Data Collection Framework for End-to-End Radio and Transport Network Quality Monitoring.
2023 15TH INTERNATIONAL CONFERENCE ON QUALITY OF MULTIMEDIA EXPERIENCE, QOMEX(2023)
关键词
QoE/QoS,automated network measurement,radio characteristics,data collection framework,synthetic network degradation,radio quality
AI 理解论文
溯源树
样例
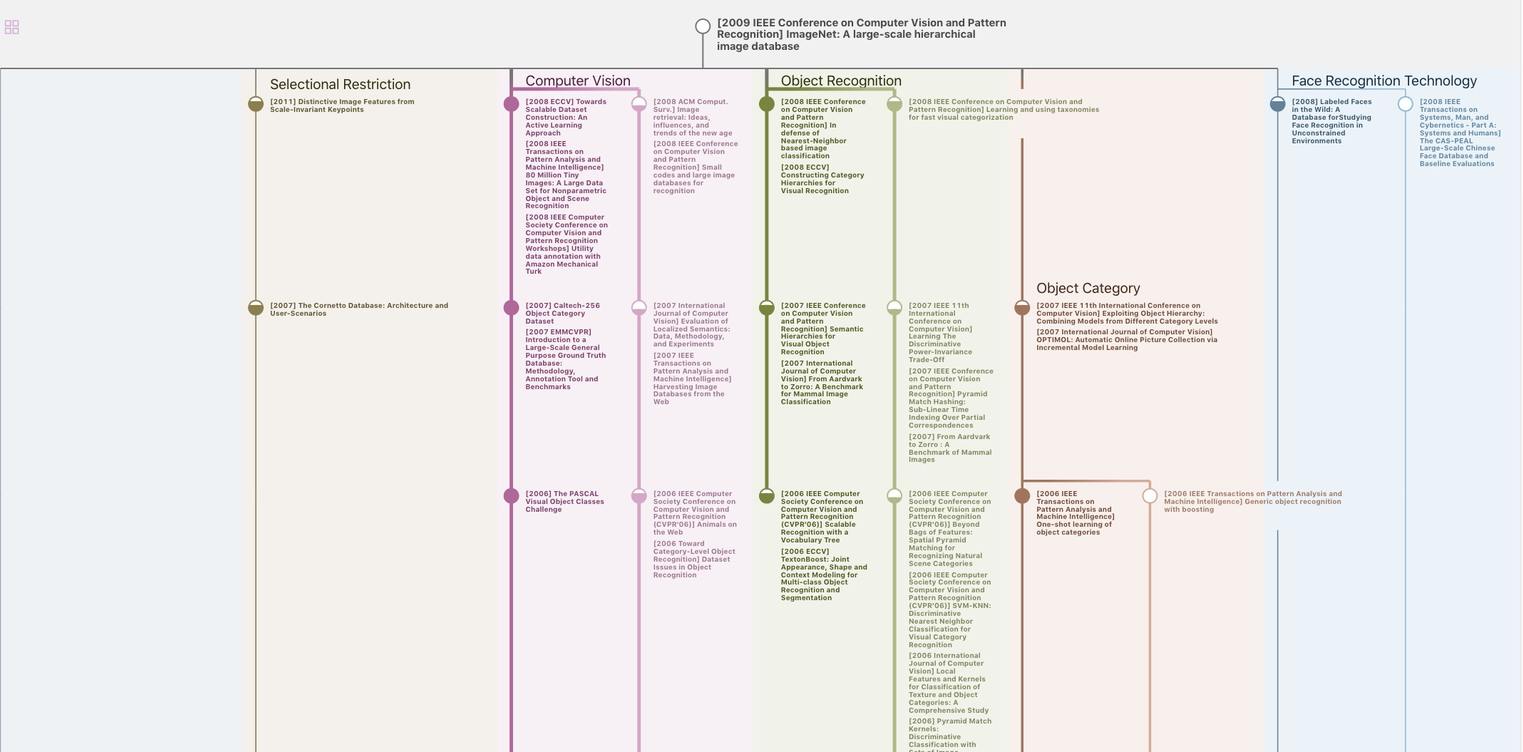
生成溯源树,研究论文发展脉络
Chat Paper
正在生成论文摘要