AI-enabled Lorentz microscopy for quantitative imaging of nanoscale magnetic spin textures
arXiv (Cornell University)(2023)
摘要
The manipulation and control of nanoscale magnetic spin textures is of rising interest as they are potential foundational units in next-generation computing paradigms. Achieving this requires a quantitative understanding of the spin texture behavior under external stimuli using in situ experiments. Lorentz transmission electron microscopy (LTEM) enables real-space imaging of spin textures at the nanoscale, but quantitative characterization of in situ data is extremely challenging. Here, we present an AI-enabled phase-retrieval method based on integrating a generative deep image prior with an image formation forward model for LTEM. Our approach uses a single out-of-focus image for phase retrieval and achieves significantly higher accuracy and robustness to noise compared to existing methods. Furthermore, our method is capable of isolating sample heterogeneities from magnetic contrast, as shown by application to simulated and experimental data. This approach allows quantitative phase reconstruction of in situ data and can also enable near real-time quantitative magnetic imaging.
更多查看译文
关键词
lorentz microscopy,spin,quantitative imaging,nanoscale
AI 理解论文
溯源树
样例
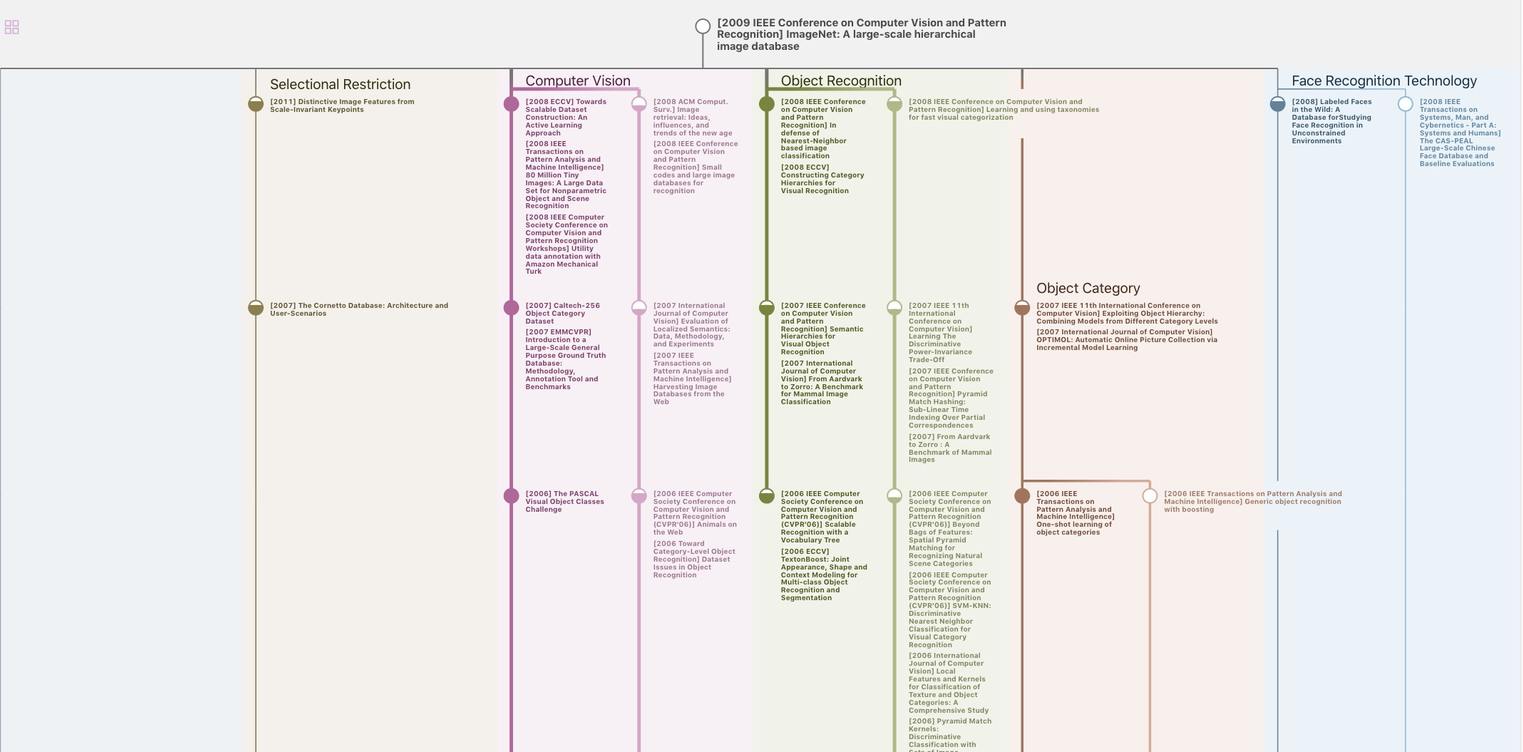
生成溯源树,研究论文发展脉络
Chat Paper
正在生成论文摘要