Fast Fixed Granular-Ball for Attribute Reduction in Label Noise Environments and Its Application in Medical Diagnosis
INTERNATIONAL JOURNAL OF MACHINE LEARNING AND CYBERNETICS(2024)
Key words
Fixed granular-ball,Neighborhood rough set,Attribute reduction,Feature selection,Label noise,Rough set
AI Read Science
Must-Reading Tree
Example
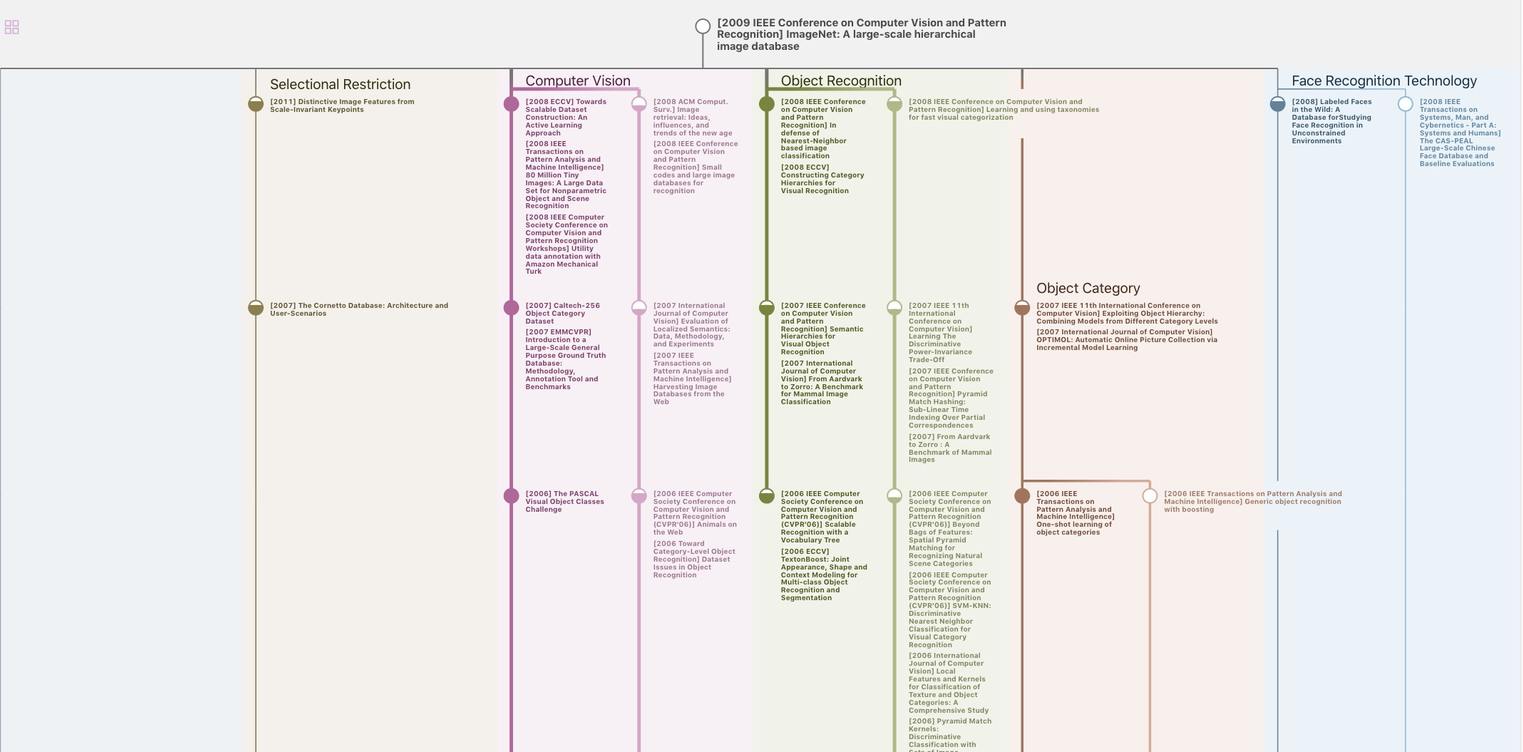
Generate MRT to find the research sequence of this paper
Chat Paper
Summary is being generated by the instructions you defined