A Prior-Guided Generative Adversarial Net for Semantically Strict Ultrasound Images Augmentation.
ADVANCED INTELLIGENT COMPUTING TECHNOLOGY AND APPLICATIONS, ICIC 2023, PT III(2023)
Key words
Ultrasound Images,Prior Guidance,Image Attribute Editing,Data Augmentation
AI Read Science
Must-Reading Tree
Example
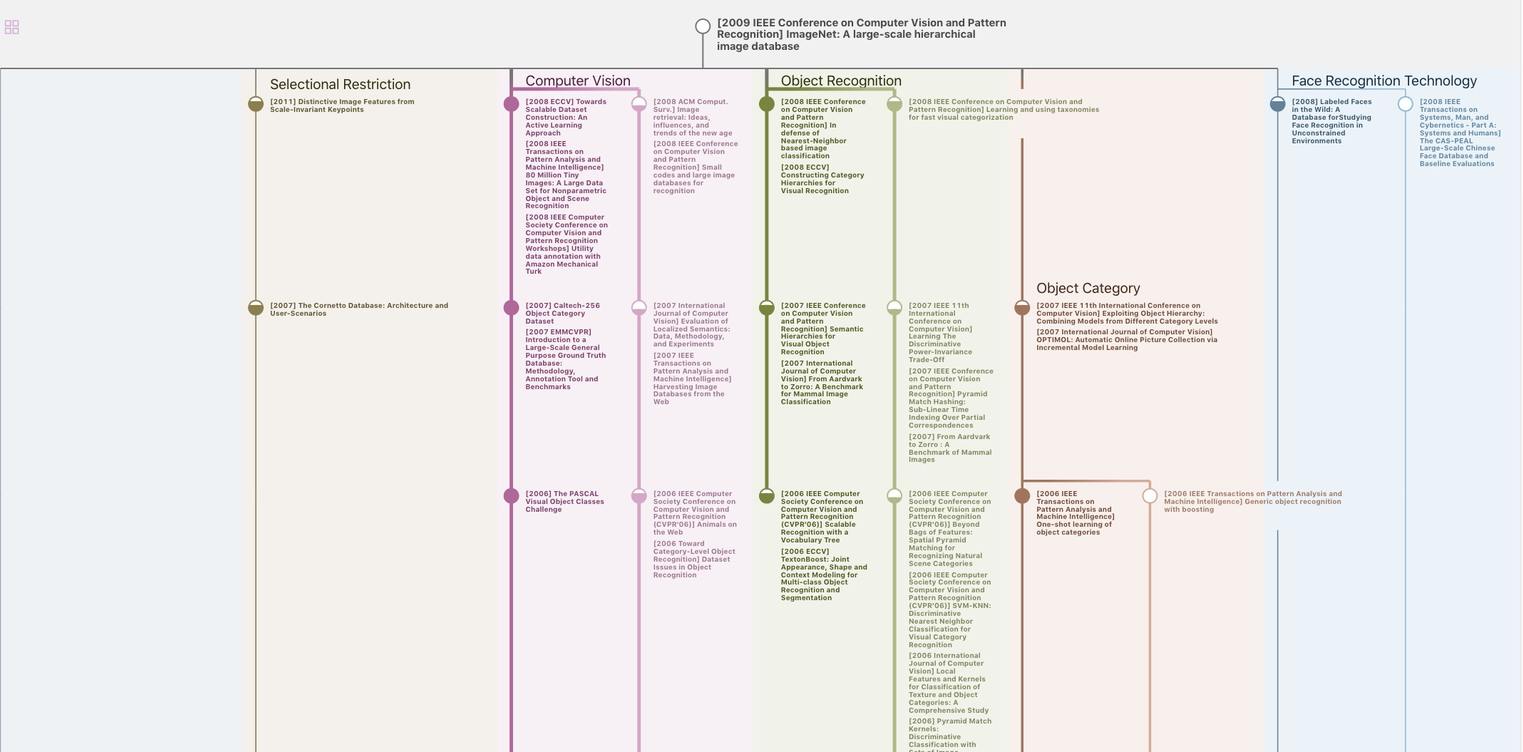
Generate MRT to find the research sequence of this paper
Chat Paper
Summary is being generated by the instructions you defined