Timeseries Anomaly Detection Using SAX and Matrix Profiles Based Longest Common Subsequence.
ICCS (2)(2023)
摘要
Similarity search is one of the most popular techniques for time series anomaly detection. This study proposes SAX-MP, a novel similarity search approach that combines Symbolic Aggregate ApproXimation (SAX) and matrix profile (MP). The proposed SAX-MP method consists of two phases. The SAX method is used in the first phase to extract all of the subsequences of a time series, convert them to symbolic strings, and store these strings in an array. In the second phase, the proposed method calculates the MP based on the symbolic strings that are represented for all subsequences extracted in the first phase. Since a subsequence is represented by a symbolic string, the MP is calculated using a distance-based longest common subsequence rather than the z-normalized Euclidean distance. Top- k discords are detected based on the similarity MP. The proposed SAX-MP is implemented on several time series datasets. Experimental results reveal that the SAX-MP method is particularly effective at detecting anomalies when compared to HOT SAX and MP-based methods.
更多查看译文
关键词
timeseries anomaly detection,sax
AI 理解论文
溯源树
样例
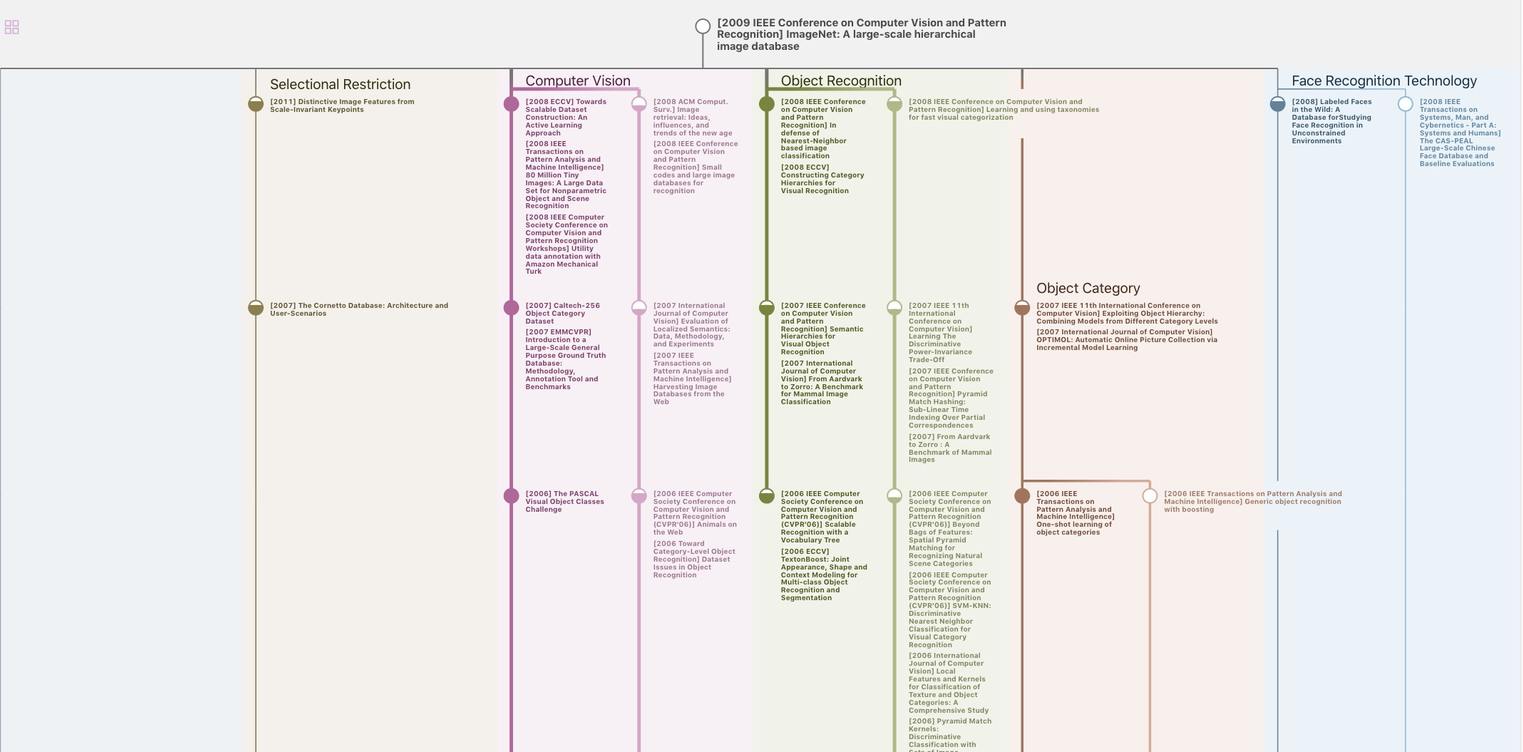
生成溯源树,研究论文发展脉络
Chat Paper
正在生成论文摘要