Top-r keyword-based community search in attributed graphs.
ICDE(2023)
摘要
Community search on attributed graphs has been widely studied recently. Most earlier works aim to retrieve communities relevant to the query nodes Q
U
and query keywords Q
W
, and some recent works begin to focus on keyword-based attributed community search (KACS) with only query keywords Q
W
, aiming to return a structural cohesive community with the highest score relevant to Q
W
. However, these scores only consider the semantic similarity between user attributes and Q
W
and neglect the semantic similarity between users in the community. Thus, we propose a new community model which considers both semantic similarities and uses triangle-connected k-truss to ensure structural cohesiveness, and study the top-r keyword-based attributed community search (rKACS) problem for a given Q
W
to provide more candidates for users to choose the preferred communities. To find the top-r communities, we first propose the Basic algorithm, which gradually finds the communities with large scores through maximal clique enumerations. Then, we further propose an improved algorithm Incremental based on two novel optimization techniques, which can significantly reduce the search space and find the maximal cliques incrementally. Extensive experimental studies on four real-world datasets validated the effectiveness and efficiency of our methods.
更多查看译文
关键词
community search,keyword search,attributed graph
AI 理解论文
溯源树
样例
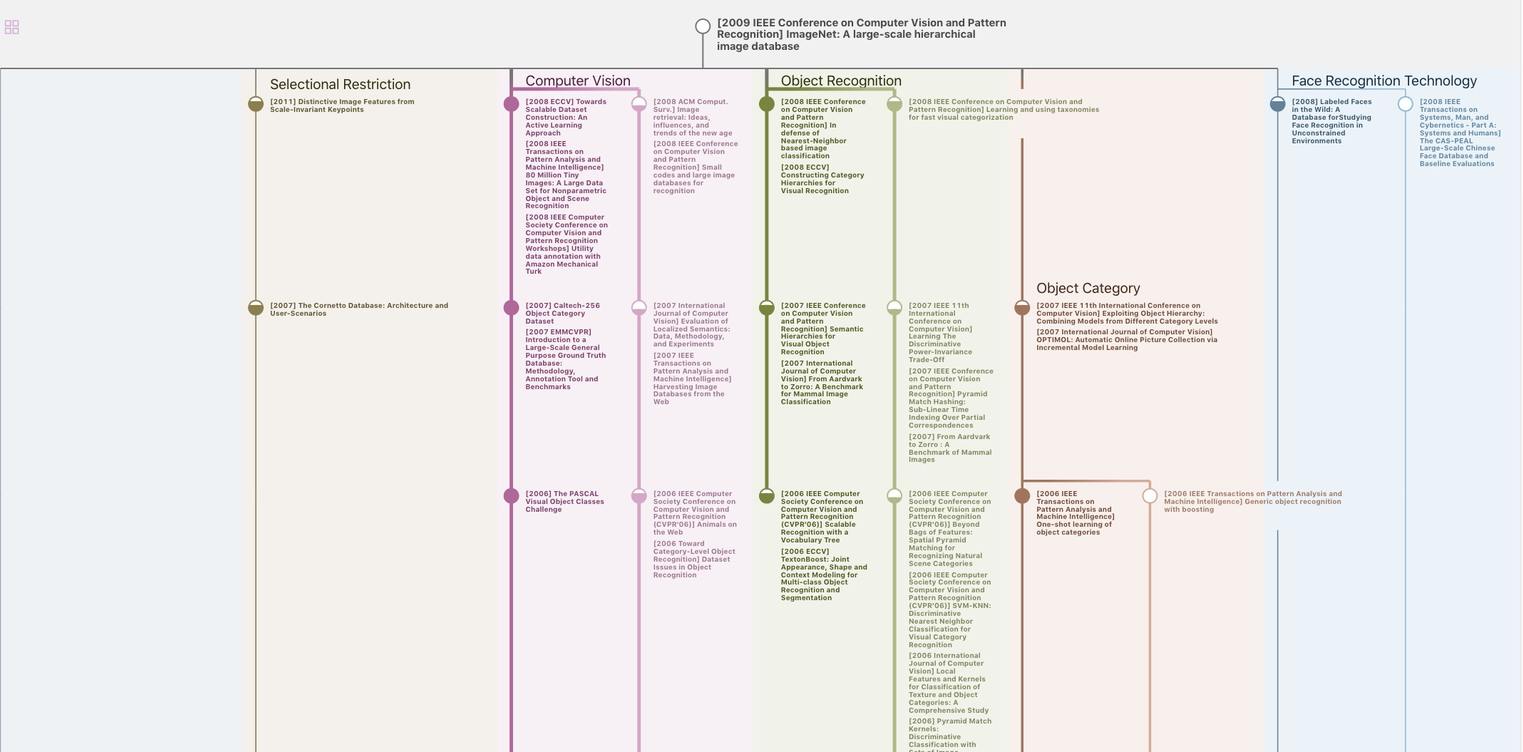
生成溯源树,研究论文发展脉络
Chat Paper
正在生成论文摘要