Fuzzy Rough Sets-Based Incremental Feature Selection for Hierarchical Classification
IEEE Transactions on Fuzzy Systems(2023)
摘要
In the era of big data, both the size and the number of features, samples, and classes continue to increase, resulting in high-dimensional classification tasks. One characteristic, among others, of big data is there exist complex structures between different classes. Hierarchical structure may be treated as the most representative one, which is mathematically depicted as a tree-like structure or directed acyclic graph. In this article, considering data in the real world may arrive dynamically, we propose an incremental feature selection approach in hierarchical classification by employing fuzzy rough set technique. First, we use the sibling strategy to reduce the scope of negative samples. Second, we present a theoretical analysis of the incremental updating of the lower approximation, positive region and dependency degree at the arrival of new samples, respectively. Third, we perform the algorithmic design of the incremental approaches. To do that, we first present two improved versions (NIDC and NIFS for short) of the existing nonincremental methods, based on NIDC, NIFS, and the aforementioned theoretical analysis, two incremental algorithms (IDU and IFS for short) are then designed to perform incremental feature selection. Finally, a numerical experiment is conducted on some commonly used datasets for hierarchical classification tasks, whose true classes are distributed to both leaf nodes and internal nodes. A comparative study is further performed to show that our approach is effective and feasible.
更多查看译文
关键词
incremental feature selection,hierarchical classification,sets-based
AI 理解论文
溯源树
样例
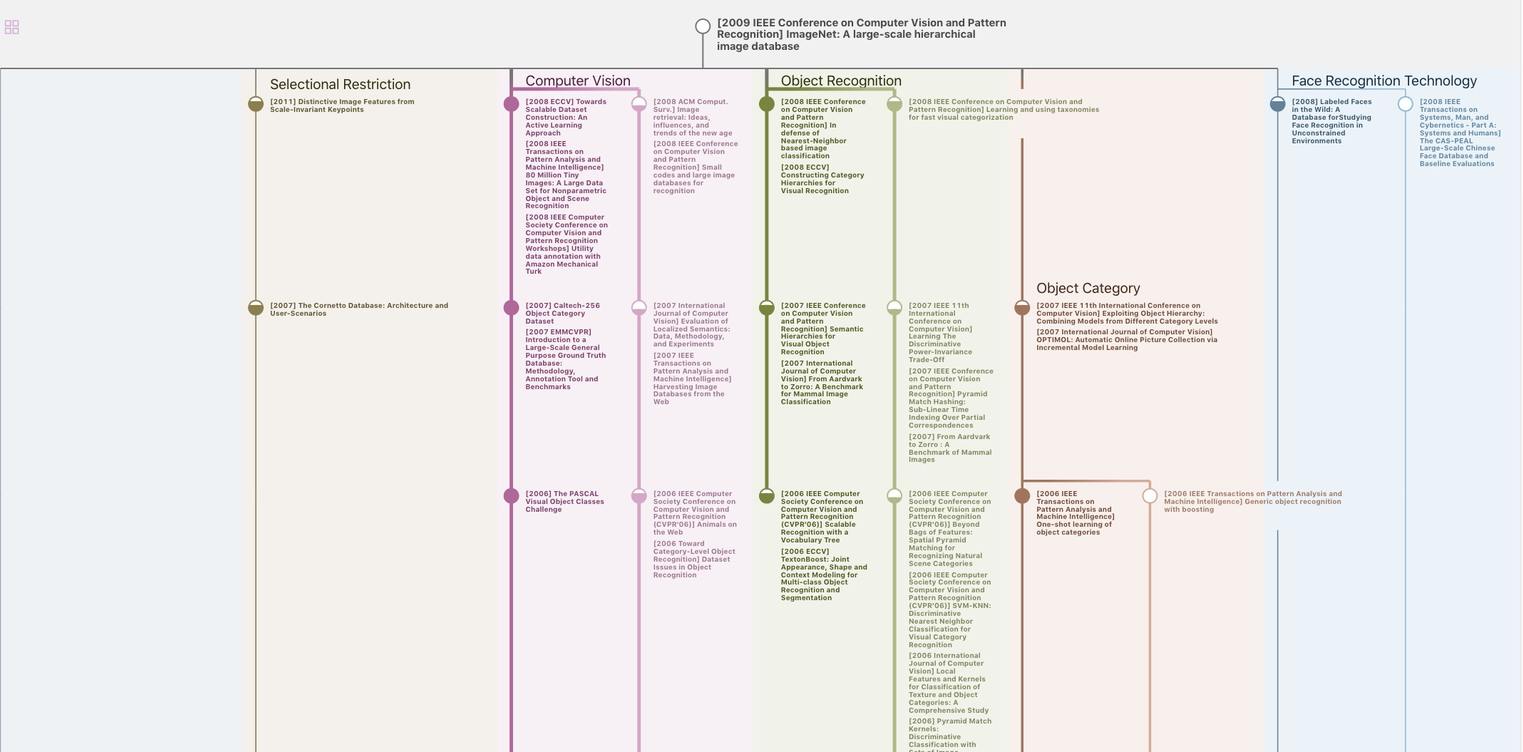
生成溯源树,研究论文发展脉络
Chat Paper
正在生成论文摘要