sasdim: self-adaptive noise scaling diffusion model for spatial time series imputation
CoRR(2023)
摘要
Spatial time series imputation is critically important to many real applications such as intelligent transportation and air quality monitoring. Although recent transformer and diffusion model based approaches have achieved significant performance gains compared with conventional statistic based methods, spatial time series imputation still remains as a challenging issue due to the complex spatio-temporal dependencies and the noise uncertainty of the spatial time series data. Especially, recent diffusion process based models may introduce random noise to the imputations, and thus cause negative impact on the model performance. To this end, we propose a self-adaptive noise scaling diffusion model named SaSDim to more effectively perform spatial time series imputation. Specially, we propose a new loss function that can scale the noise to the similar intensity, and propose the across spatial-temporal global convolution module to more effectively capture the dynamic spatial-temporal dependencies. Extensive experiments conducted on three real world datasets verify the effectiveness of SaSDim by comparison with current state-of-the-art baselines.
更多查看译文
关键词
series imputation,diffusion model,spatial time
AI 理解论文
溯源树
样例
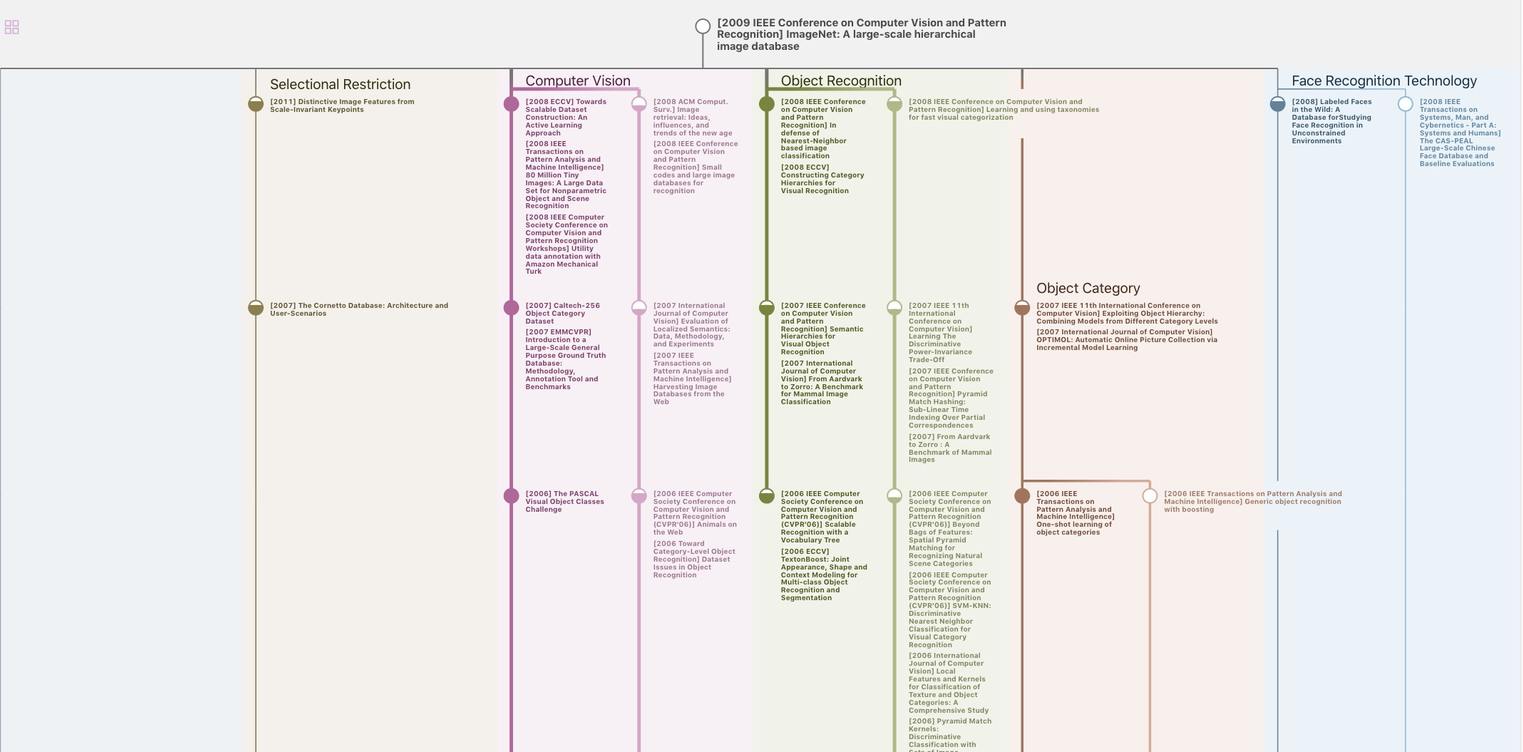
生成溯源树,研究论文发展脉络
Chat Paper
正在生成论文摘要