Deep-seeded Clustering for Unsupervised Valence-Arousal Emotion Recognition from Physiological Signals
CoRR(2023)
关键词
Emotion Recognition,Affective Computing,Physiological Signals,Deep Learning for EEG,Neuroimaging Data Analysis
AI 理解论文
溯源树
样例
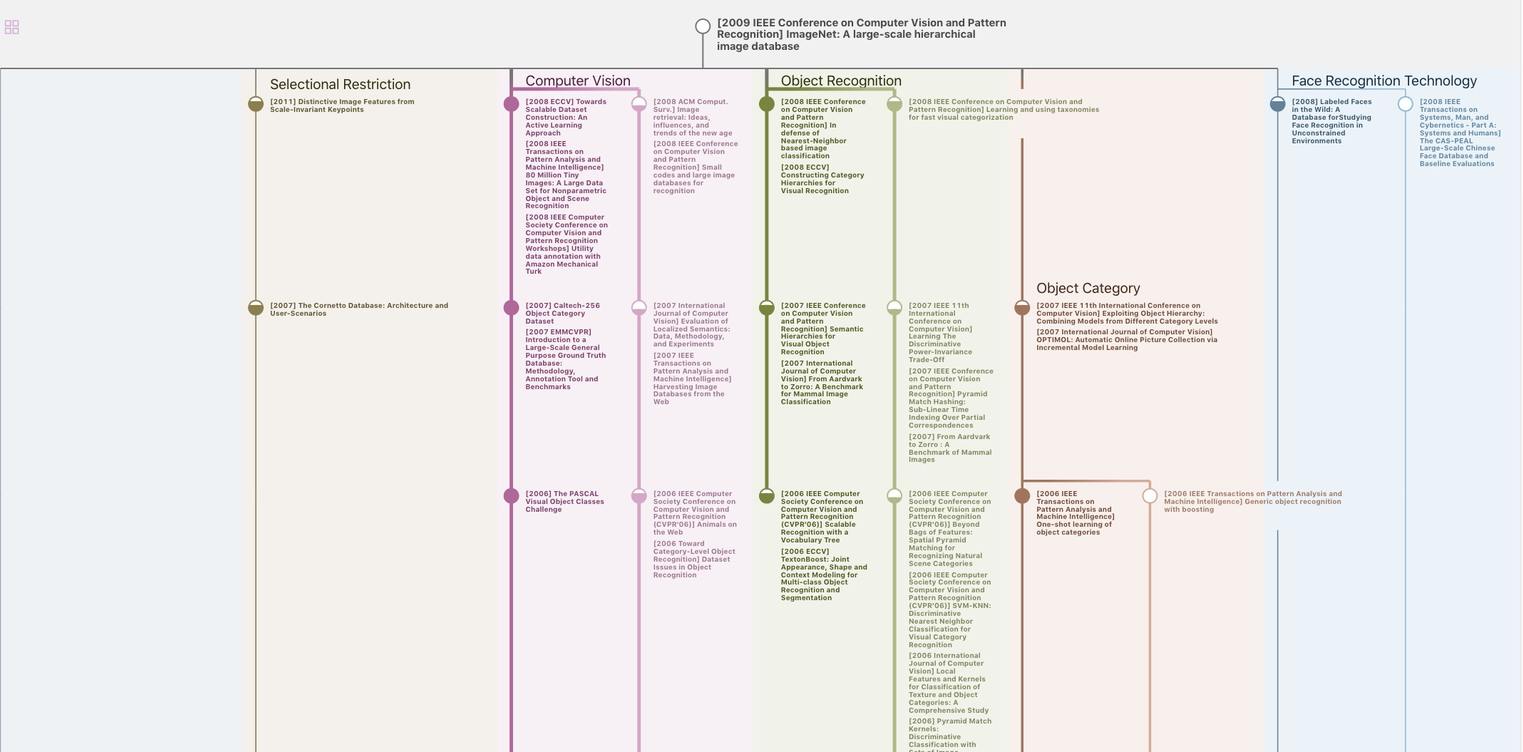
生成溯源树,研究论文发展脉络
Chat Paper
正在生成论文摘要