Beta quantile regression for robust estimation of uncertainty in the presence of outliers
ICASSP 2024 - 2024 IEEE International Conference on Acoustics, Speech and Signal Processing (ICASSP)(2023)
摘要
Quantile Regression (QR) can be used to estimate aleatoric uncertainty in deep neural networks and can generate prediction intervals. Quantifying uncertainty is particularly important in critical applications such as clinical diagnosis, where a realistic assessment of uncertainty is essential in determining disease status and planning the appropriate treatment. The most common application of quantile regression models is in cases where the parametric likelihood cannot be specified. Although quantile regression is quite robust to outlier response observations, it can be sensitive to outlier covariate observations (features). Outlier features can compromise the performance of deep learning regression problems such as style translation, image reconstruction, and deep anomaly detection, potentially leading to misleading conclusions. To address this problem, we propose a robust solution for quantile regression that incorporates concepts from robust divergence. We compare the performance of our proposed method with (i) least trimmed quantile regression and (ii) robust regression based on the regularization of case-specific parameters in a simple real dataset in the presence of outlier. These methods have not been applied in a deep learning framework. We also demonstrate the applicability of the proposed method by applying it to a medical imaging translation task using diffusion models.
更多查看译文
关键词
Quantile regression,Diffusion models,Robust divergence
AI 理解论文
溯源树
样例
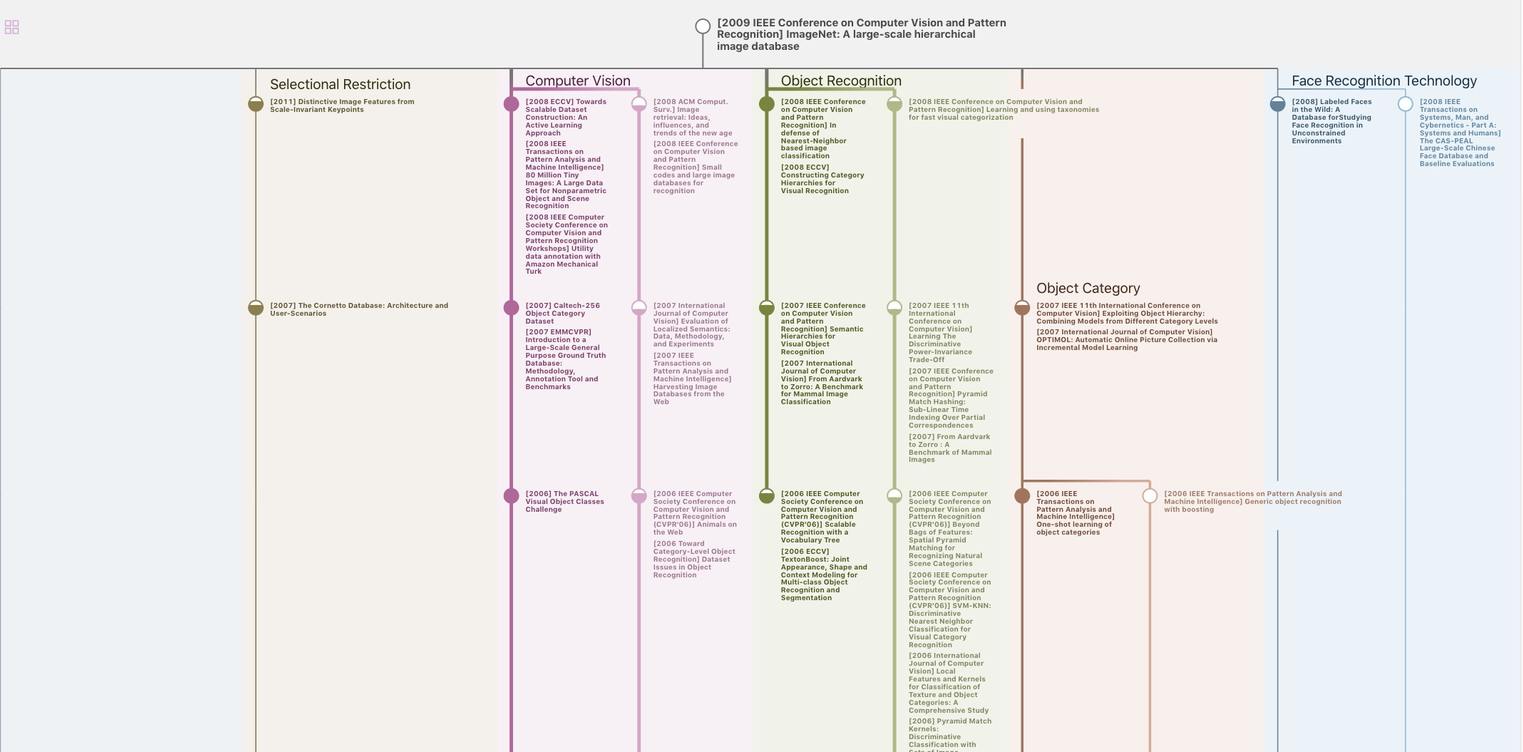
生成溯源树,研究论文发展脉络
Chat Paper
正在生成论文摘要