Gtpsum: Guided Tensor Product Framework for Abstractive Summarization
The Journal of Supercomputing(2023)
关键词
Guidance signal,Role embedding,Unfaithful problem,Syntactic errors
AI 理解论文
溯源树
样例
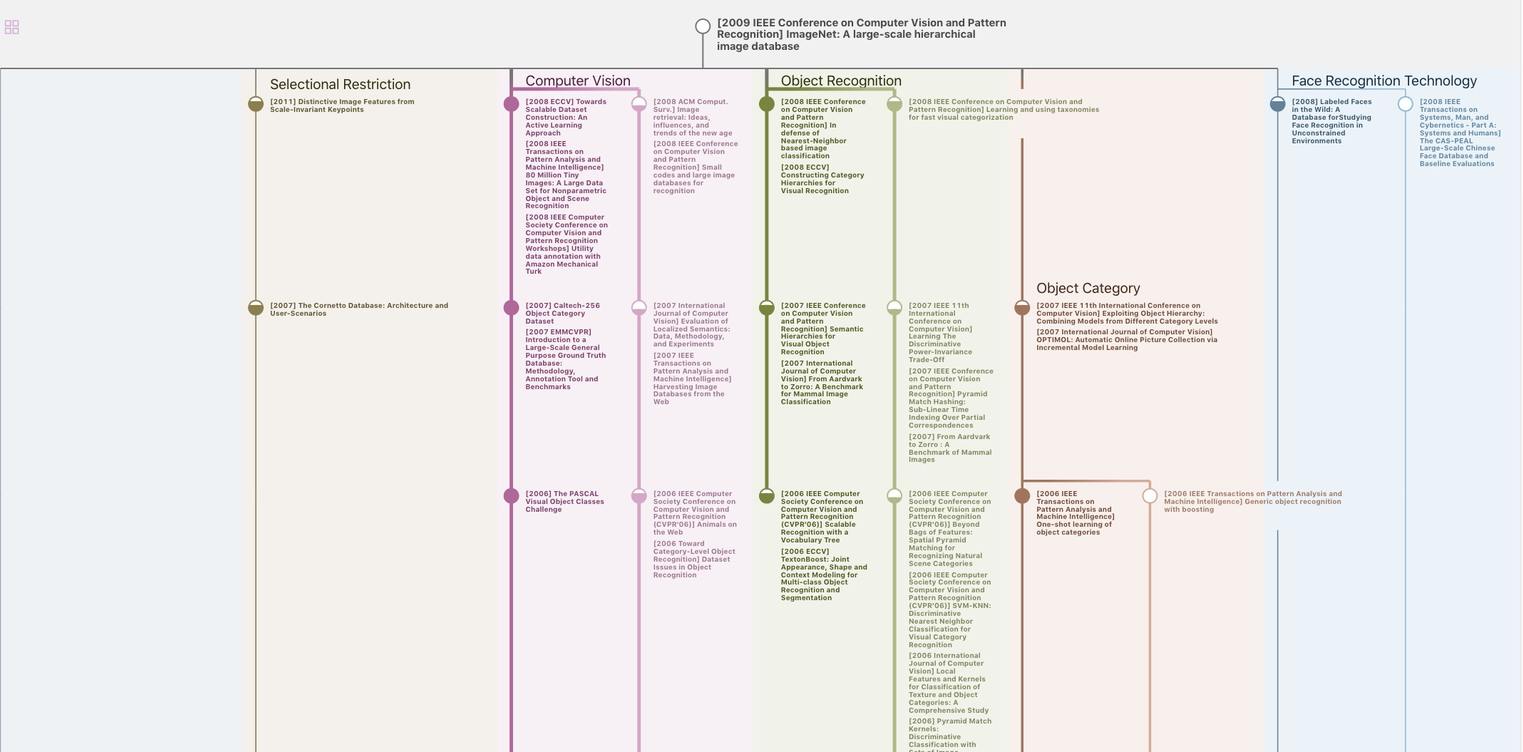
生成溯源树,研究论文发展脉络
Chat Paper
正在生成论文摘要
The Journal of Supercomputing(2023)