NN-EVP: A physics informed neural network-based elasto-viscoplastic framework for predictions of grain size-aware flow response under large deformations
CoRR(2023)
摘要
We propose a physics informed, neural network-based elasto-viscoplasticity (NN-EVP) constitutive modeling framework for predicting the flow response in metals as a function of underlying grain size. The developed NN-EVP algorithm is based on input convex neural networks as a means to strictly enforce thermodynamic consistency, while allowing high expressivity towards model discovery from limited data. It utilizes state-of-the-art machine learning tools within PyTorch's high-performance library providing a flexible tool for data-driven, automated constitutive modeling. To test the performance of the framework, we generate synthetic stress-strain curves using a power law-based model with phenomenological hardening at small strains and test the trained model for strain amplitudes beyond the training data. Next, experimentally measured flow responses obtained from uniaxial deformations are used to train the framework under large plastic deformations. Ultimately, the Hall-Petch relationship corresponding to grain size strengthening is discovered by training flow response as a function of grain size, also leading to efficient extrapolation. The present work demonstrates a successful integration of neural networks into elasto-viscoplastic constitutive laws, providing a robust automated framework for constitutive model discovery that can efficiently generalize, while also providing insights into predictions of flow response and grain size-property relationships in metals and metallic alloys under large plastic deformations.
更多查看译文
关键词
deformations,flow,grain,nn-evp,network-based,elasto-viscoplastic,size-aware
AI 理解论文
溯源树
样例
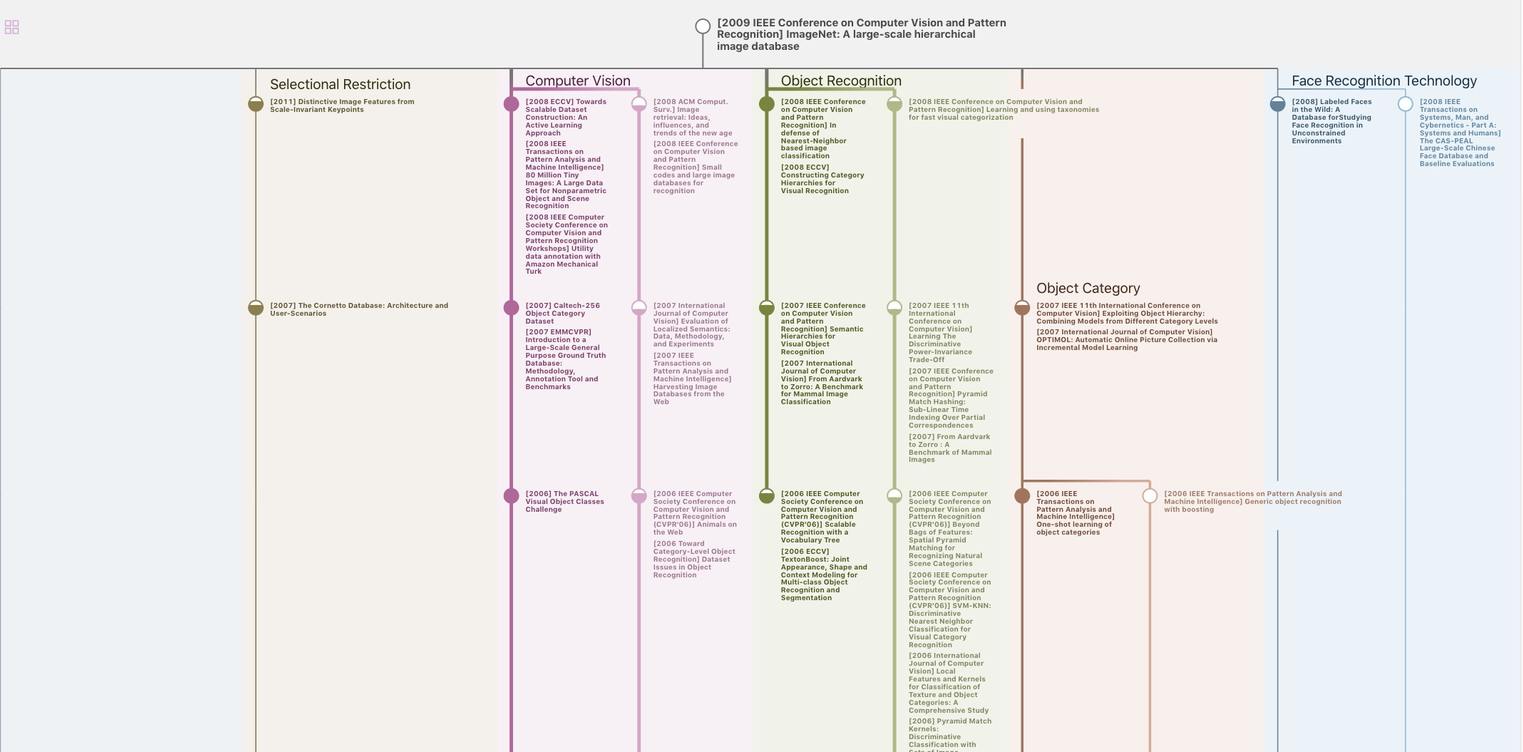
生成溯源树,研究论文发展脉络
Chat Paper
正在生成论文摘要