YOLIC: an Efficient Method for Object Localization and Classification on Edge Devices.
IMAGE AND VISION COMPUTING(2024)
关键词
Object localization and classification,Cell-wise segmentation,Real-time detection,Tiny AI
AI 理解论文
溯源树
样例
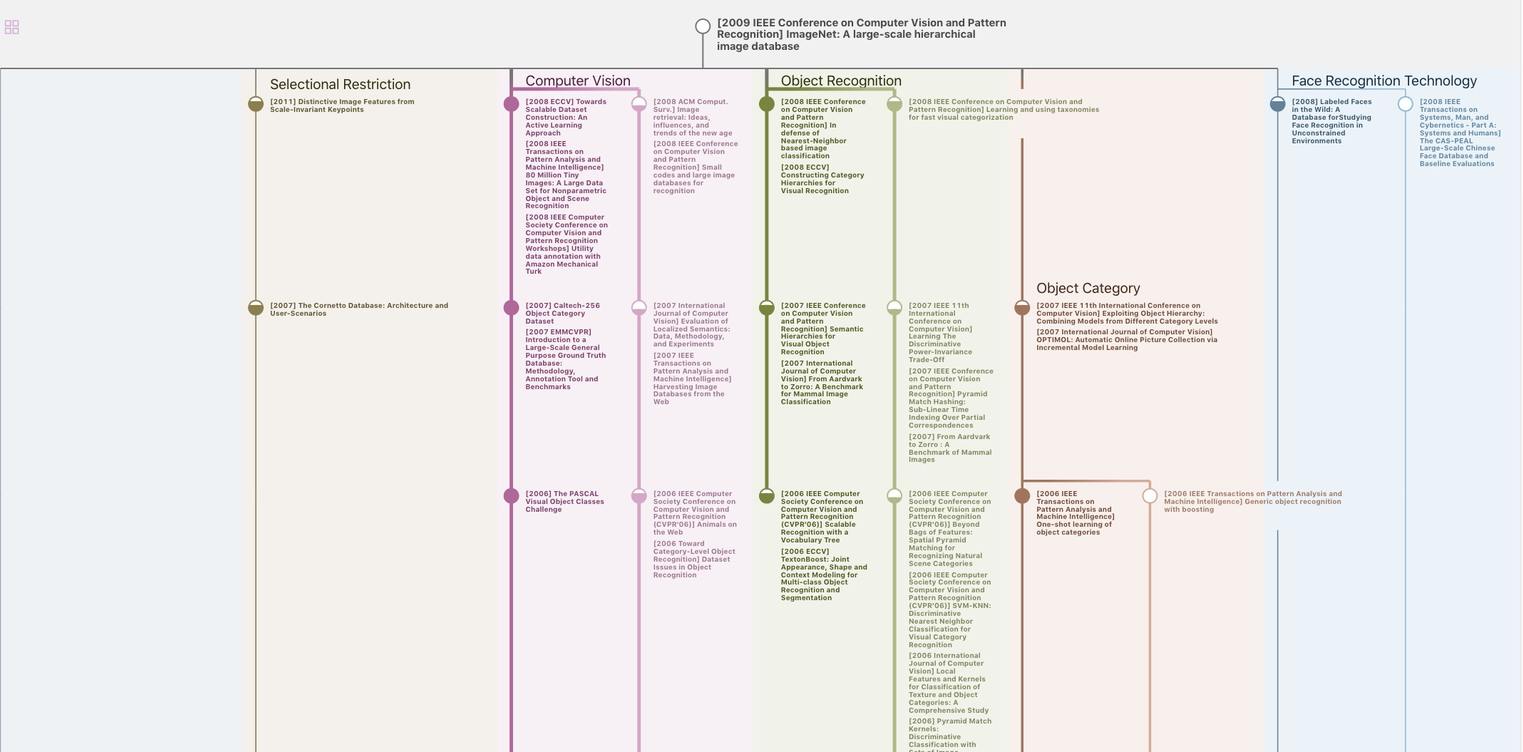
生成溯源树,研究论文发展脉络
Chat Paper
正在生成论文摘要