Detection of Cancer Recurrence Using Systemic Inflammatory Markers and Machine Learning after Concurrent Chemoradiotherapy for Head and Neck Cancers
CANCERS(2023)
摘要
Simple Summary The neutrophil-to-lymphocyte ratio (NLR) and the platelet-to-lymphocyte ratio (PLR) are known as prognosticators in head and neck cancers, but the clinical significance of those values after treatment remains unclear. In this study, we aimed to investigate the relationship between temporal changes in the NLR and PLR values after treatment and cancer recurrence in head and neck cancer patients. We proposed developing a DNN model that utilizes these changes to predict recurrence after CCRT, comparing its performance with traditional machine learning (ML) models. The results of this study showed that the DNN model outperformed the other models, and feature selection using the recursive feature elimination algorithm further improved the performance, with ROC-AUC and PR-AUC values of 0.883 & PLUSMN; 0.027 and 0.778 & PLUSMN; 0.042, respectively. The results could improve prognostication and risk assessment in head and neck cancers. Additionally, this study may inspire further research and advancements in utilizing advanced ML techniques for personalized cancer management and surveillance. Pretreatment values of the neutrophil-to-lymphocyte ratio (NLR) and the platelet-to-lymphocyte ratio (PLR) are well-established prognosticators in various cancers, including head and neck cancers. However, there are no studies on whether temporal changes in the NLR and PLR values after treatment are related to the development of recurrence. Therefore, in this study, we aimed to develop a deep neural network (DNN) model to discern cancer recurrence from temporal NLR and PLR values during follow-up after concurrent chemoradiotherapy (CCRT) and to evaluate the model's performance compared with conventional machine learning (ML) models. Along with conventional ML models such as logistic regression (LR), random forest (RF), and gradient boosting (GB), the DNN model to discern recurrences was trained using a dataset of 778 consecutive patients with primary head and neck cancers who received CCRT. There were 16 input features used, including 12 laboratory values related to the NLR and the PLR. Along with the original training dataset (N = 778), data were augmented to split the training dataset (N = 900). The model performance was measured using ROC-AUC and PR-AUC values. External validation was performed using a dataset of 173 patients from an unrelated external institution. The ROC-AUC and PR-AUC values of the DNN model were 0.828 & PLUSMN; 0.032 and 0.663 & PLUSMN; 0.069, respectively, in the original training dataset, which were higher than the ROC-AUC and PR-AUC values of the LR, RF, and GB models in the original training dataset. With the recursive feature elimination (RFE) algorithm, five input features were selected. The ROC-AUC and PR-AUC values of the DNN-RFE model were higher than those of the original DNN model (0.883 & PLUSMN; 0.027 and 0.778 & PLUSMN; 0.042, respectively). The ROC-AUC and PR-AUC values of the DNN-RFE model trained with a split dataset were 0.889 & PLUSMN; 0.032 and 0.771 & PLUSMN; 0.044, respectively. In the external validation, the ROC-AUC values of the DNN-RFE model trained with the original dataset and the same model trained with the split dataset were 0.710 and 0.784, respectively. The DNN model with feature selection using the RFE algorithm showed the best performance among the ML models to discern a recurrence after CCRT in patients with head and neck cancers. Data augmentation by splitting training data was helpful for model performance. The performance of the DNN-RFE model was also validated with an external dataset.
更多查看译文
关键词
neck cancers,cancer recurrence,concurrent chemoradiotherapy,systemic inflammatory markers
AI 理解论文
溯源树
样例
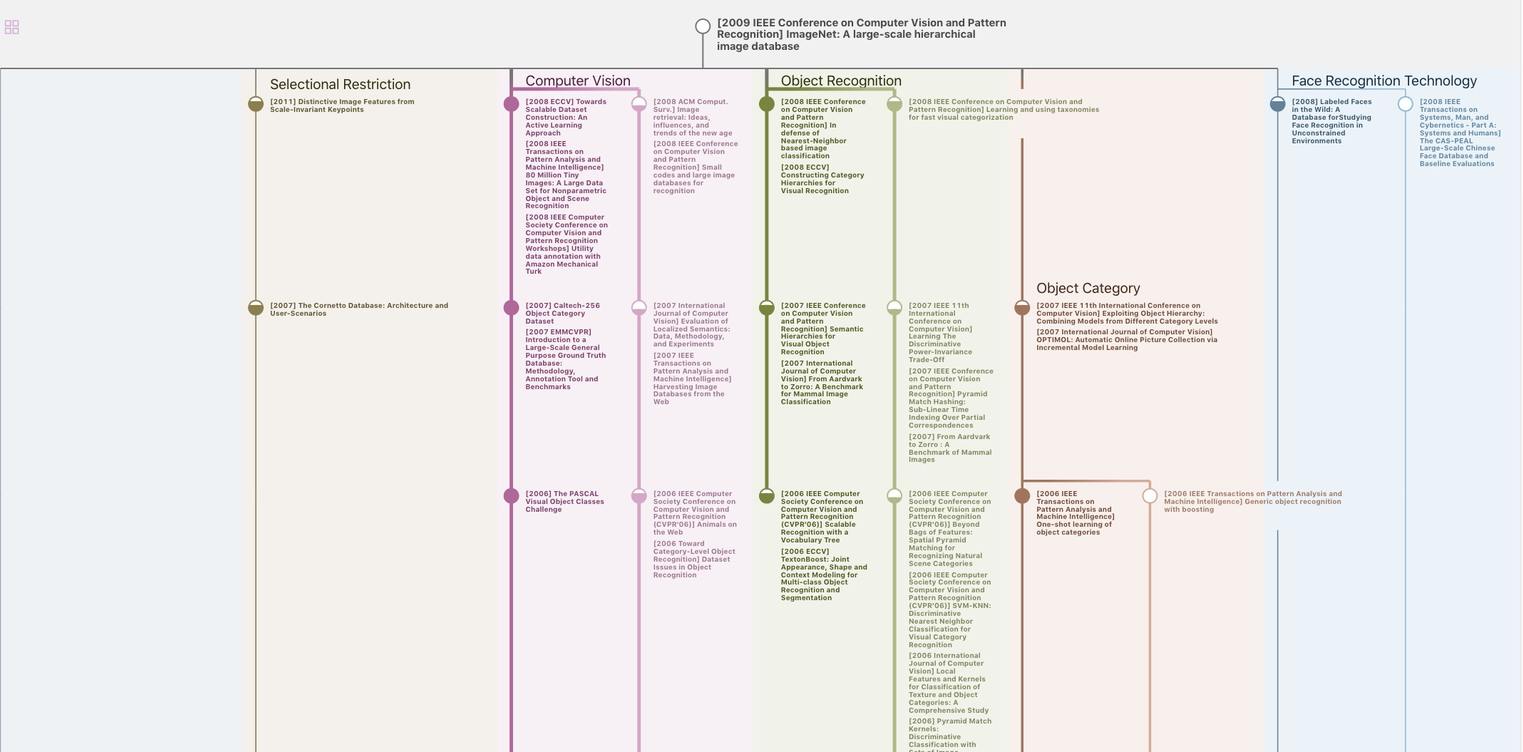
生成溯源树,研究论文发展脉络
Chat Paper
正在生成论文摘要