Axiomatic Aggregations of Abductive Explanations
THIRTY-EIGHTH AAAI CONFERENCE ON ARTIFICIAL INTELLIGENCE, VOL 38 NO 10(2024)
Key words
Description Logics
AI Read Science
Must-Reading Tree
Example
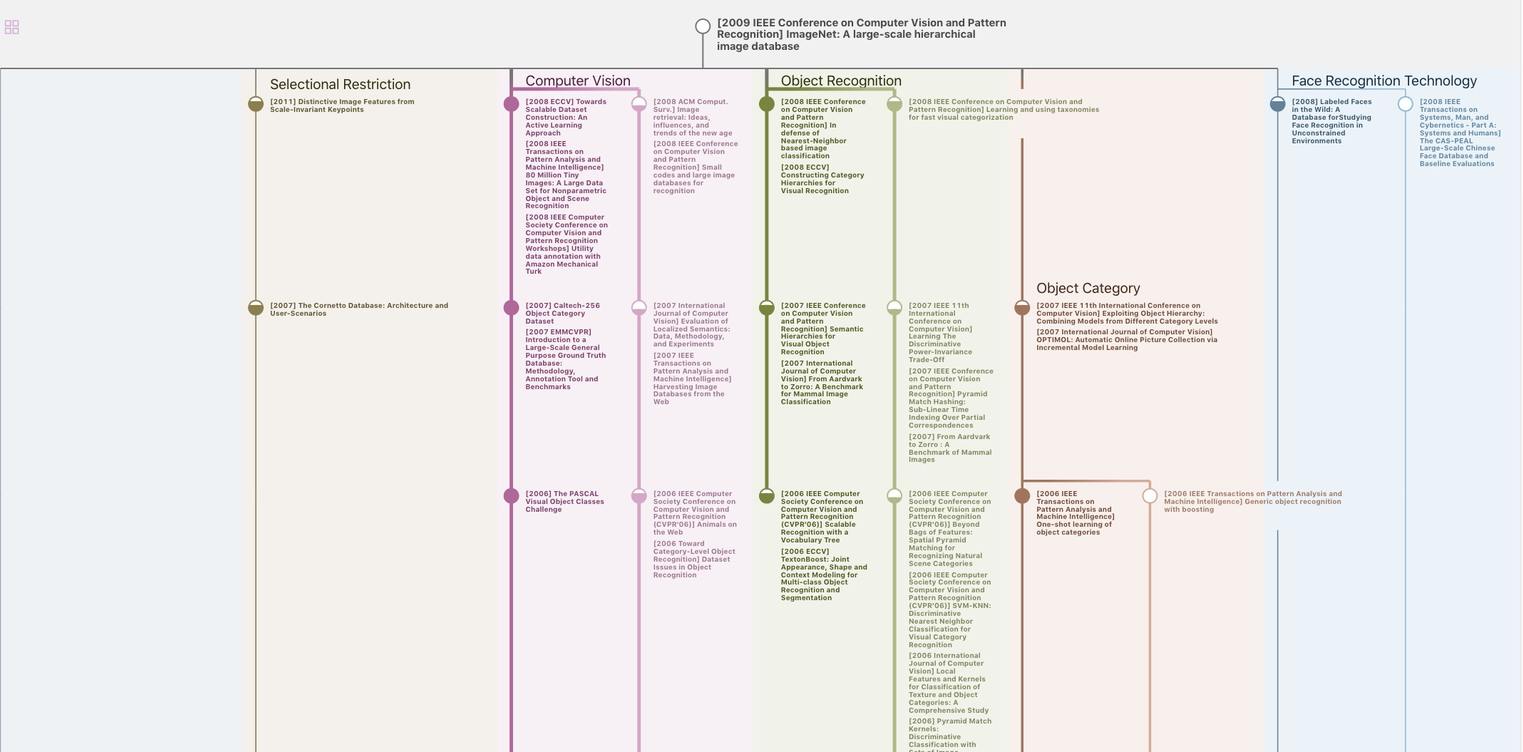
Generate MRT to find the research sequence of this paper
Chat Paper
Summary is being generated by the instructions you defined