Ensemble of Unsupervised Learned Image Representations Based On Variational Autoencoders for Lung Adenocarcinoma Subtype Differentiation.
ISBI(2023)
摘要
Lung adenocarcinoma is a type of non-small cell lung cancer that accounts for about 40% of all lung cancers, which is divided into different molecular and histological subtypes associated with particular prognosis and treatment. Pathologists stratify for diagnosis mainly by its histo-morphological visual features and patterns, which tends to be challenging because of the nature of lung tissue, a mixture of histologically complex patterns and not having a specialized grading system. Here, an unsupervised computational approach based on an ensemble of tissue-specialized variational auto-encoders, which were trained per histopathology subtype, to build an unsupervised embedded tissue-image representation. This representation was used to train a Random Forest classifier of three lung adenocarcinoma histology subtypes (lepidic, papillary and solid), and a 2D-visually interpretable projection from the learned embedded representation. Experimental results achieve an average F-score of 0.72 +/- 0.05 in the test dataset and a well-separated 2D visual mapping of tissue subtypes.
更多查看译文
关键词
Digital Pathology, Classification, Tissue Representation, Variational Autoencoder, Lung Adenocarcinoma
AI 理解论文
溯源树
样例
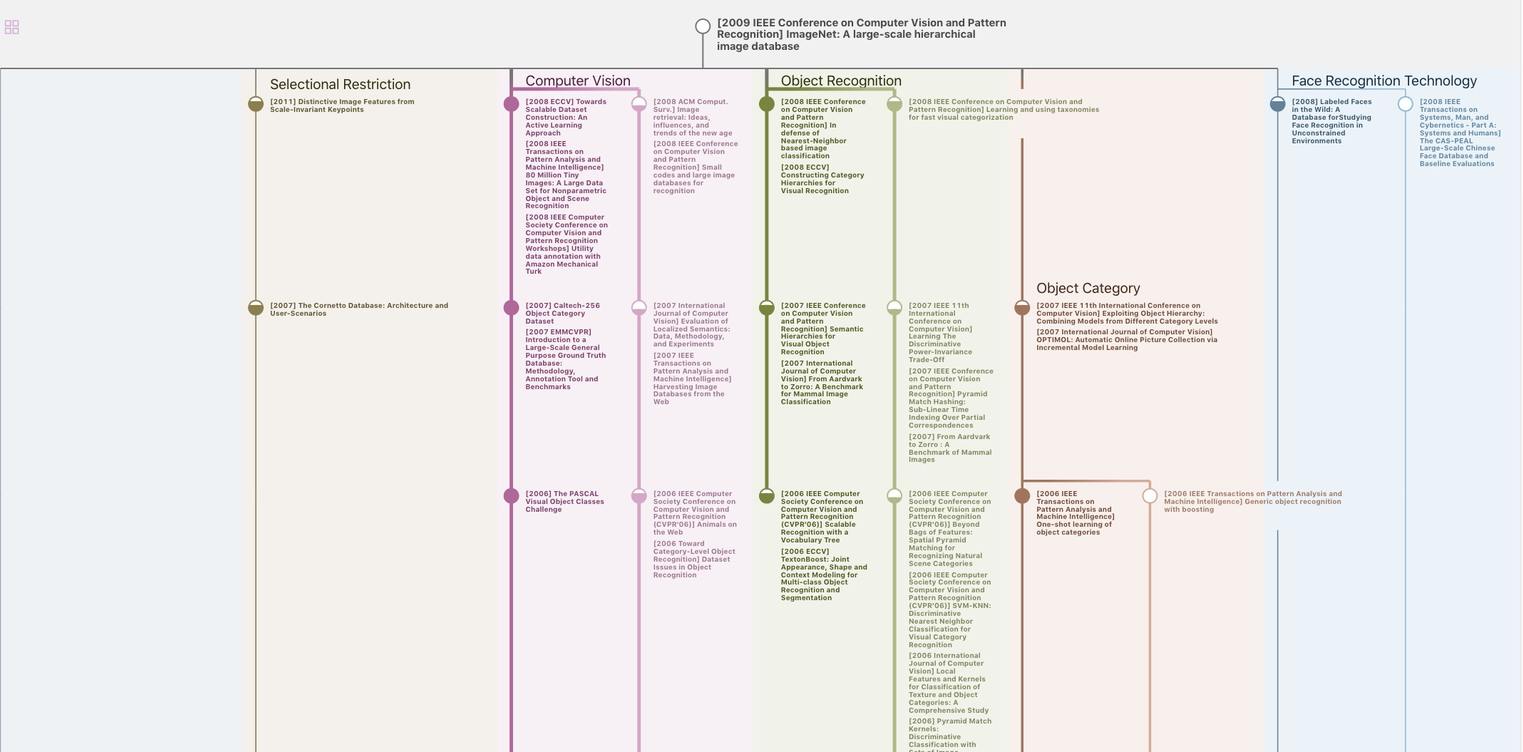
生成溯源树,研究论文发展脉络
Chat Paper
正在生成论文摘要