Finding Long-COVID: Temporal Topic Modeling of Electronic Health Records from the N3C and RECOVER Programs
medrxiv(2023)
摘要
Post-Acute Sequelae of SARS-CoV-2 infection (PASC), also known as Long-COVID, encompasses a variety of complex and varied outcomes following COVID-19 infection that are still poorly understood. We clustered over 600 million condition diagnoses from 14 million patients available through the National COVID Cohort Collaborative (N3C), generating hundreds of highly detailed clinical phenotypes. Assessing patient clinical trajectories using these clusters allowed us to identify individual conditions and phenotypes strongly increased after acute infection. We found many conditions increased in COVID-19 patients compared to controls, and using a novel method to predict patient/cluster assignment over time, we additionally found phenotypes specific to patient sex, age, wave of infection, and PASC diagnosis status. While many of these results reflect known PASC symptoms, the resolution provided by this unprecedented data scale suggests avenues for improved diagnostics and mechanistic understanding of this multifaceted disease.
### Competing Interest Statement
The authors have declared no competing interest.
### Funding Statement
The analyses described in this publication were conducted with data and tools accessed through the NCATS N3C Data Enclave https://covid.cd2h.org and N3C Attribution & Publication Policy v 1.2-2020-08-25b supported by NCATS U24 TR002306, Axle Informatics Subcontract: NCATS-P00438-B, National Institutes of Health grant NHLBI RECOVER Agreement OT2HL161847-01, and CTSA award No. UM1TR004360 from the National Center for Advancing Translational Sciences.
### Author Declarations
I confirm all relevant ethical guidelines have been followed, and any necessary IRB and/or ethics committee approvals have been obtained.
Yes
The details of the IRB/oversight body that provided approval or exemption for the research described are given below:
The Office of Human Subjects Research Institutional Review Board of Johns Hopkins University gave ethical approval for this work
I confirm that all necessary patient/participant consent has been obtained and the appropriate institutional forms have been archived, and that any patient/participant/sample identifiers included were not known to anyone (e.g., hospital staff, patients or participants themselves) outside the research group so cannot be used to identify individuals.
Yes
I understand that all clinical trials and any other prospective interventional studies must be registered with an ICMJE-approved registry, such as ClinicalTrials.gov. I confirm that any such study reported in the manuscript has been registered and the trial registration ID is provided (note: if posting a prospective study registered retrospectively, please provide a statement in the trial ID field explaining why the study was not registered in advance).
Yes
I have followed all appropriate research reporting guidelines, such as any relevant EQUATOR Network research reporting checklist(s) and other pertinent material, if applicable.
Yes
The N3C Data Enclave is managed under the authority of the NIH; information can be found at . The N3C data transfer to NCATS is performed under a Johns Hopkins University Reliance Protocol # IRB00249128 or individual site agreements with NIH. Enclave data is protected, and can be accessed for COVID-related research with an approved (1) IRB protocol and (2) Data Use Request (DUR). Enclave and data access instructions can be found at .
更多查看译文
关键词
temporal topic modeling,electronic health records,recover programs,n3c,long-covid
AI 理解论文
溯源树
样例
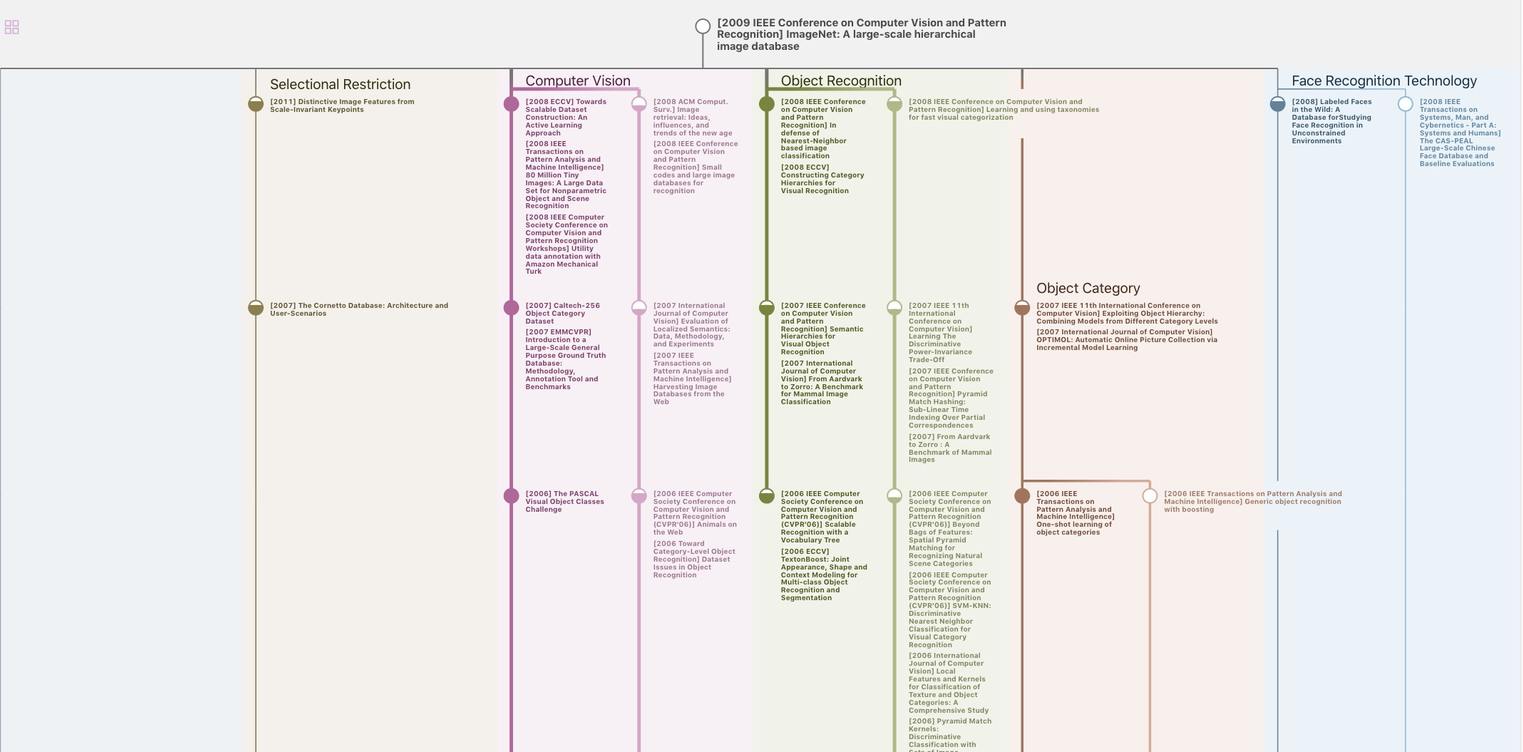
生成溯源树,研究论文发展脉络
Chat Paper
正在生成论文摘要