Federated Active Semi-Supervised Learning With Communication Efficiency
IEEE TRANSACTIONS ON SYSTEMS MAN CYBERNETICS-SYSTEMS(2023)
摘要
Federated learning (FL) unites multiple participants to collaboratively learn a global consensus model on the centralized server by aggregating their individual models trained locally on clients. To meet the goal of obtaining an optimal model, sufficient labeled data and myriad communications are required during training. However, the major problems are the limited budget for manually annotating unlabeled instances and the restricted bandwidth of server and clients. This article presents a communication-efficient federated active semi-supervised learning (CEFedASSL) framework that unites active learning (AL) clients and a semi-supervised learning (SSL) client to train models on unlabeled data while achieving communication efficiency. In each AL client, different query strategies are, respectively, applied for the local model to obtain a more robust model and query only the optimal samples which significantly reduces the cost of annotation. Subsequently, these optimal samples are encrypted as input to fine-tune the pretrained model of the SSL client by performing self-training, thereby enhancing the model performance while preserving the privacy of data. Furthermore, we propose an efficient selective aggregation strategy to reduce the communication cost between clients and the server. Empirical experiments on four different learning tasks demonstrate that the proposed CEFedASSL distinctively outperforms the common FL algorithms in terms of both model performance and communication costs.
更多查看译文
关键词
learning,communication,semi-supervised
AI 理解论文
溯源树
样例
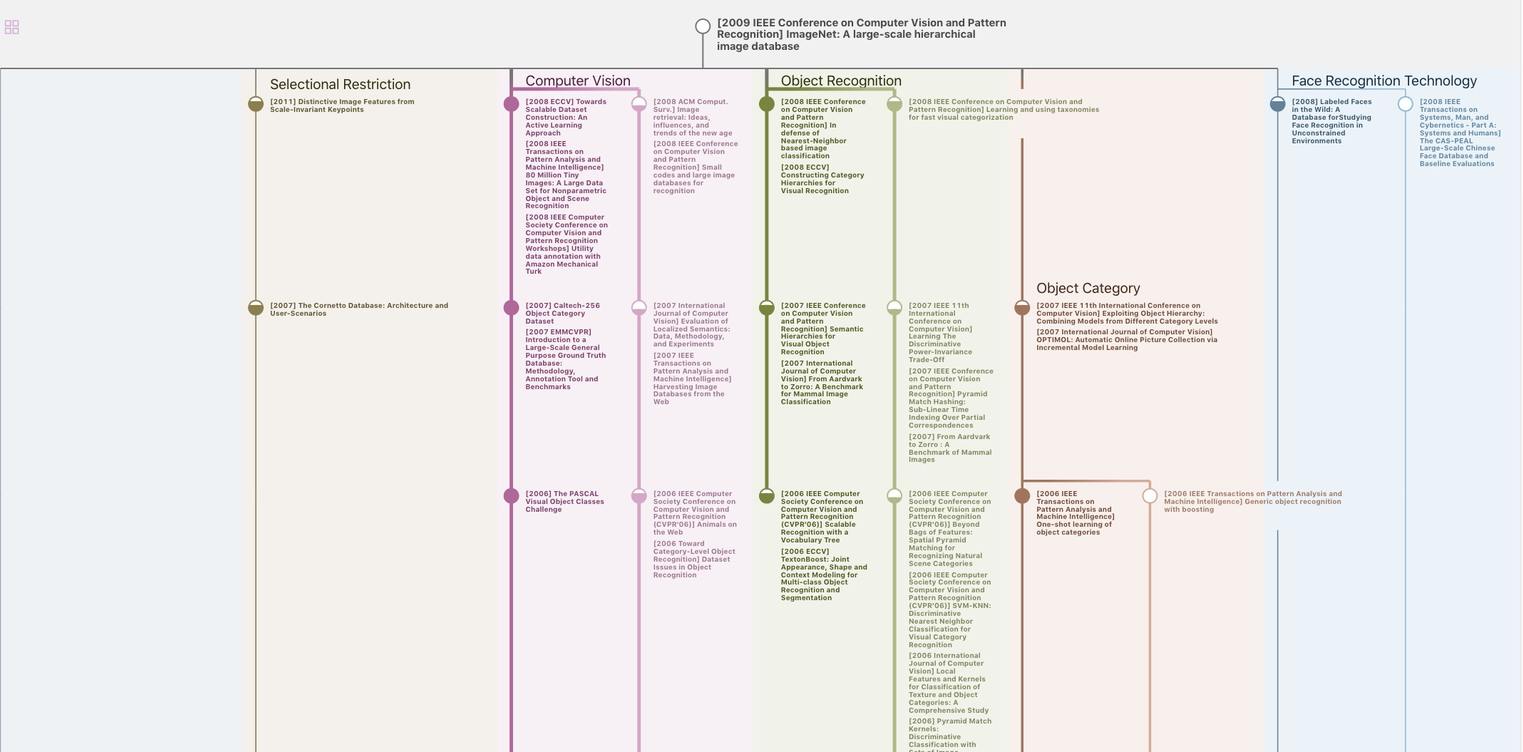
生成溯源树,研究论文发展脉络
Chat Paper
正在生成论文摘要