Multi-Class Segmentation of Dark-Grown Arabidopsis Seedlings with U-Net
2023 11th International Conference on Bioinformatics and Computational Biology (ICBCB)(2023)
摘要
Plant organ phenotyping represents a powerful tool to investigate the effects of biotic and abiotic factors on plant growth and development. Phenotyping typically involves the initial capture of high-resolution images of the plant of interest and the subsequent measurement of different morphometric parameters of specific plant organs. This second step is typically very time consuming and difficult to automate. To deal with this bottleneck, we developed a multi-class segmentation model based on U-shape network to identify hypocotyls and roots of young seedlings of the reference plant Arabidopsis thaliana. We applied a balanced cross entropy loss function to learn an alternative optimal network structure for this multi-class segmentation task. We evaluated our segmentation machine using 66 images of the wild-type Arabidopsis strain Col-0, as well as 34 images of the highly agravitropic and morphologically distinct mutant strain, aux1-7. Our model achieved a mean BFscore of 0.81 for Col-0 seedlings and 0.75 for aux1-7 mutant seedlings on the test dataset. Our model was also able to maintain accuracy in these two morphologically different genotypes suggesting that our segmentation procedure could be successfully applied to Arabidopsis seedlings showing broad morphological differences due to their genotype or treatment conditions. Appropriate segmentation is the first step in identifying phenotypic changes under hormone-mediated stress response. The identified growth parameters will be useful to identify the response associated with both abiotic and biotic stresses, which include but not limited to drought stress, heat stress, and the presence of pests or pathogens. The quantification of these parameters will aid assessment of genetic factors that contribute to the stress response.
更多查看译文
关键词
Multi-class segmentation,plant phenotype,U-Net,encoder-decoder,deep learning
AI 理解论文
溯源树
样例
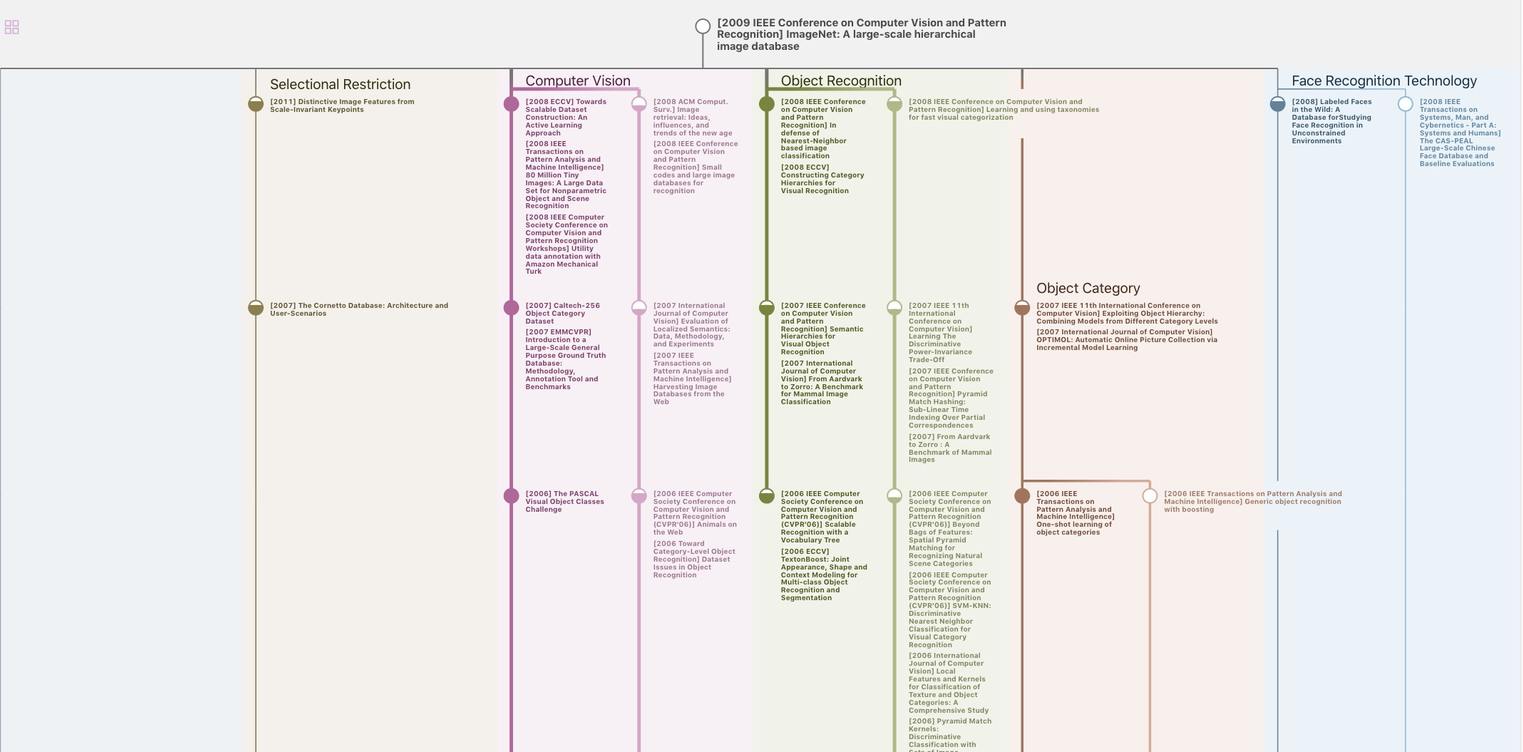
生成溯源树,研究论文发展脉络
Chat Paper
正在生成论文摘要