Predicting Soil Carbon by Efficiently Using Variation in a Mid-Ir Soil Spectral Library
openalex(2021)
Key words
Soil Carbon Sequestration,Digital Soil Mapping,Terrain Analysis,Global Soil Information,Soil Properties
AI Read Science
Must-Reading Tree
Example
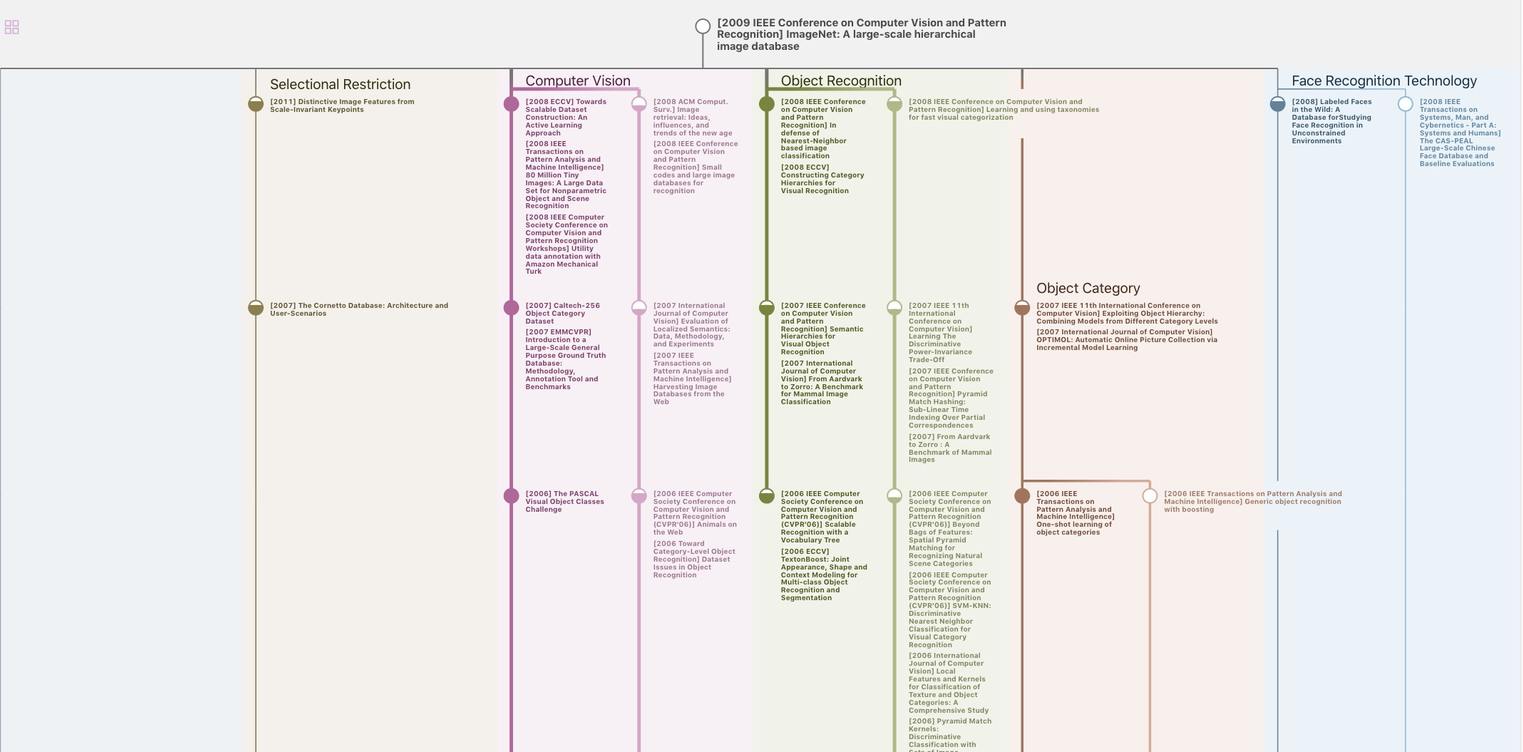
Generate MRT to find the research sequence of this paper
Chat Paper
Summary is being generated by the instructions you defined