Fine Segmentation and Difference-Aware Shape Adjustment for Category-Level 6dof Object Pose Estimation
APPLIED INTELLIGENCE(2023)
Key words
6DoF pose estimation,Shape adjustment,Object segmentation,RGB-D images
AI Read Science
Must-Reading Tree
Example
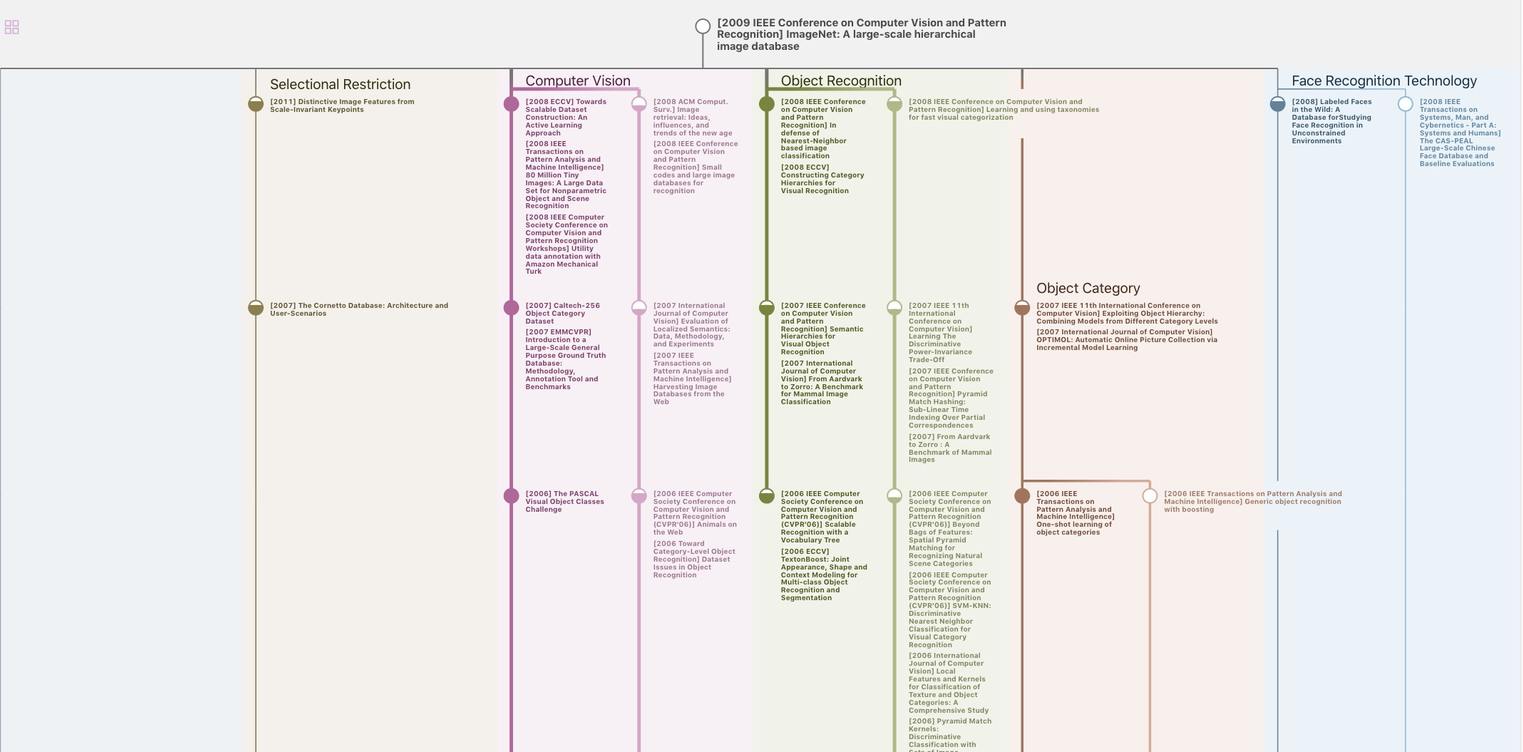
Generate MRT to find the research sequence of this paper
Chat Paper
Summary is being generated by the instructions you defined