Anomalous Situations Recognition in Surveillance Images Using Deep Learning
CMC-COMPUTERS MATERIALS & CONTINUA(2023)
关键词
Anomaly detection,anomalous events,anomalous behavior,anomalous objects,violence detection,deep learning
AI 理解论文
溯源树
样例
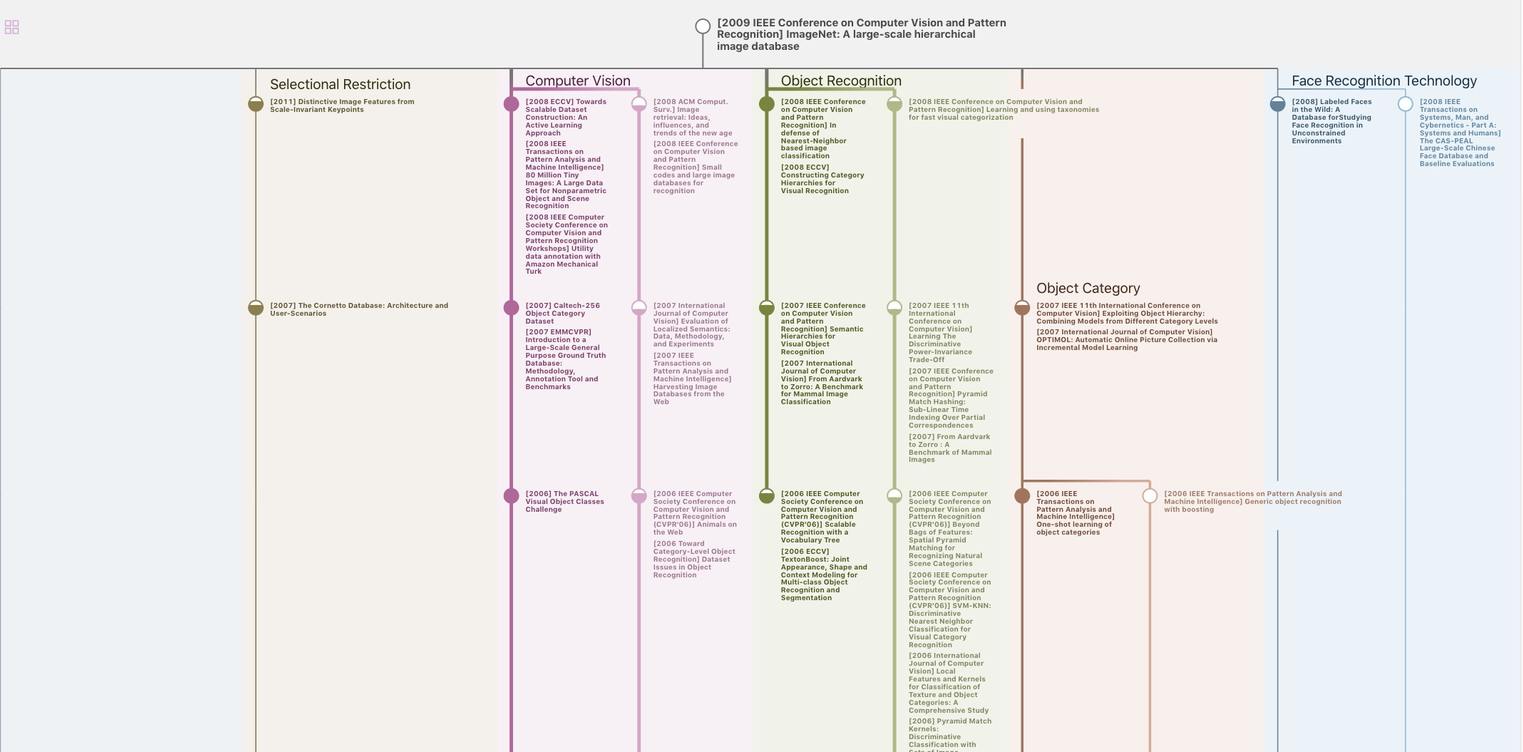
生成溯源树,研究论文发展脉络
Chat Paper
正在生成论文摘要