SafeShift: Safety-Informed Distribution Shifts for Robust Trajectory Prediction in Autonomous Driving
2024 35TH IEEE INTELLIGENT VEHICLES SYMPOSIUM, IEEE IV 2024(2024)
Key words
Domain Shift,Autonomous Vehicles,Robust Predictor,Trajectory Prediction,Prediction Model,Collision,Counterfactual,Real-world Datasets,Remediation Strategies,Individual Characteristics,Individual Scores,Social Characteristics,Pedestrian,Cross-entropy Loss,Traffic Congestion,Prediction Task,External Agents,Confidence Value,Motion Prediction,Alternative Metrics,Vulnerable Road Users,Meta Information,Robust Prediction Model,Time Headway
AI Read Science
Must-Reading Tree
Example
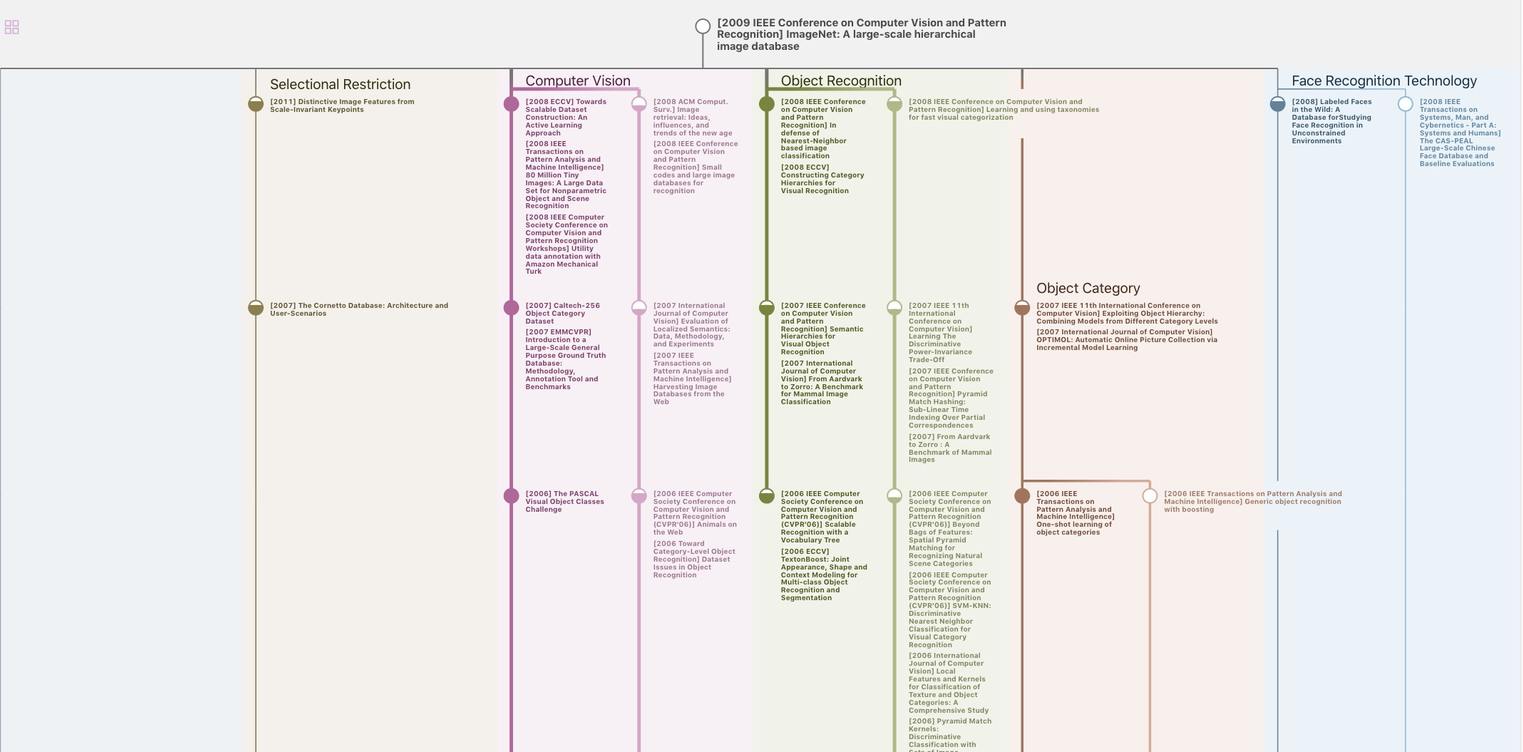
Generate MRT to find the research sequence of this paper
Chat Paper
Summary is being generated by the instructions you defined