Greedy-Based Online Fair Allocation with Adversarial Input: Enabling Best-of-Many-Worlds Guarantees
THIRTY-EIGHTH AAAI CONFERENCE ON ARTIFICIAL INTELLIGENCE, VOL 38 NO 9(2024)
Key words
Federated Learning
AI Read Science
Must-Reading Tree
Example
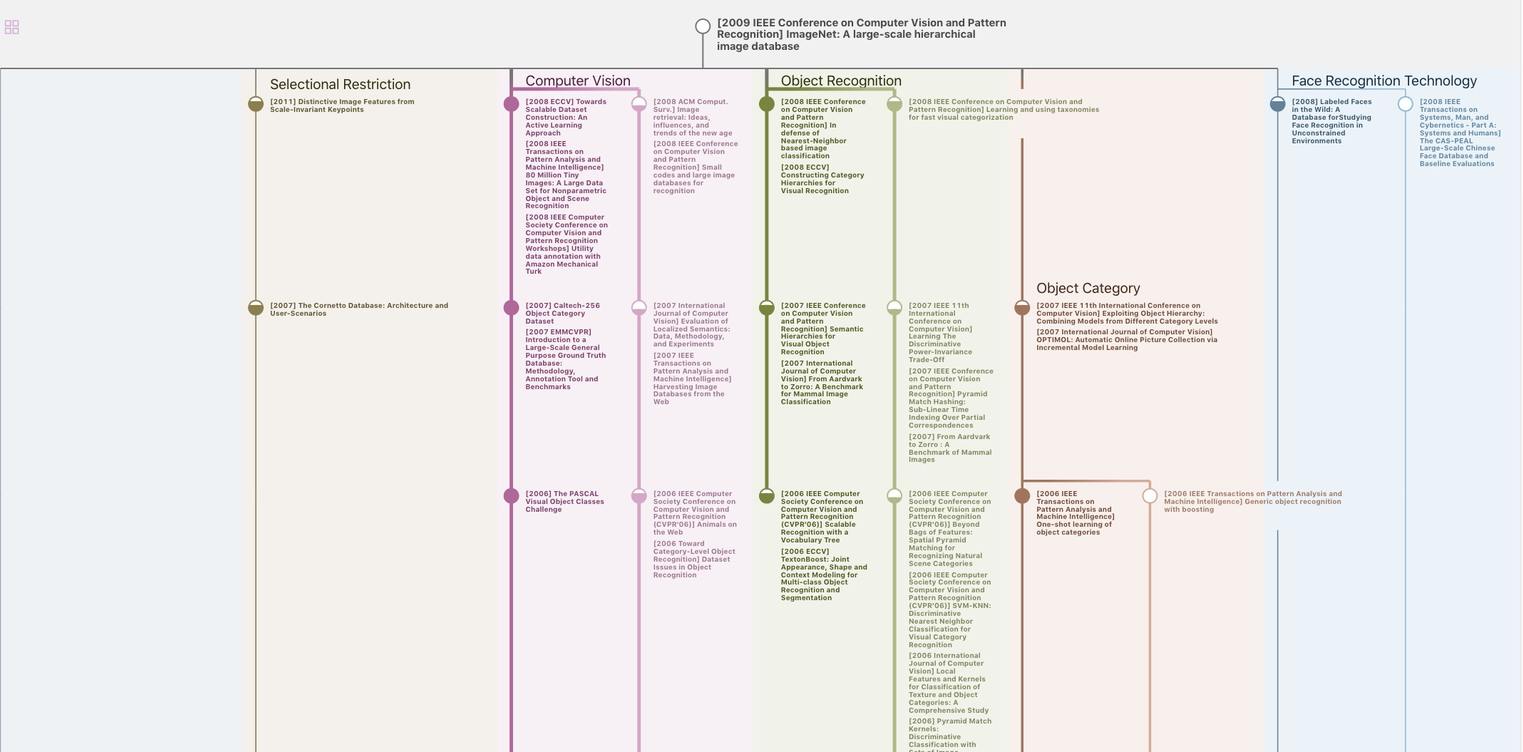
Generate MRT to find the research sequence of this paper
Chat Paper
Summary is being generated by the instructions you defined