Automated Cell Counting System Using Improved Implicit Activation Based U-Net (Ia-U-net)
2023 IEEE 5th Eurasia Conference on Biomedical Engineering, Healthcare and Sustainability (ECBIOS)(2023)
Key words
deep learning,U-net,automated cell counting
AI Read Science
Must-Reading Tree
Example
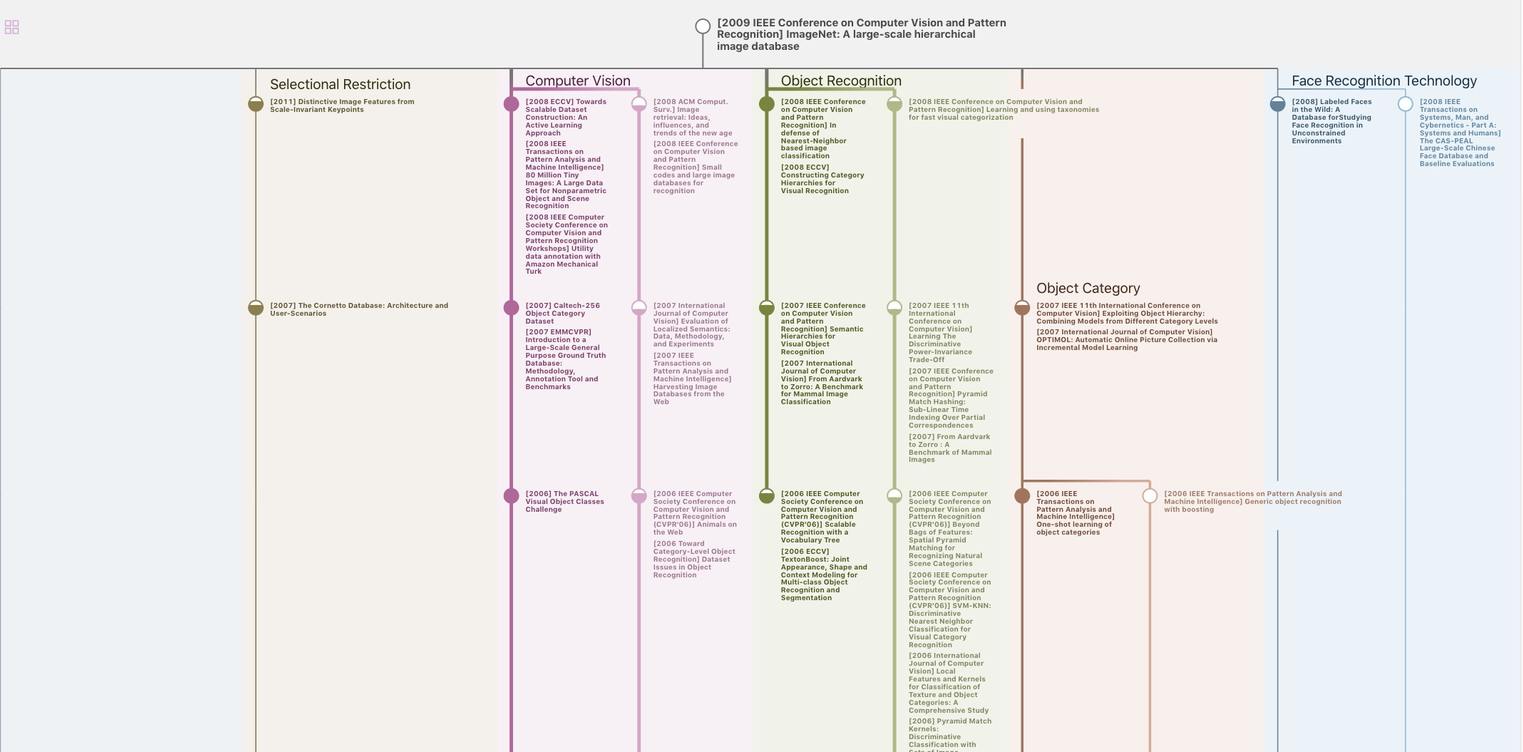
Generate MRT to find the research sequence of this paper
Chat Paper
Summary is being generated by the instructions you defined