An Unsupervised Domain Adaptation Method for Intelligent Bearing Fault Diagnosis Based on Signal Reconstruction by Cycle-Consistent Adversarial Learning
IEEE Sensors Journal(2023)
Key words
Bearing,cycle-consistent adversarial (CCA) learning,fault diagnosis,transfer learning,unsupervised domain adaptation
AI Read Science
Must-Reading Tree
Example
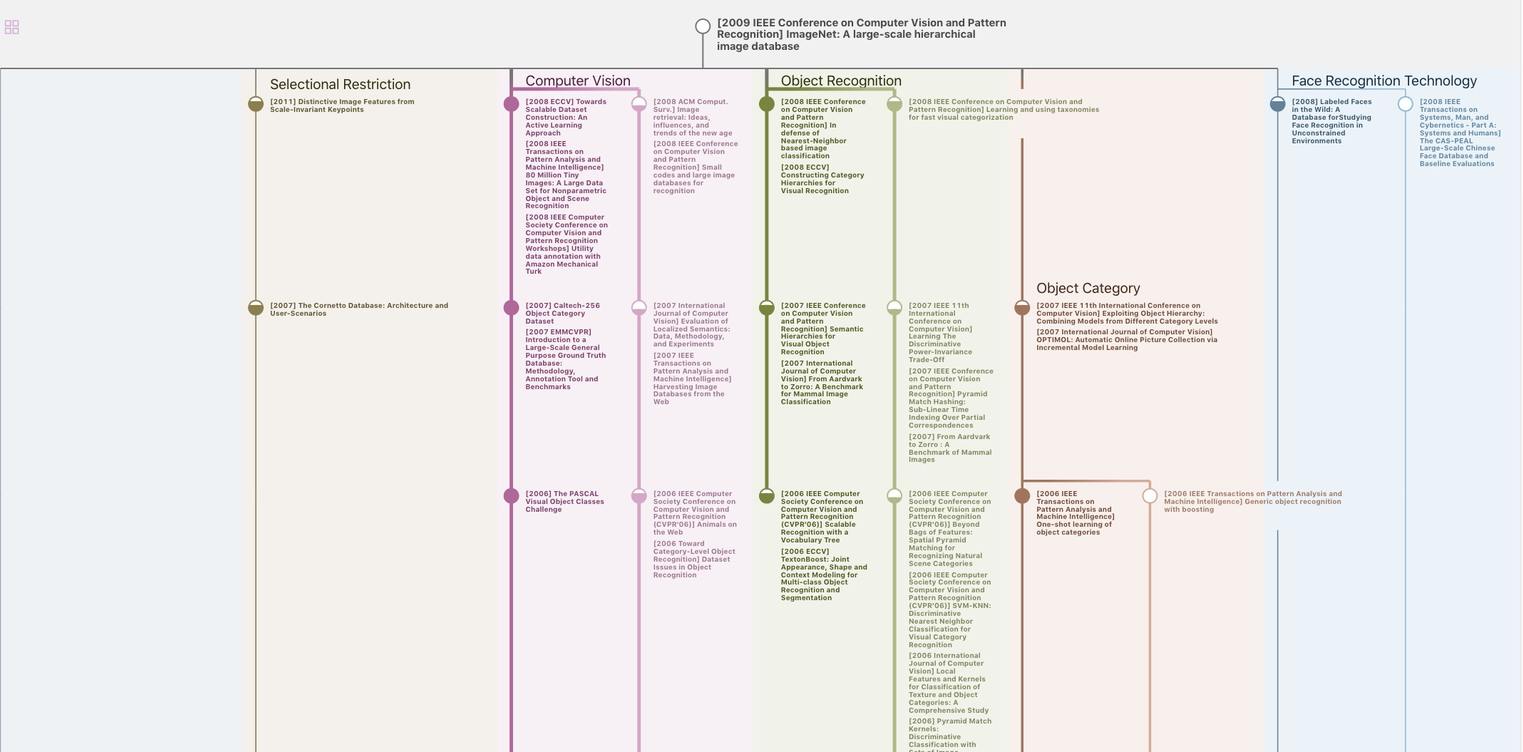
Generate MRT to find the research sequence of this paper
Chat Paper
Summary is being generated by the instructions you defined