Privacy-Protected Person Re-Identification via Virtual Samples.
IEEE Trans. Inf. Forensics Secur.(2023)
摘要
Most person re-identification (re-ID) approaches are based on representation learning of pedestrian images, which assume that the person's appearance captured by cameras in the target is fully available. However, the exposure of appearance could cause serious privacy leakages. To address this issue, we focus on a new privacy-protected person re-ID task where the person's appearance is unavailable for training. We first overcome the dilemma of lacking real person images by utilizing the virtual pedestrian samples (e.g., PersonX). Then, we introduce a composition of data augmentations to simulate real conditions, where the learned model is transferred to the real target in a black way. Specifically, the background behind, surrounding illumination, pose, scale, and attributes of pedestrians from the target scene, irrelevant to privacy, are utilized to generate virtual images. With above privacy-irrelevant information, we propose a Translation-Rendering-Sampling (TRS) framework to generate images toward the distribution of the real-world dataset (including background, pose, attribute, etc). Extensive experiments are conducted on several realistic re-ID datasets excluding the person's appearance. The experiments show that our method outperforms the baseline significantly as well as some transfer learning methods.
更多查看译文
关键词
person,samples,privacy-protected,re-identification
AI 理解论文
溯源树
样例
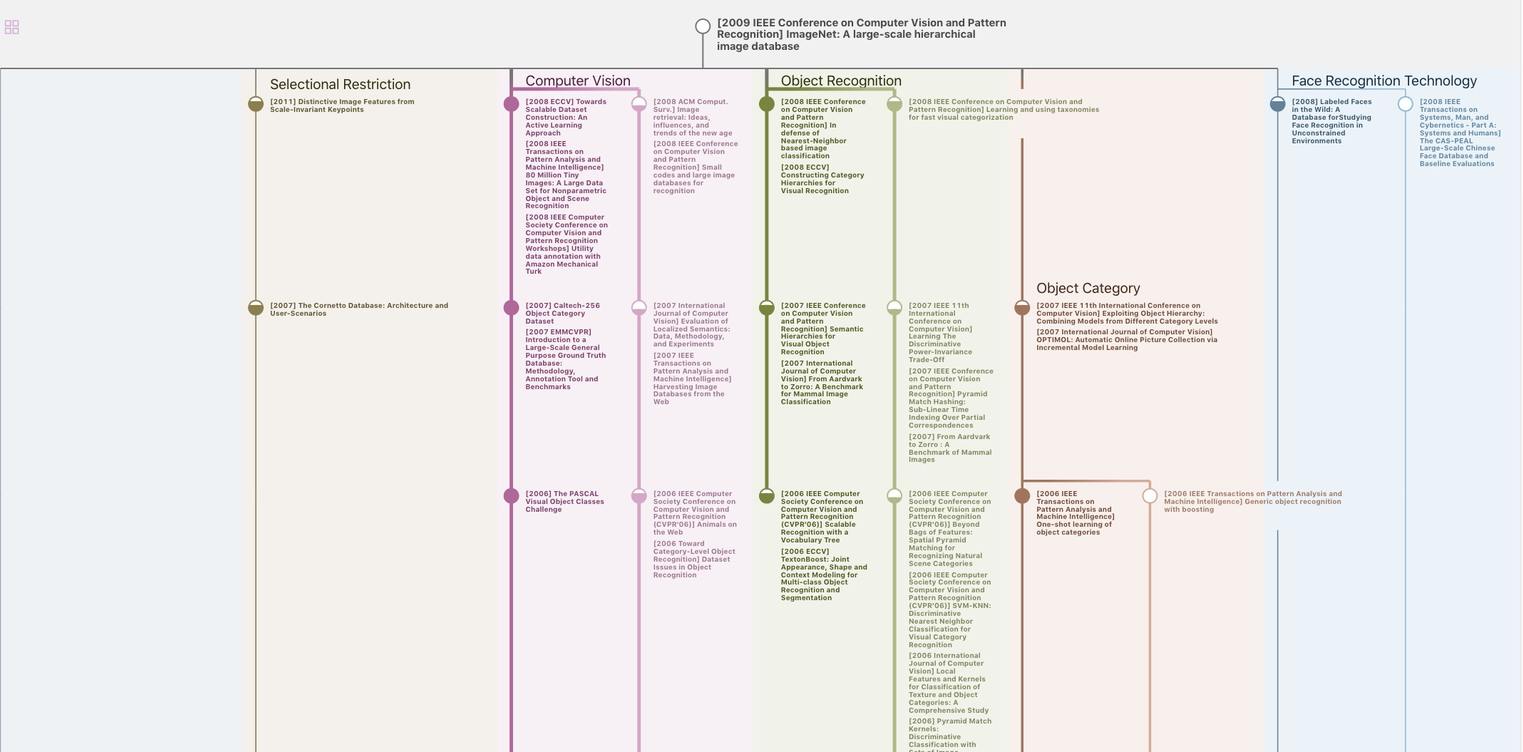
生成溯源树,研究论文发展脉络
Chat Paper
正在生成论文摘要