A Multi-Point Synergistic Gradient Evolution Method for Topology Optimization Leveraging Neural Network with Applications in Converged and Diverse Designs
COMPUTATIONAL MECHANICS(2024)
关键词
Topology optimization,Population-based algorithm,Neural network,Diverse designs,Loss function
AI 理解论文
溯源树
样例
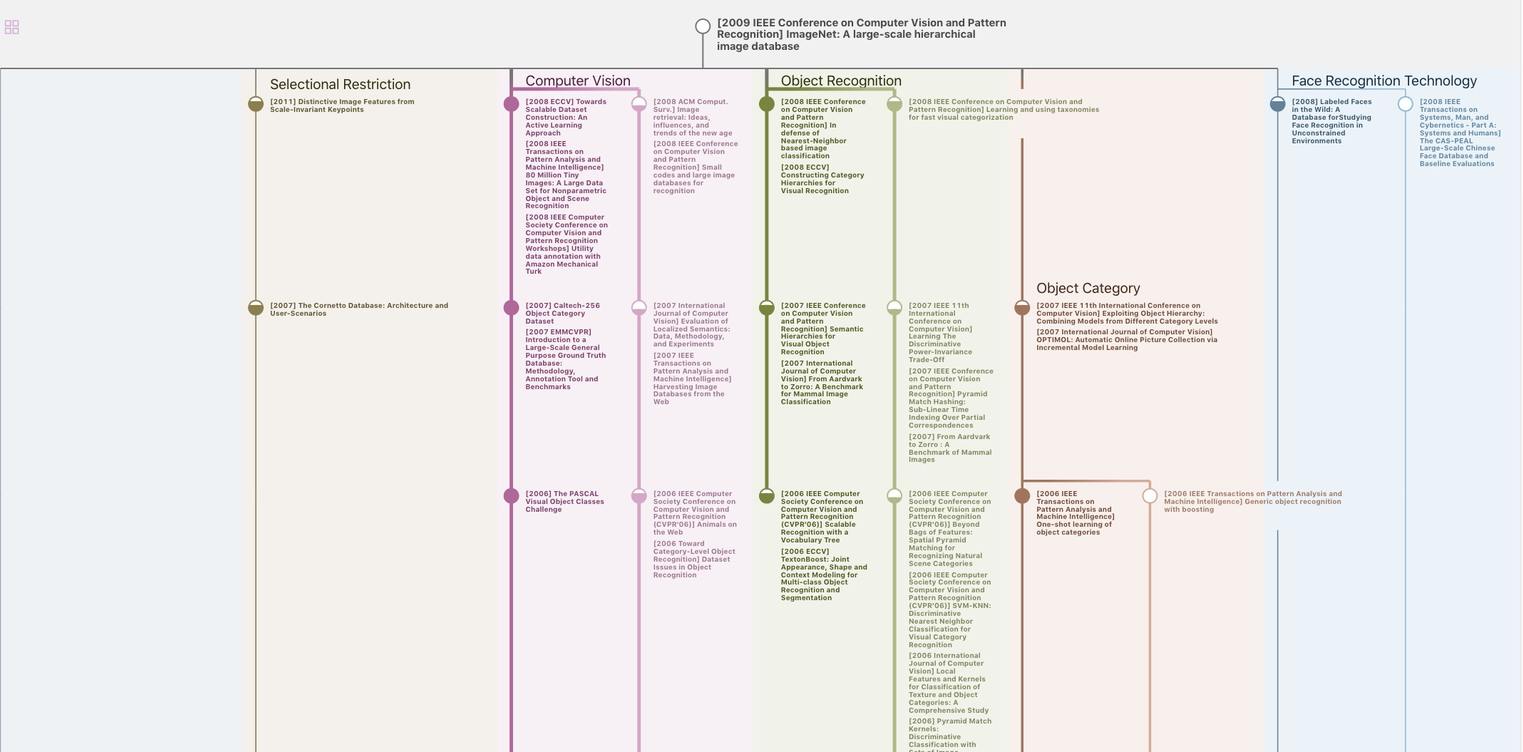
生成溯源树,研究论文发展脉络
Chat Paper
正在生成论文摘要