Acoustic-Based Detection of UAVs Using Machine Learning: Analysis of Distance and Environmental Effects.
2023 IEEE SENSORS APPLICATIONS SYMPOSIUM, SAS(2023)
Key words
UAV,drone,detection,ROC,lineal discriminant,MLP,RBFN,SVM and Random Forest
AI Read Science
Must-Reading Tree
Example
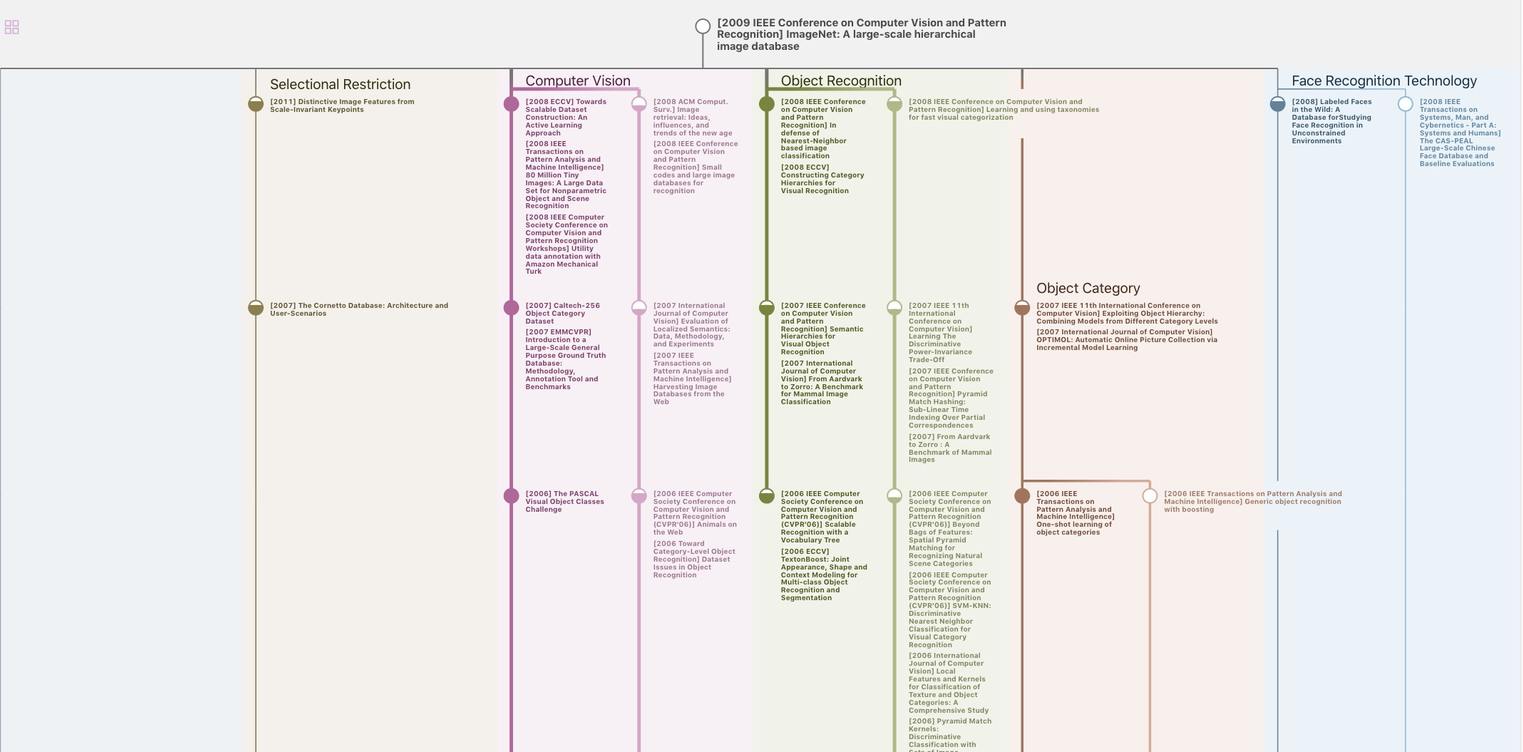
Generate MRT to find the research sequence of this paper
Chat Paper
Summary is being generated by the instructions you defined