Knowledge Acquisition of Multi-Granularity Ordered Information Systems
APPLIED SOFT COMPUTING(2023)
关键词
Attribute reduction,Optimal scale,Dominance relation,Rough set,Multi-scale ordered system
AI 理解论文
溯源树
样例
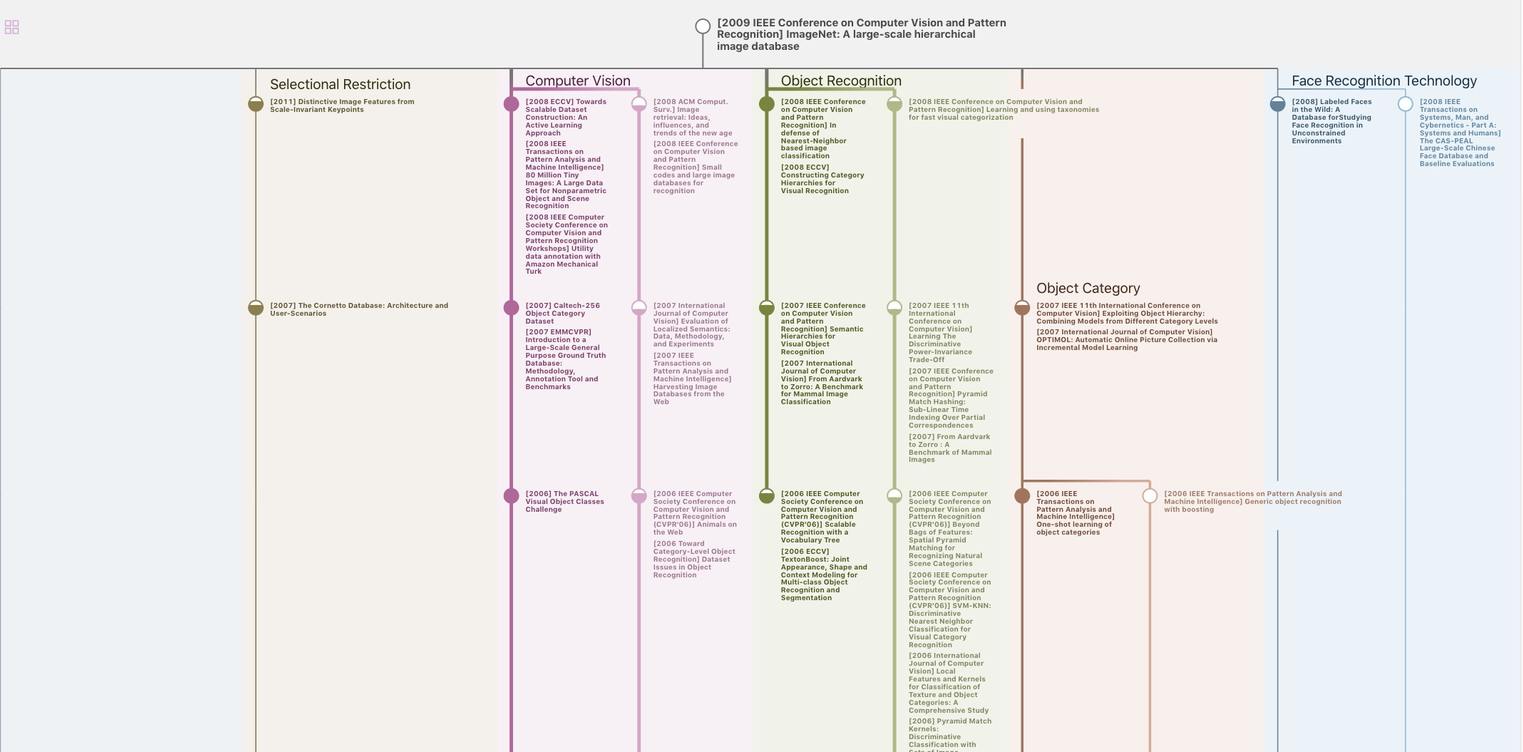
生成溯源树,研究论文发展脉络
Chat Paper
正在生成论文摘要
APPLIED SOFT COMPUTING(2023)