MAD Max Beyond Single-Node: Enabling Large Machine Learning Model Acceleration on Distributed Systems
CoRR(2023)
摘要
Training and deploying large machine learning (ML) models is time-consuming and requires significant distributed computing infrastructures. Based on real-world large model training on datacenter-scale infrastructures, we show 14~32% of all GPU hours are spent on communication with no overlapping computation. To minimize the outstanding communication latency, in this work, we develop an agile performance modeling framework to guide parallelization and hardware-software co-design strategies. Using the suite of real-world large ML models on state-of-the-art GPU training hardware, we demonstrate 2.24x and 5.27x throughput improvement potential for pre-training and inference scenarios, respectively.
更多查看译文
关键词
large machine learning model
AI 理解论文
溯源树
样例
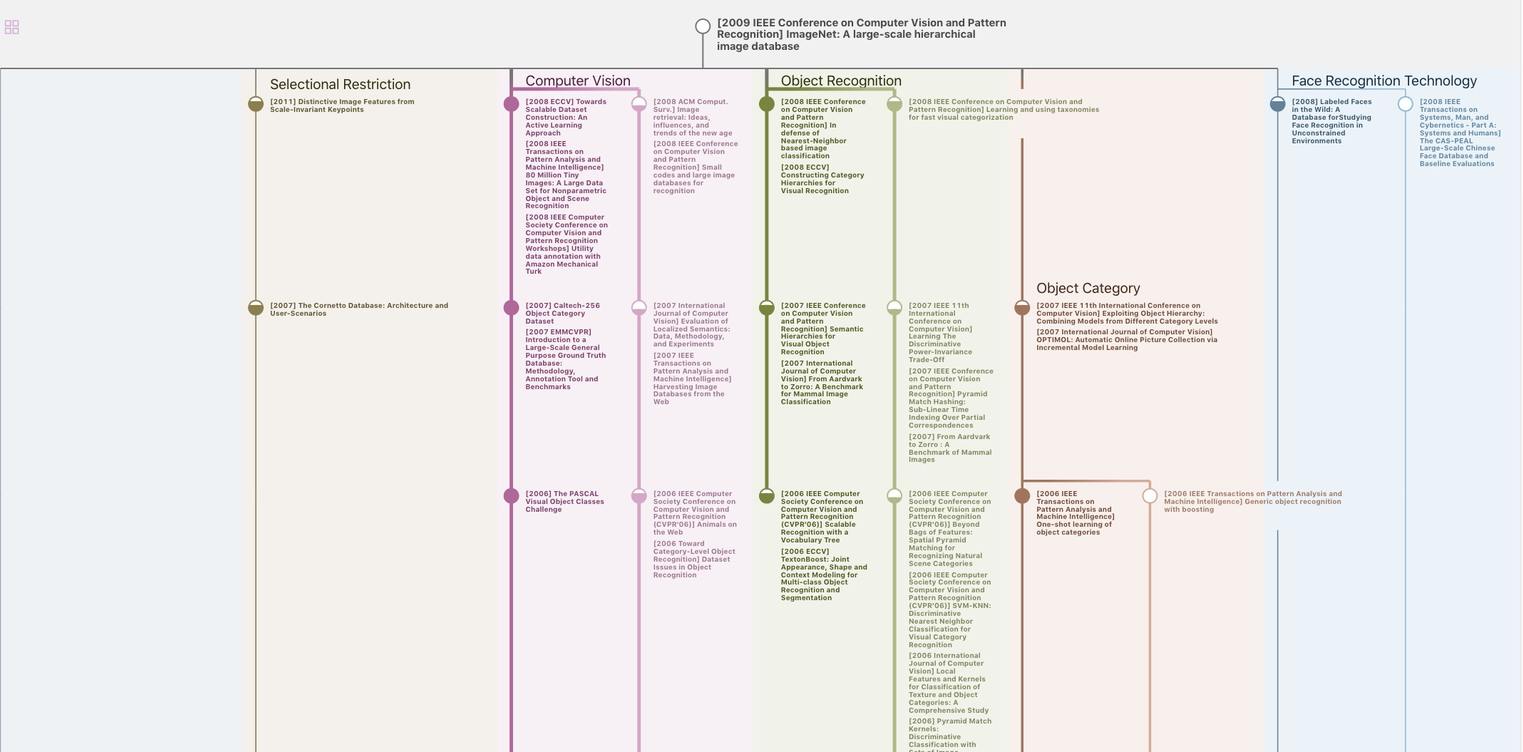
生成溯源树,研究论文发展脉络
Chat Paper
正在生成论文摘要