InfoCL: Alleviating Catastrophic Forgetting in Continual Text Classification from an Information Theoretic Perspective
EMNLP 2023(2023)
关键词
Representation Learning,Meta-Learning,Language Understanding,Clustering Analysis,Attention Mechanism
AI 理解论文
溯源树
样例
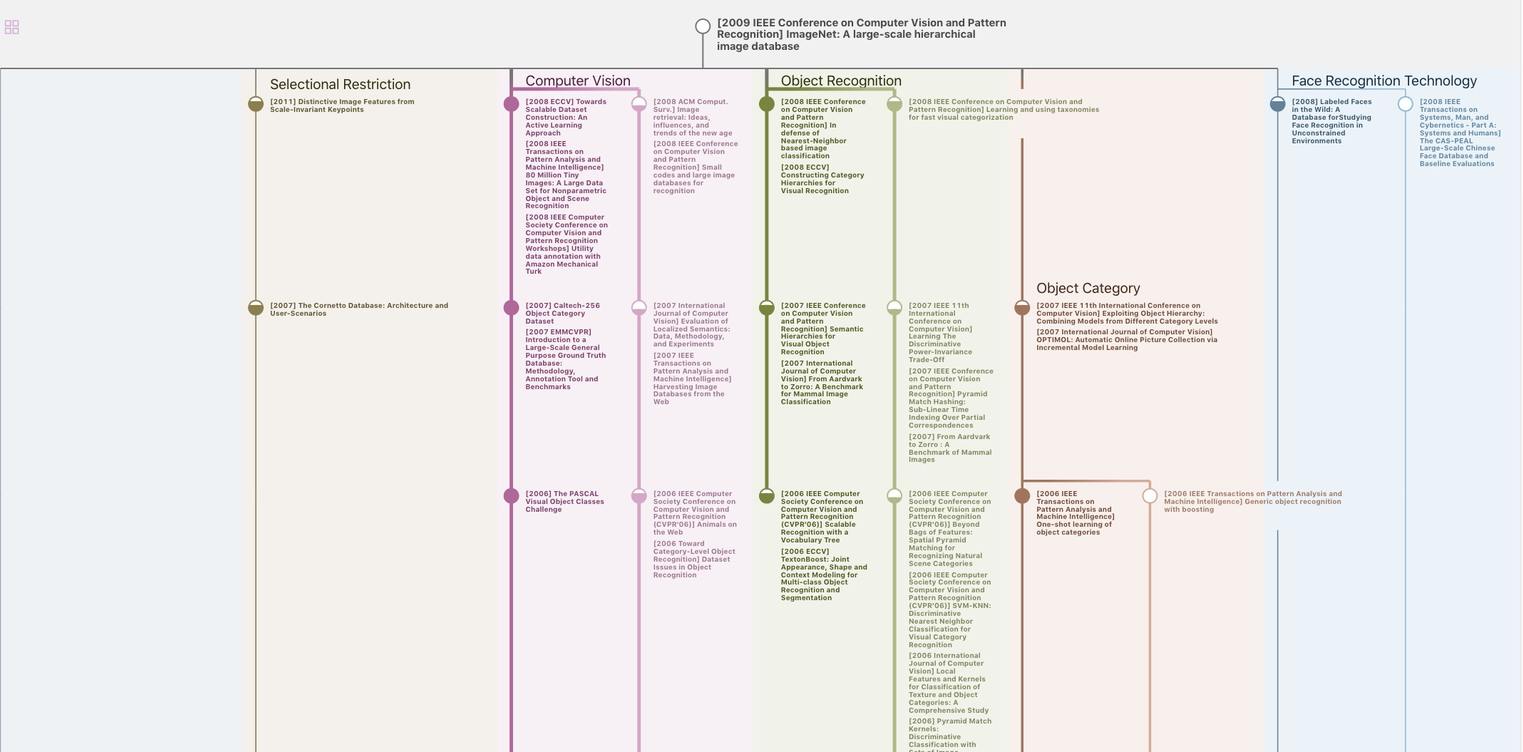
生成溯源树,研究论文发展脉络
Chat Paper
正在生成论文摘要