Tool Wear Prediction Method Based on Bidirectional Long Short-Term Memory Neural Network of Single Crystal Silicon Micro-Grinding
INTERNATIONAL JOURNAL OF ADVANCED MANUFACTURING TECHNOLOGY(2024)
Key words
Micro-grinding,Tool wear prediction,Neural network,BiLSTM,Small sample,Multi-sourced heterogeneous data
AI Read Science
Must-Reading Tree
Example
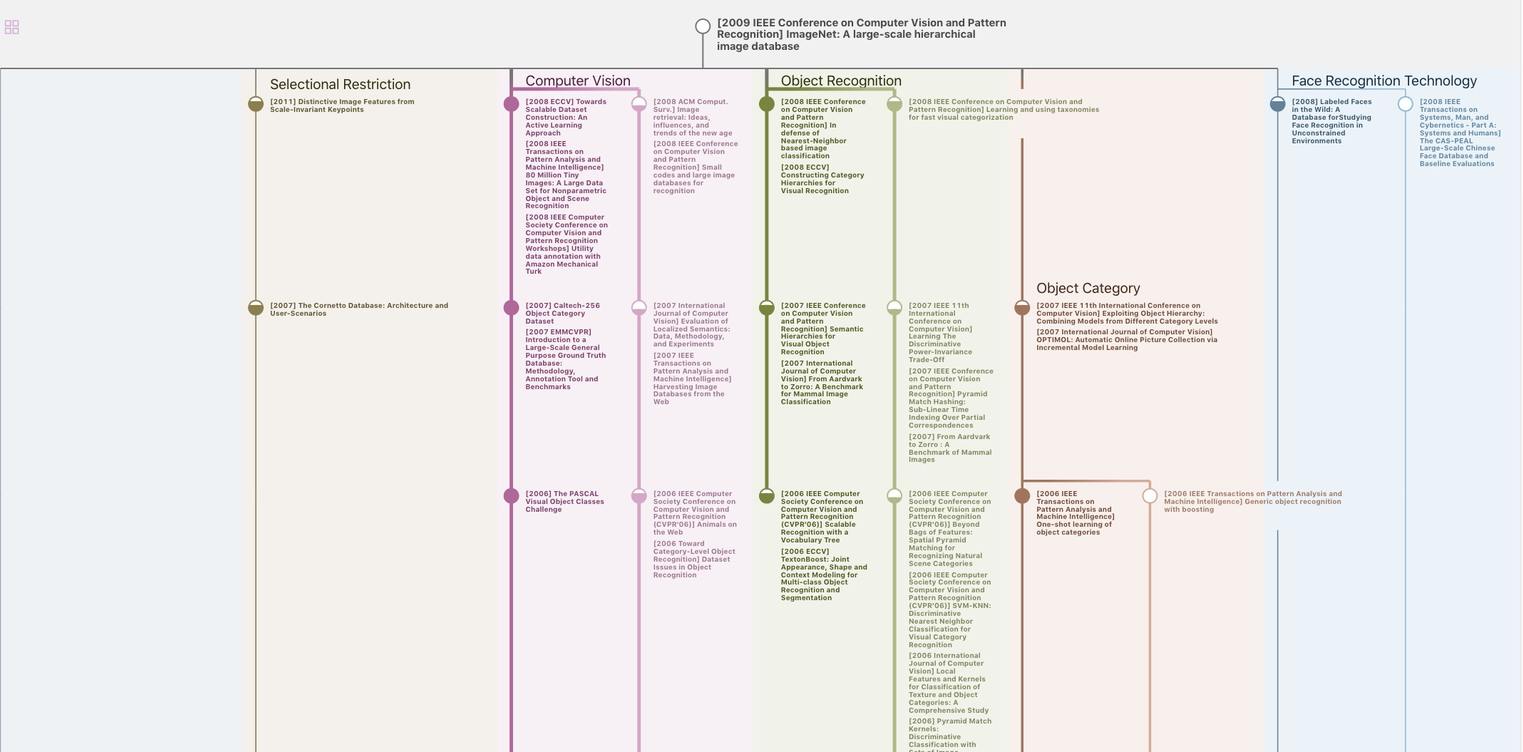
Generate MRT to find the research sequence of this paper
Chat Paper
Summary is being generated by the instructions you defined