from the template to compensate the distorted features. The bottleneck could partially filter out anomaly features, while the compensation further converts the reminding anomaly features towards normal with template guidance. Finally, anomalies are detected in terms of the cosine distance between the pre-trained features of an inference image and the corresponding restored anomaly-free features. Experimental results demonstrate the effectiveness of our approach, which achieves the state-of-the-art performance on the MVTec LOCO AD dataset.
更多查看译文
AI 理解论文
溯源树
样例
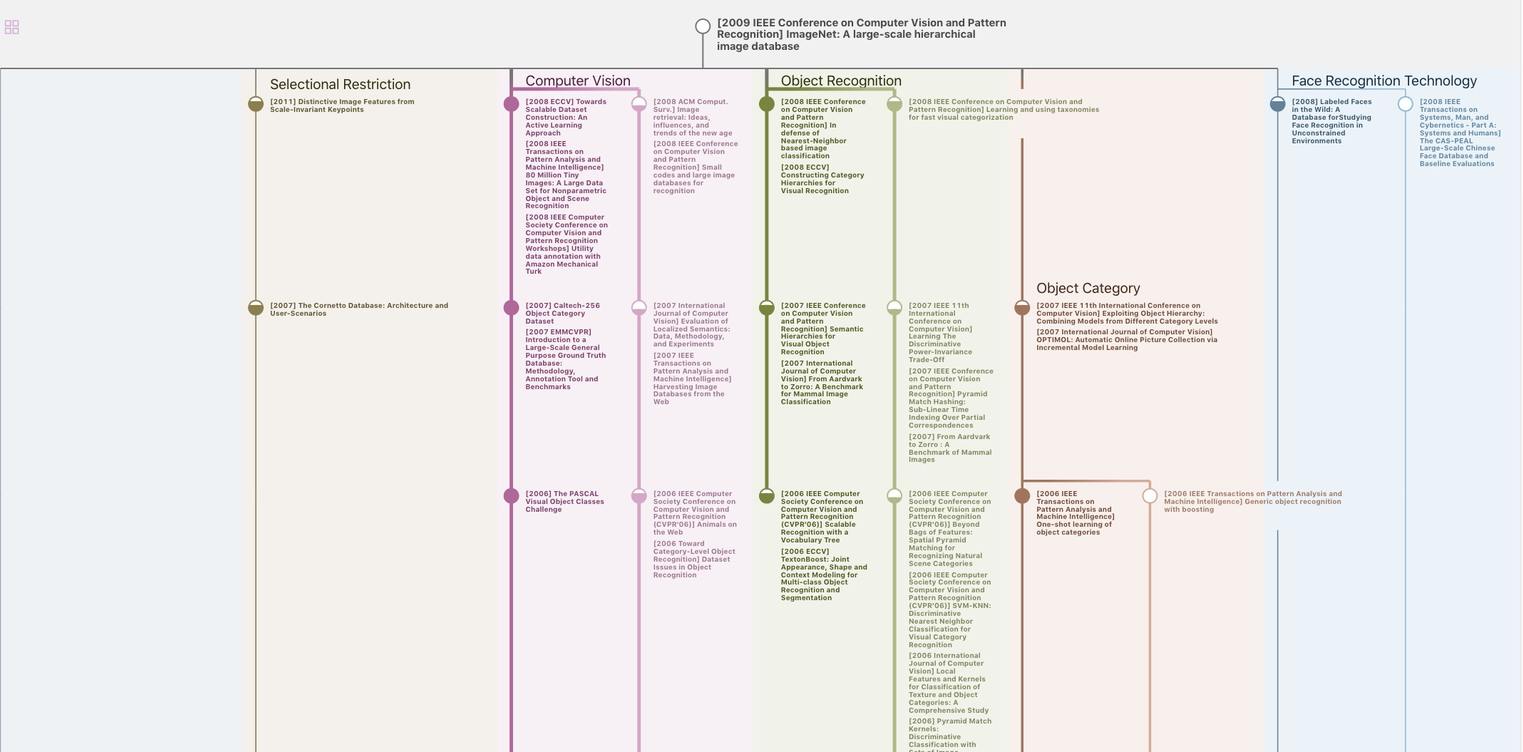
生成溯源树,研究论文发展脉络
Chat Paper
正在生成论文摘要