FedMoS: Taming Client Drift in Federated Learning with Double Momentum and Adaptive Selection.
INFOCOM(2023)
摘要
Federated learning (FL) enables massive clients to collaboratively train a global model by aggregating their local updates without disclosing raw data. Communication has become one of the main bottlenecks that prolongs the training process, especially under large model variances due to skewed data distributions. Existing efforts mainly focus on either single momentum-based gradient descent, or random client selection for potential variance reduction, yet both often lead to poor model accuracy and system efficiency. In this paper, we propose FedMoS, a communication-efficient FL framework with coupled double momentum-based update and adaptive client selection, to jointly mitigate the intrinsic variance. Specifically, FedMoS maintains customized momentum buffers on both server and client sides, which track global and local update directions to alleviate the model discrepancy. Taking momentum results as input, we design an adaptive selection scheme to provide a proper client representation during FL aggregation. By optimally calibrating clients’ selection probabilities, we can effectively reduce the sampling variance, while ensuring unbiased aggregation. Through a rigid analysis, we show that FedMoS can attain the theoretically optimal ${{\mathcal{O}}}\left({{T^{ - 2/3}}}\right)$ convergence rate. Extensive experiments using real-world datasets further validate the superiority of FedMoS, with 58%-87% communication reduction for achieving the same target performance compared to state-of-the-art techniques.
更多查看译文
关键词
adaptive client selection,adaptive selection scheme,client drift,client sides,communication-efficient FL framework,coupled double momentum-based update,customized momentum buffers,existing efforts,federated learning,FedMoS,FL aggregation,global model,intrinsic variance,local updates,main bottlenecks,massive clients,model discrepancy,model variances,momentum results,poor model accuracy,potential variance reduction,proper client representation,random client selection,raw data,sampling variance,single momentum-based gradient descent,skewed data distributions,system efficiency,training process,unbiased aggregation
AI 理解论文
溯源树
样例
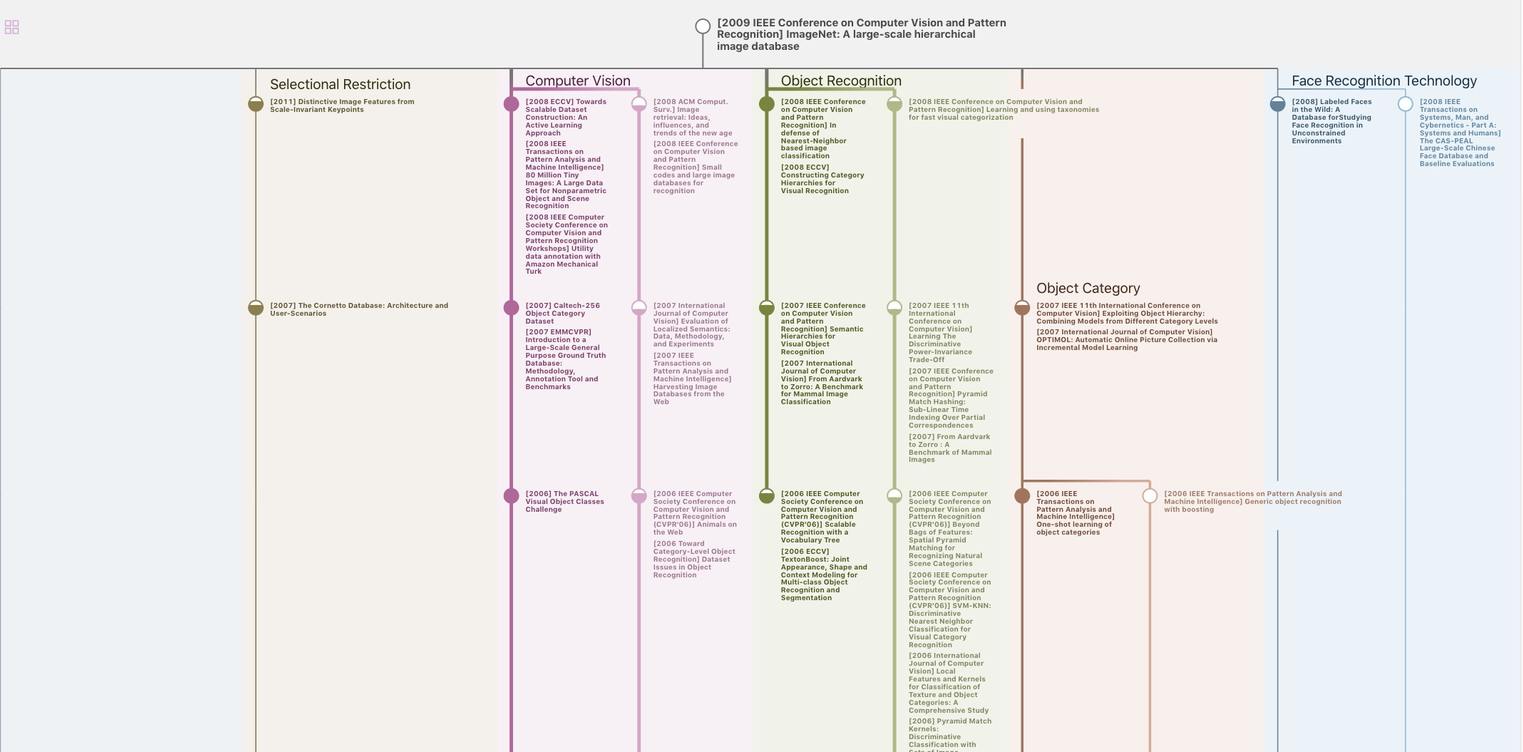
生成溯源树,研究论文发展脉络
Chat Paper
正在生成论文摘要