Mitigating Action Hysteresis in Traffic Signal Control with Traffic Predictive Reinforcement Learning.
KDD 2023(2023)
Key words
Traffic Signal Control,Traffic State Prediction,Reinforcement Learning,Graph Convolutional Networks,Attention Mechanism
AI Read Science
Must-Reading Tree
Example
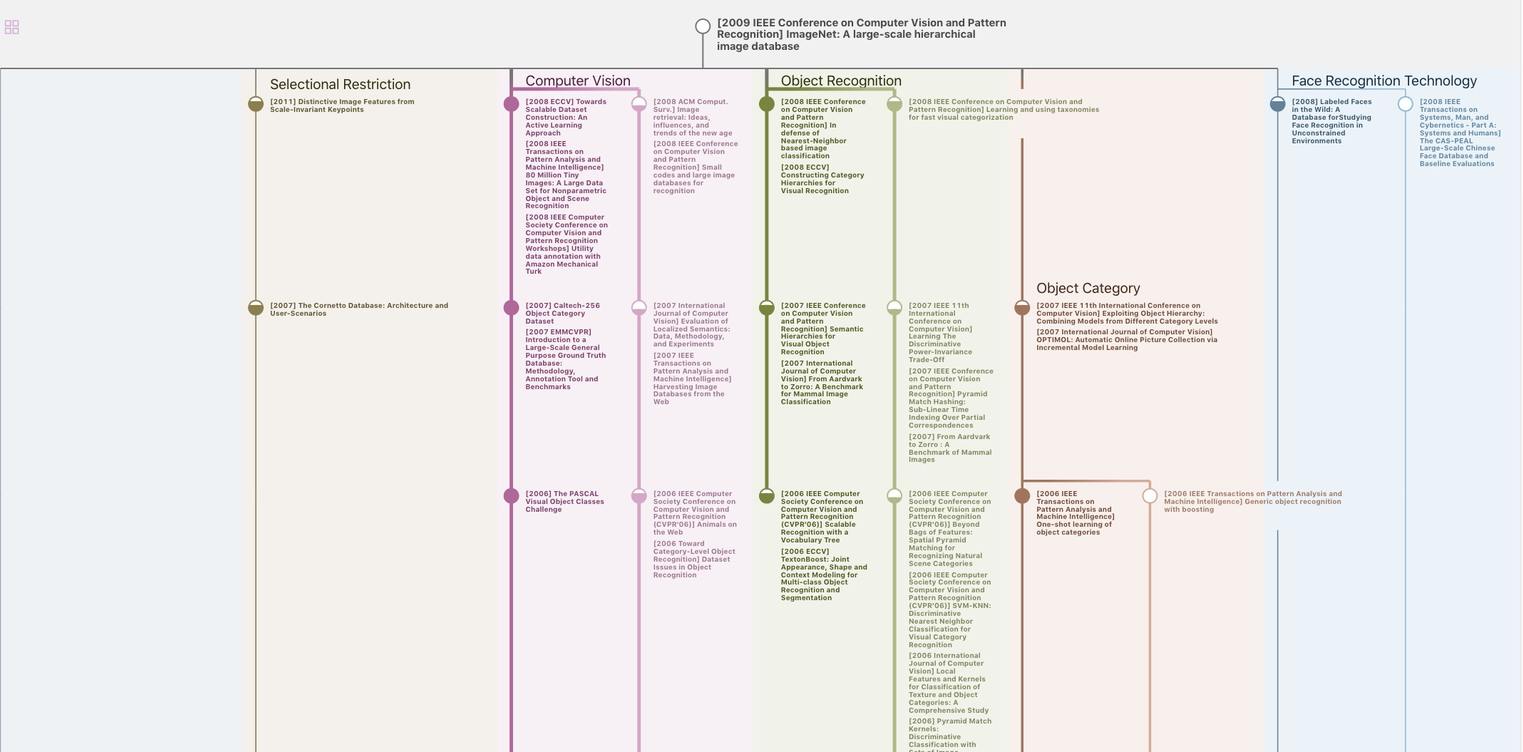
Generate MRT to find the research sequence of this paper
Chat Paper
Summary is being generated by the instructions you defined