Deep Generative Model for Inverse Design of High-Temperature Superconductor Compositions with Predicted T c > 77 K.
ACS applied materials & interfaces(2023)
摘要
Identifying new superconductors with high transition temperatures ( > 77 K) is a major goal in modern condensed matter physics. The inverse design of high superconductors relies heavily on an effective representation of the superconductor hyperspace due to the underlying complexity involving many-body physics, doping chemistry and materials, and defect structures. In this study, we propose a deep generative model that combines two widely used machine learning algorithms, namely, the variational auto-encoder (VAE) and the generative adversarial network (GAN), to systematically generate unknown superconductors under the given high condition. After training, we successfully identified the distribution of the representative hyperspace of superconductors with different , in which many superconductor constituent elements were found adjacent to each other with their neighbors in the periodic table. Equipped with the conditional distribution of , our deep generative model predicted hundreds of superconductors with > 77 K, as predicted by the published prediction models in the literature. For the copper-based superconductors, our results reproduced the variation in Tc as a function of the Cu concentration and predicted an optimal = 129.4 K, when the Cu concentration reached 2.41 in HgBaCaCuOTl. We expect that such an inverse design model and comprehensive list of potential high Tc superconductors would greatly facilitate future research activities in superconductors.
更多查看译文
关键词
high T (c) superconductors, inverse design, deep generative model, variationalautoencoder, generative adversarial network
AI 理解论文
溯源树
样例
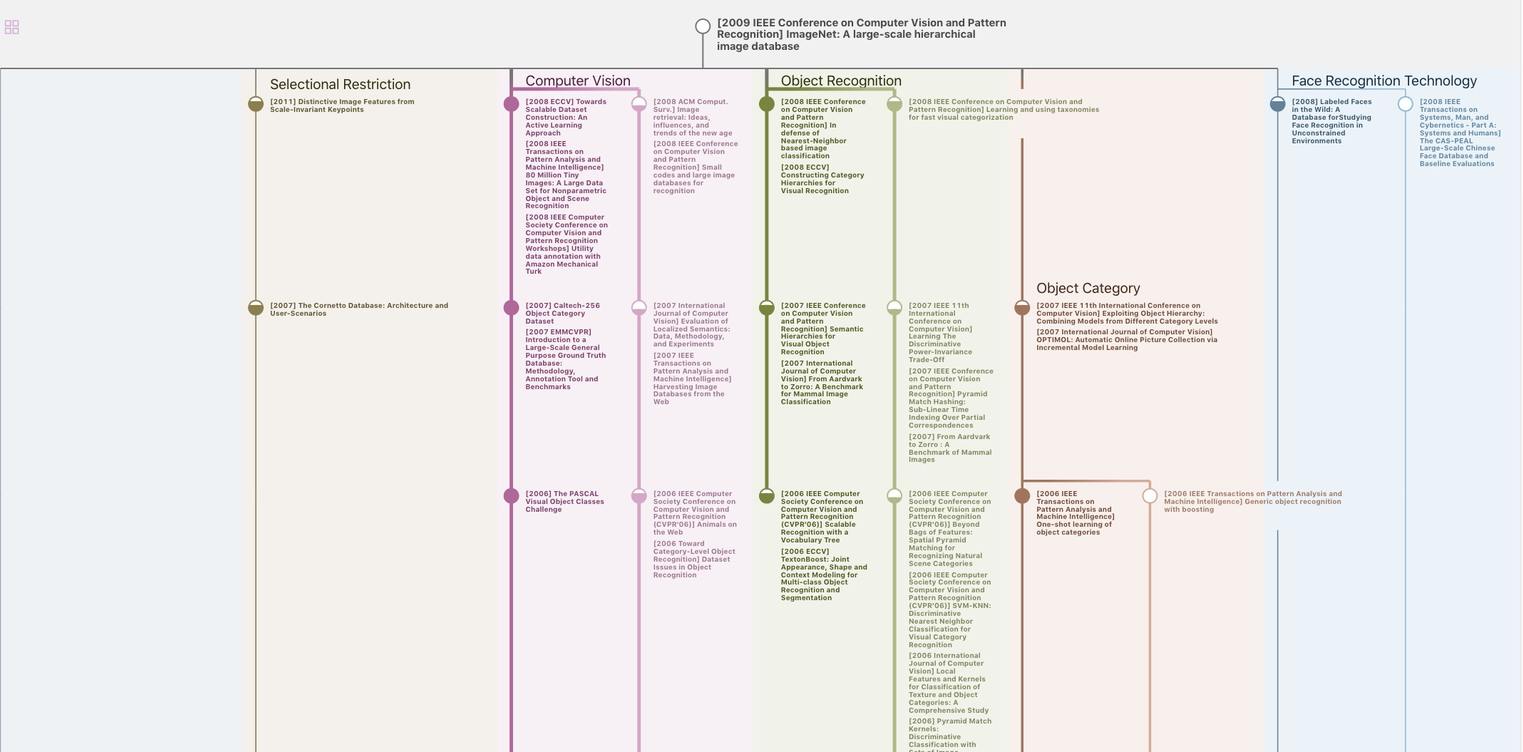
生成溯源树,研究论文发展脉络
Chat Paper
正在生成论文摘要