Learning with Real Data Without Real Labels: a Strategy for Extrapolated Full-Waveform Inversion with Field Data
GEOPHYSICAL JOURNAL INTERNATIONAL(2023)
Key words
Computational seismology,Controlled source seismology,Waveform inversion,Low-frequency extrapolation,Deep learning
AI Read Science
Must-Reading Tree
Example
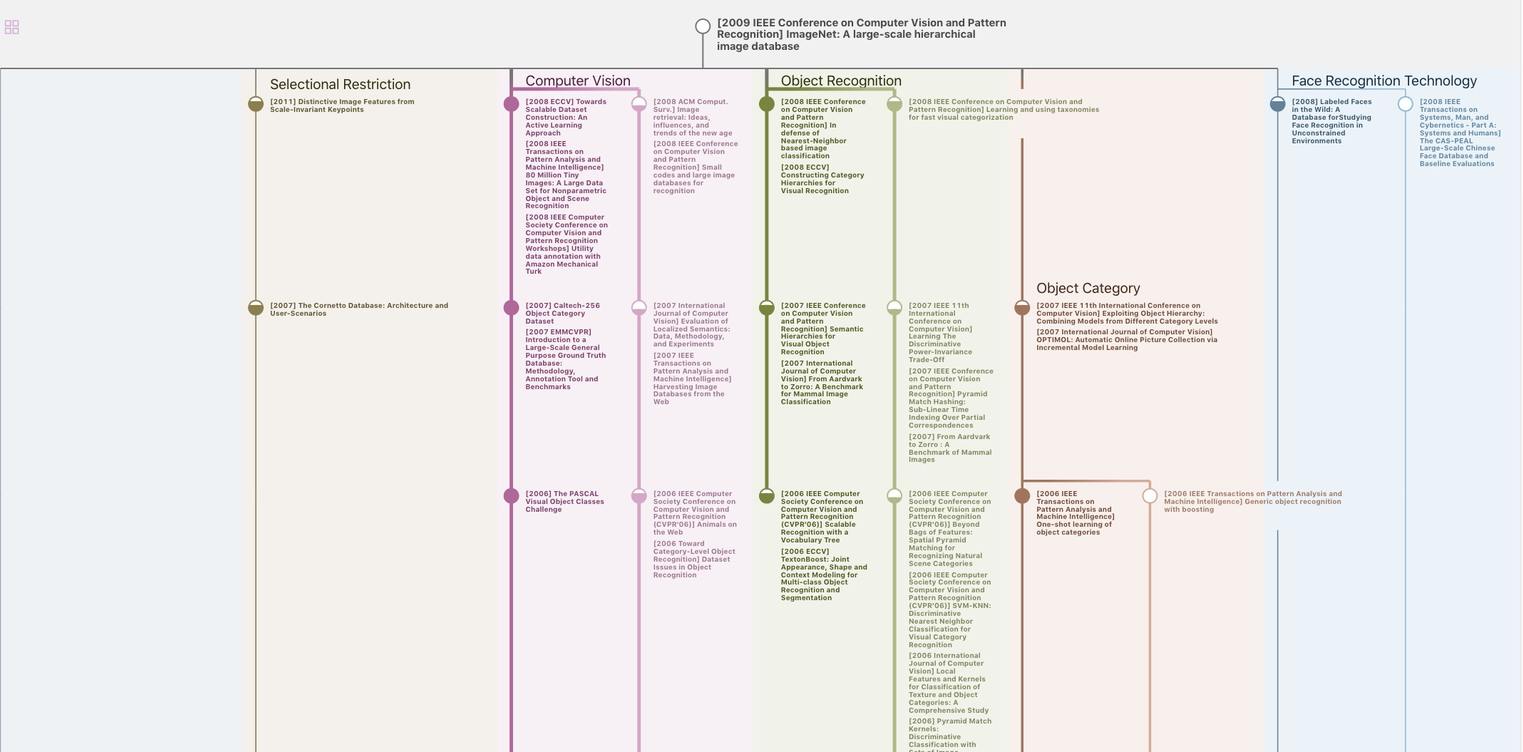
Generate MRT to find the research sequence of this paper
Chat Paper
Summary is being generated by the instructions you defined