A Dual Decision-Making Continuous Reinforcement Learning Method Based on Sim2Real
INTERNATIONAL JOURNAL OF SOFTWARE ENGINEERING AND KNOWLEDGE ENGINEERING(2023)
摘要
Continuous reinforcement learning carries potential security risks when applied in real-world scenarios, which could have significant societal implications. While its field of application is expanding, the majority of applications still remain confined to virtual environments. If only a single continuous learning method is applied to an unmanned system, it will still forget previously learned experiences, and retraining will be required when it encounters unknown environments. This reduces the learning efficiency of the unmanned system. To address these issues, some scholars have suggested prioritizing the experience playback pool and using transfer learning to apply previously learned strategies to new environments. However, these methods only alleviate the speed at which the unmanned system forgets its experiences and do not fundamentally solve the problem. Additionally, they cannot prevent dangerous actions and falling into local optima. Therefore, we propose a dual decision-making continuous learning method based on simulation to reality (Sim2Real). This method employs a knowledge body to eliminate the local optimal dilemma, and corrects bad strategies in a timely manner to ensure that the unmanned system makes the best decision every time. Our experimental results demonstrate that our method has a 30% higher success rate than other state-of-the-art methods, and the model transfer to real scenes is still highly effective.
更多查看译文
关键词
Social computing,continuous learning,reinforcement learning,simulation to reality (Sim2Real)
AI 理解论文
溯源树
样例
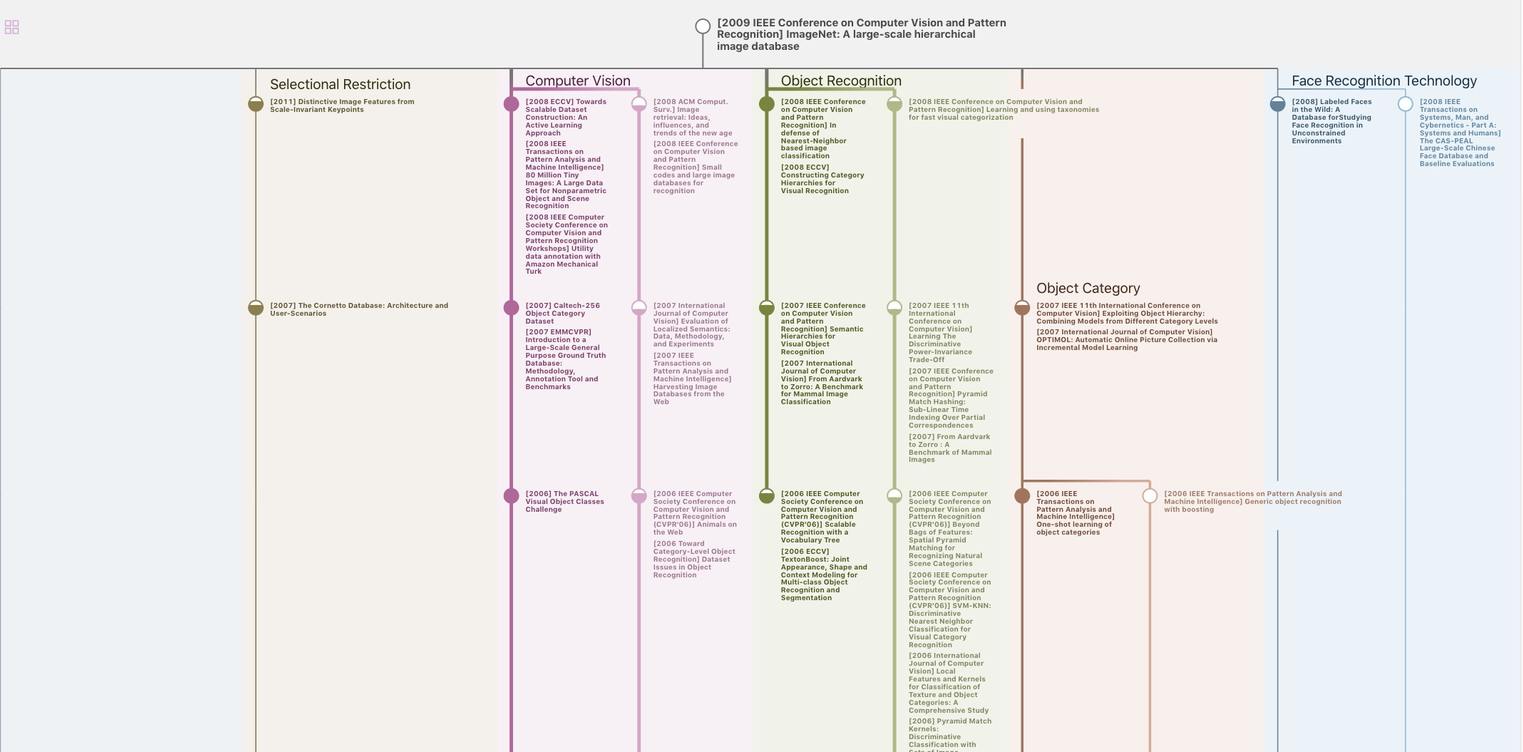
生成溯源树,研究论文发展脉络
Chat Paper
正在生成论文摘要