Comparative analysis of machine learning vs. traditional modeling approaches for predicting in-hospital mortality after cardiac surgery: temporal and spatial external validation based on a nationwide cardiac surgery registry
EUROPEAN HEART JOURNAL-QUALITY OF CARE AND CLINICAL OUTCOMES(2023)
摘要
Aims Preoperative risk assessment is crucial for cardiac surgery. Although previous studies suggested machine learning (ML) may improve in-hospital mortality predictions after cardiac surgery compared to traditional modeling approaches, the validity is doubted due to lacking external validation, limited sample sizes, and inadequate modeling considerations. We aimed to assess predictive performance between ML and traditional modelling approaches, while addressing these major limitations. Methods and results Adult cardiac surgery cases (n = 168 565) between 2013 and 2018 in the Chinese Cardiac Surgery Registry were used to develop, validate, and compare various ML vs. logistic regression (LR) models. The dataset was split for temporal (2013-2017 for training, 2018 for testing) and spatial (geographically-stratified random selection of 83 centers for training, 22 for testing) experiments, respectively. Model performances were evaluated in testing sets for discrimination and calibration. The overall in-hospital mortality was 1.9%. In the temporal testing set (n = 32 184), the best-performing ML model demonstrated a similar area under the receiver operating characteristic curve (AUC) of 0.797 (95% CI 0.779-0.815) to the LR model (AUC 0.791 [95% CI 0.775-0.808]; P = 0.12). In the spatial experiment (n = 28 323), the best ML model showed a statistically better but modest performance improvement (AUC 0.732 [95% CI 0.710-0.754]) than LR (AUC 0.713 [95% CI 0.691-0.737]; P = 0.002). Varying feature selection methods had relatively smaller effects on ML models. Most ML and LR models were significantly miscalibrated. Conclusion ML provided only marginal improvements over traditional modelling approaches in predicting cardiac surgery mortality with routine preoperative variables, which calls for more judicious use of ML in practice.
更多查看译文
关键词
nationwide cardiac surgery registry,cardiac surgery,machine learning,mortality,in-hospital
AI 理解论文
溯源树
样例
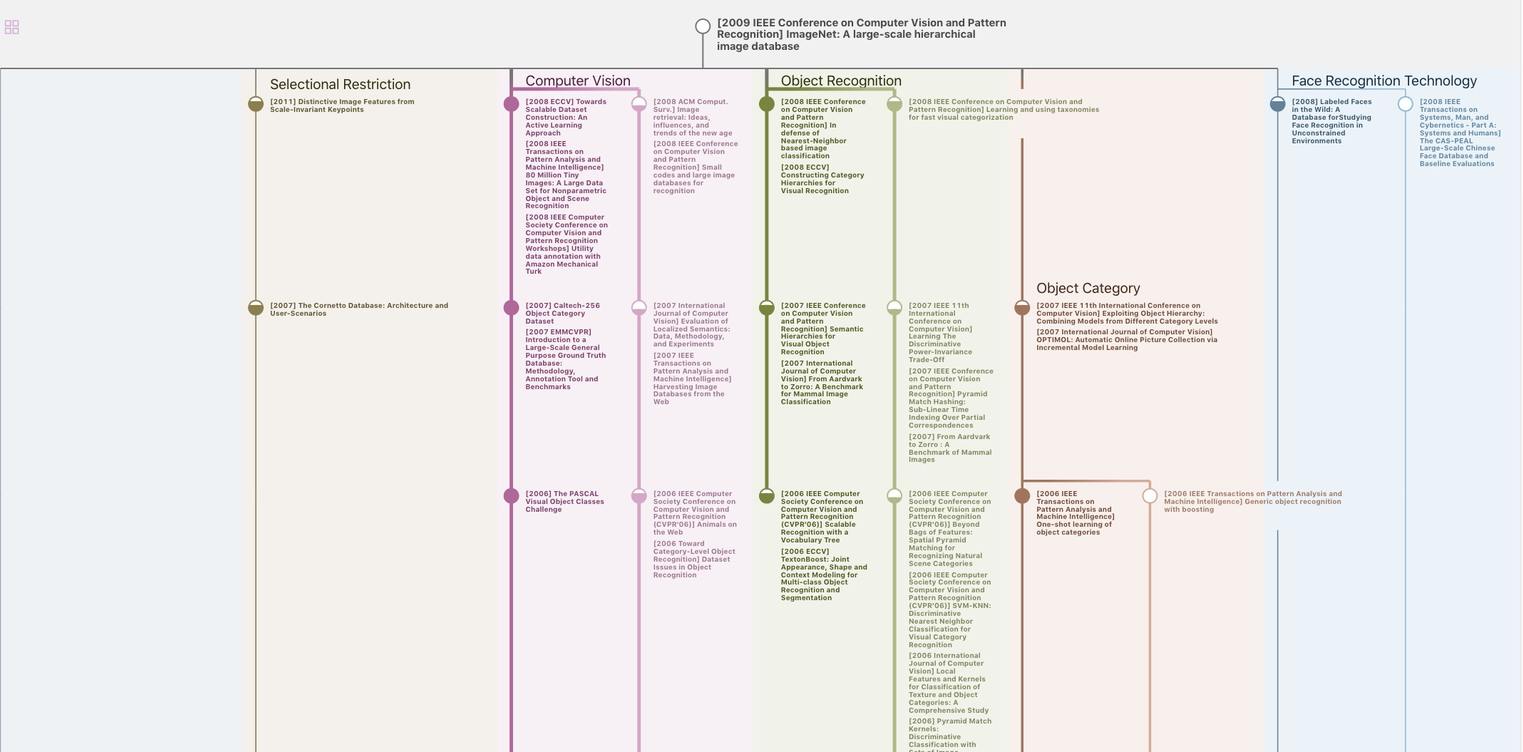
生成溯源树,研究论文发展脉络
Chat Paper
正在生成论文摘要